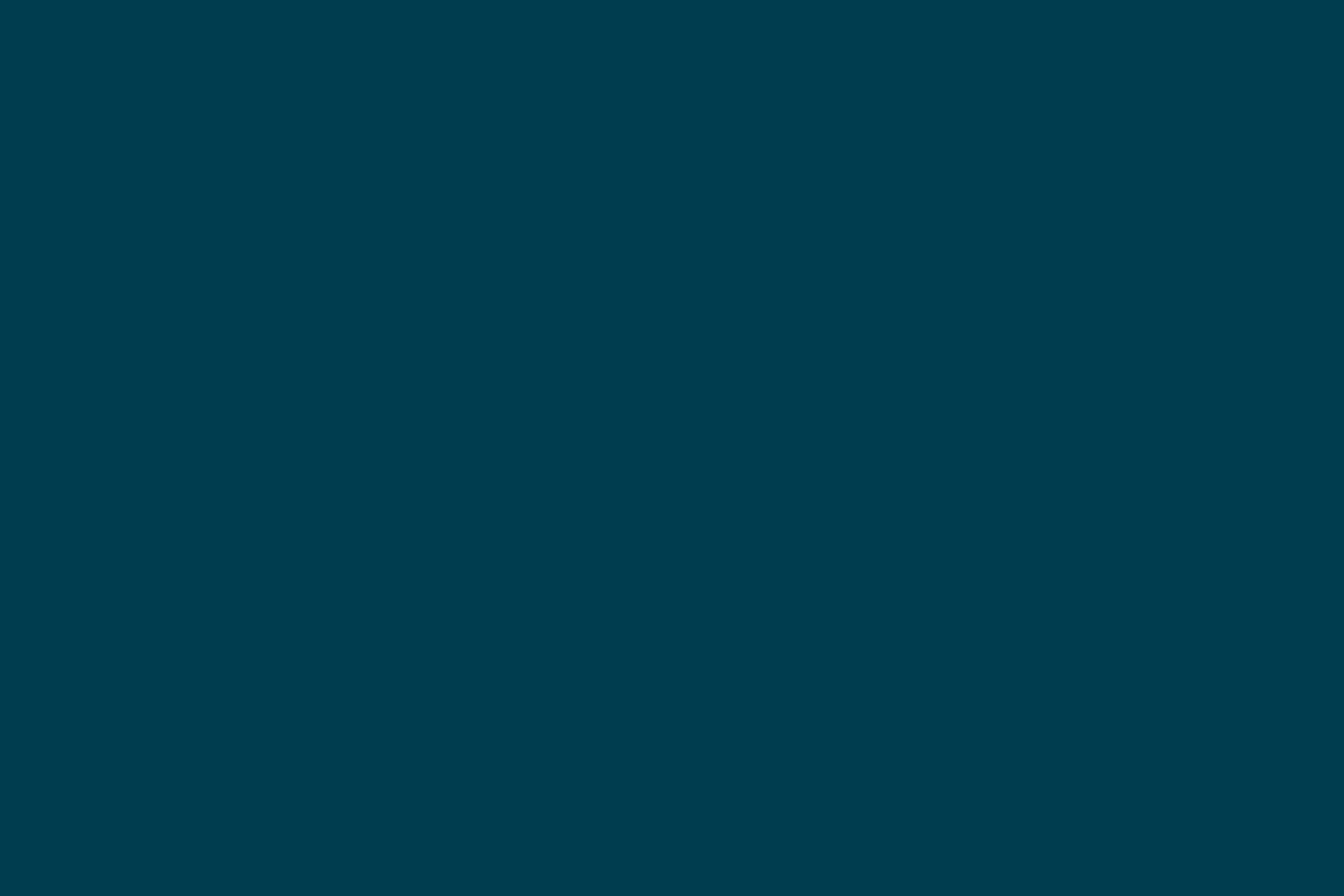
9 Data Trends You Need to Know For 2016
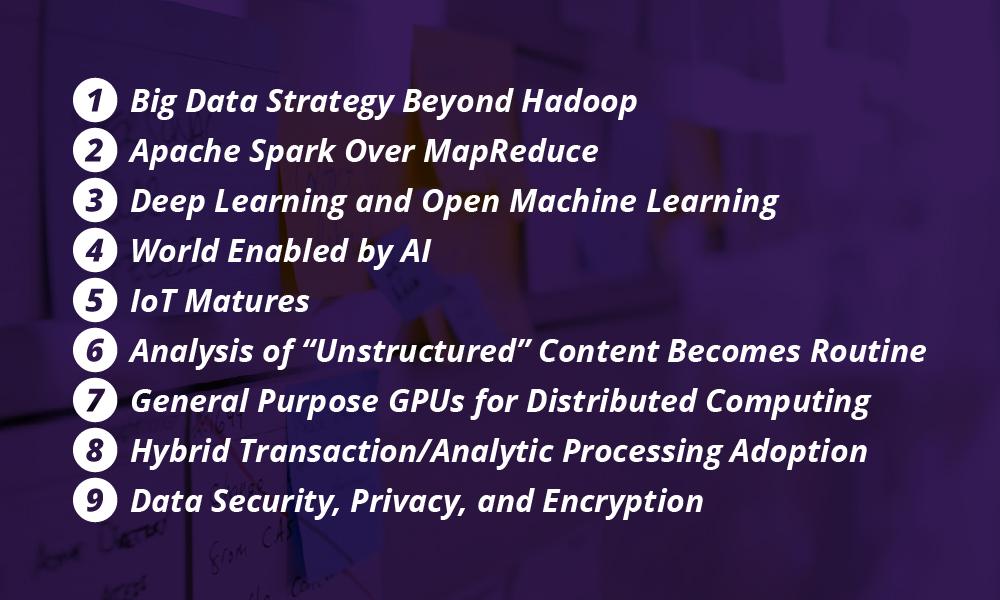
1. Big Data Strategy Beyond Hadoop. After years of rapid technology-focused adoption of Hadoop and related alternatives to conventional databases, we will see a shift toward more business-focused data strategies. These carefully crafted strategies will involve chief data officers (CDOs) and other business leaders, and will be guided by innovation opportunities and the creation of business value from data. The latest generation of exciting advances in data science and data engineering techniques will spark creative business opportunities and the data infrastructure will play a supporting role. Real benefits will best be achieved through the strategic alignment of high value opportunities with the right technologies to support innovative solutions.
2. Apache Spark Over MapReduce. In memory data processing, Apache Spark burst on the scene in 2014 as a Top-Level Apache Project and was the dominate buzz in 2015 as being enterprise ready, and saw significant early adoption. Expect that 2016 will see an explosion of Spark adoption by fast followers and organizations who are seeking to replace legacy data management platforms. Spark on Hadoop YARN is likely to dominate this explosion and will greatly reduce the need for MapReduce processing.
3. Deep Learning and Open Machine Learning. In late 2015, Google open-sourced TensorFlow, its machine learning platform. Just a few weeks later IBM released its machine learning technology, SystemML, into the open source community. These latest projects join a growing plethora of existing open source machine learning platforms such as DL4J (for implementing deep learning in Java). Data scientists and technologists now have, at their fingertips, the world’s leading algorithms for advanced predictive analytics. Expect this to propel the innovative creation of value from data in ways that we’ve never previously imagined.
4. World Enabled by AI. Out of favor since the 1970s, artificial intelligence (AI) is becoming hot again. Examples like autonomous vehicles, facial recognition, stock trading, and medical diagnosis are exciting the imaginations of the current generation of technologists. Moreover, the power of distributed, parallel computing is more accessible than ever before, making it possible to experiment with many novel ideas. At the same time the rich data needed to feed machine learning algorithms is more prolific, diverse, and readily available than ever before. While you may have to wait a few more years to get your self-driving car, you can expect your life to get a little bit better in 2016 because of the innovative uses of AI.
5. IoT Matures. As far back as 1999 Kevin Ashton coined the phrase “internet of things” (IoT), and the world has seen interesting advances in the use of sensors and interconnected devices. The IoT phenomenon has rapidly been gathering steam in recent years with companies like GE, Cisco Systems, and Ericsson contributing. According to Gartner, IoT will include 26 billion operational units by 2020, and IoT product and service providers will generate over $300 billion in incremental revenue as a result.
Expect 2016 to see the embracing of open standards that improve device monitoring, data acquisition and analysis, and overall information sharing. We will also see a divergence in the issues surrounding types of data collected by these devices. Personal, consumer-driven data will increase security and privacy complexities. Enterprise-driven data will increase the complexities of issues like knowledge sharing, storage architectures, and usage patterns.
All of these sensors and devices produce large volumes of data about many things, some of which have never before been monitored. The combination of ever cheaper sensors and devices and the ease with which the collected data can be analyzed will generate an explosion of innovative new products and concepts in 2016.
7. General Purpose GPUs for Distributed Computing. Unlike multiple core CPUs, which have a dozen or so cores, GPUs (Graphics Processing Units) integrate hundreds to thousands of computing cores. GPUs were originally developed to accelerate computationally expensive graphics functions. Recently, however, General Purpose GPU (GP-GPU) adaptations have stretched this technology to handle parallel and distributed tasks. The supercomputer segment has embraced GPU technology as an integral part of computational advancement.
Until 2015, general programming for GPU was intensive, requiring developers to manage the hardware level details of this infrastructure. Nvidia’s CUDA is a parallel computing platform and programming model, however, that provides an API that abstracts the underlying hardware from the program. Additionally, Khronos Group’s Open Computing Language (OpenCL) is a framework for writing programs that execute across heterogeneous platforms consisting of CPUs, GPUs, as well as digital signal processors (DSPs), field-programmable gate arrays (FPGAs) and other processors or hardware accelerators.
With these programming abstractions comes the realistic ability for many organizations to consider GPU infrastructure rather than (or in addition to) CPU compute clusters. Look for the combination of open source cloud computing software such as OpenStack and Cloud Foundry to enable the use of GPU hardware to build private and public cloud computing platforms.
In 2014 Gartner coined the acronym HTAP (Hybrid Transaction/Analytic Processing) to describe a new type of technology that supports both operational and analytical use cases without any additional data management infrastructure. HTAP enables the real-time detection of trends and signals that enables rapid and immediate response. HTAP can enable retailers to quickly identify items that are trending as best-sellers within the past hour and immediately create customized offers for that item.
Conventional DBMS technologies are not capable of supporting HTAP due to their inherent locking contention and inability to scale (I/O and memory). However, the emergence of NewSQL technologies couples the performance and scalability of NoSQL technologies with the ACID properties of tradtional DBMS technologies to enable this hybrid ability to handle OLTP, OLAP, and other analytical queries. HTAP functionality is offered by database companies, such as MemSQL, VoltDB, NuoDB and InfinitumDB. Expect to see the adoption of these technologies by organizations looking to avoid the complexities of separate data management solutions.
Disclaimer: The statements and opinions expressed in this article are those of the author(s) and do not necessarily reflect the positions of Thoughtworks.