Perspectives
Introduction: Higher speeds, higher stakes
The pressures that surround product development have ramped up significantly. Demands for speed and scale have always been present, but in a tougher economic environment, resources shrink even as the stakes rise.
“Circumstances are causing a shift towards what we call value-driven ways of working,” says Farooq Ali, Principal, Product Strategy & Delivery at Thoughtworks. “There’s greater scrutiny on ROI, and more emphasis than ever on aligning product work with customer and business outcomes. A second order effect is a shift towards leaner product teams and processes, with people starting to think about how to accomplish more with less.”
At the same time the development environment is growing more intricate as companies strive for differentiation and products incorporate more features.
“What’s really important is identifying that unique value proposition for a product, and creating a product roadmap to actually maintain a sustainable competitive advantage,” says Rujia Wang, Thoughtworks’ Head of Customer Experience, Product and Design.
“Today’s challenge isn’t about adding new ideas; it’s how you scale (given) the dependencies and complexity in the product ecosystem,” Zichuan Xiong, Head of Generative AI Product, Thoughtworks, says.
Even as AI presents problems for product teams struggling to meet simultaneous demands for desirability, viability, feasibility, usability and responsibility, they’re increasingly looking to it – and generative AI (GenAI) in particular - as the solution. One survey of close to 600 companies across 11 industries found one-third are already actively exploring the use of GenAI in product design and simulation. Market research suggests that the product development market for GenAI will balloon to US$1 billion by 2032.
Generative AI adoption in product development set to accelerate through 2032
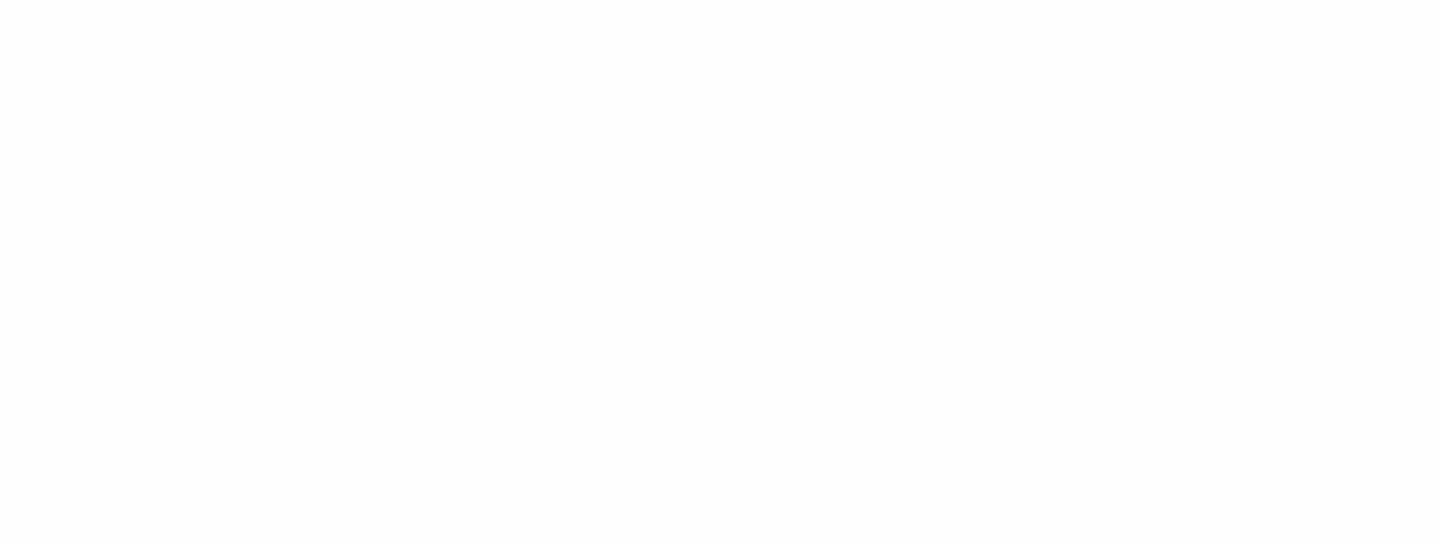
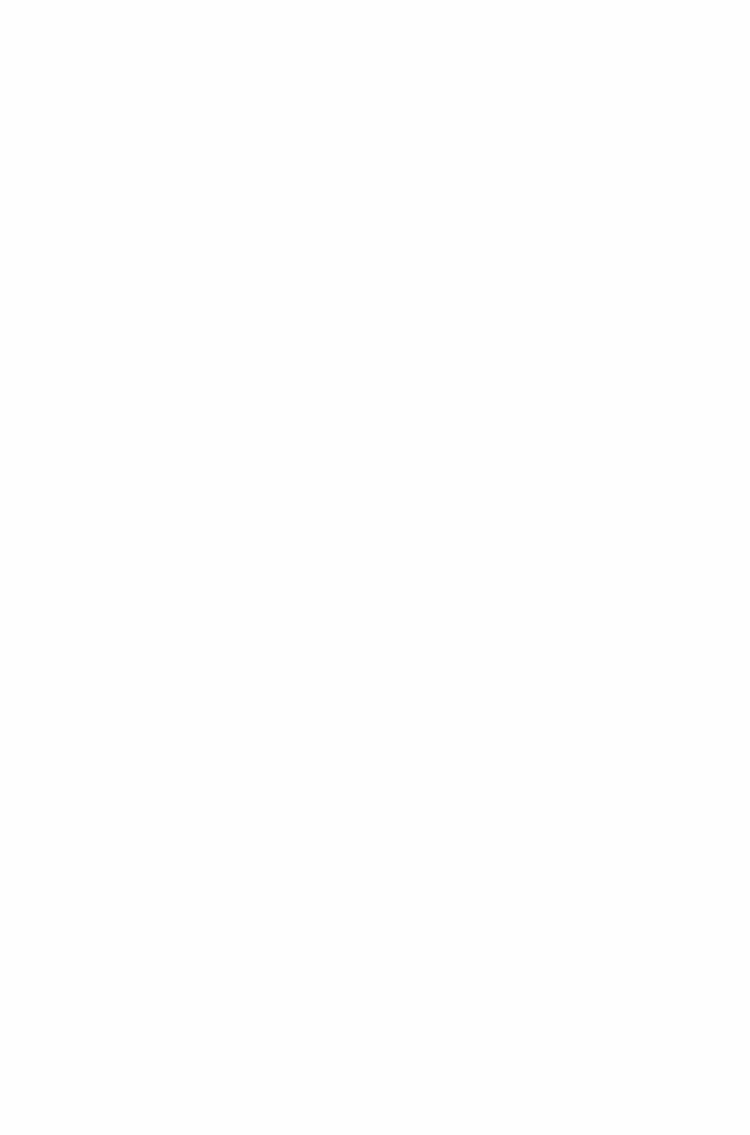
At least in theory, the capacity for GenAI to supplement product development is enormous. Companies have long used automation to reduce some of the more mundane aspects of the development burden. But with GenAI, the expectation is that by extending even further – and more deeply – into more creative facets of the process, teams will be freer to focus on the most critical product features and innovations, contributing more directly and substantially to business value.
“GenAI has the potential to sort through a lot of the complexities of product development for us, because it can consider thousands of data points on customer interactions and market signals that would take a human possibly months to make sense of,” notes Wang.
“It can reduce the cost of traditional prototyping to near zero,” adds Xiong. “For example, you can create an application and task GenAI with figuring out if you add certain features, how it impacts your portfolio based on knowledge of other portfolio values, because it’s exceptionally good at identifying patterns, and curating new findings by using natural language.”
“Outlearning your competition is the new competitive advantage,” says Ali. “The rate at which you can learn about and from the market allows you to de-risk your product decisions. And GenAI can augment and enable a lot of that.”
Yet, as with all new tools, there’s a degree of uncertainty about exactly how GenAI should be applied – and mounting awareness that, left unchecked, its impacts may not be entirely positive.
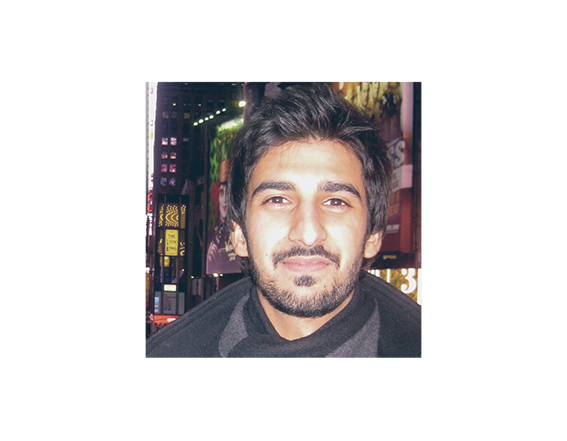
“Outlearning your competition is the new competitive advantage. The rate at which you can learn about and from the market allows you to de-risk your product decisions. And GenAI can augment and enable a lot of that.”
Farooq Ali
Principal, Product Strategy & Delivery, Thoughtworks
i. Augmentation along the product development spectrum
As Ali notes, the product development value chain can be broadly broken down into a few key stages:
Research and analysis
Ideation and planning
Design and development
Testing and feedback
Production and deployment
Launch and marketing
Customer support
Most, if not all, of these stages are “ripe for innovation with GenAI,” Ali says.
This is in part because GenAI is a genuine sea change. “The main difference with what came before is that GenAI is creative, and in turn, enhances the creativity of both product and design practitioners,” says Wang. “It can be used throughout the entire product development process, from product definition to solution design, to build, launch, and evolution.”
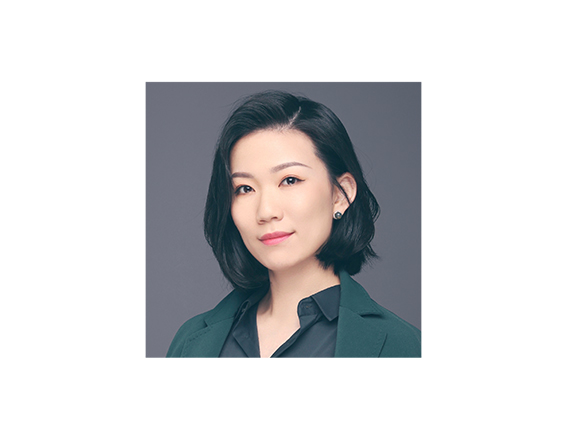
“(GenAI) can be used throughout the entire product development process, from product definition to solution design, to build, launch, and evolution.”
Rujia Wang
Head of Customer Experience, Product and Design, Thoughtworks
In the research and analysis stage, GenAI amplifies the organization’s ability to find, collect and analyze pertinent data on market conditions and customer preferences exponentially – and perhaps even more importantly, can introduce new perspectives.
“AI can quickly synthesize vast amounts of information to provide a coherent understanding of market trends, signals that are in the market and customer needs,” Ali explains. “But we always end up resorting to selection bias when we're thinking about what to focus on. What AI can really do is give us a broader aperture, and an ability to scale the analysis and synthesis of that research.”
For example, with one client, Thoughtworks was looking at opportunities around the emerging field of generative business intelligence, and trying to map out the competitive landscape. We used Boba, an experimental idea generation copilot, to research how other companies are using GenAI for business intelligence – and within a matter of seconds, got a list of all those exploring the space without resorting to potentially expensive market research.
Another example is in customer interviews – a frequent tactic to understand their roles, pains and gains so these can be fed into product design.
“Interviews generate a lot of notes, recordings, and so on,” Ali says. “There are now tools that allow you to summarize interview recordings into key insights from the conversation and cluster them into themes, which can then be explored further by researchers.”
When it comes to ideation and planning, AI is partnering with humans to support the, at times, agonizing early stages of the creative process.
“GenAI can be used everywhere, but it’s in the solution design part of the product development process where I think we’ll get the most short-term upside,” says Wang. “It's really lowering the marginal cost of imagination, ideas, visualization and communication. GenAI solves the blank page problem that we often encounter at the beginning of the creative process. It expands our thinking on what’s possible by giving us a whole host of ideas to explore and build on.”
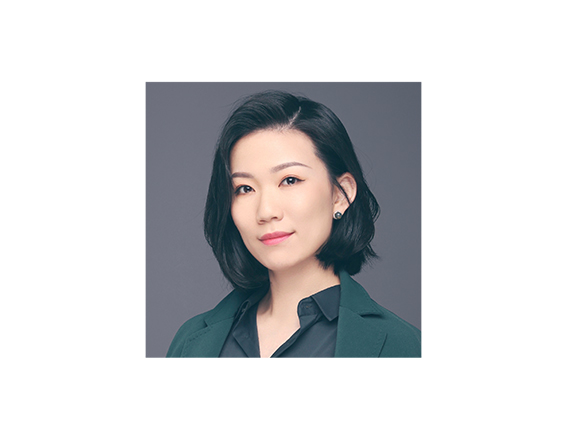
"GenAI solves the blank page problem that we often encounter at the beginning of the creative process. It expands our thinking on what’s possible by giving us a whole host of ideas to explore and build on.”
Rujia Wang
Head of Customer Experience, Product and Design, Thoughtworks
One case in point is snack giant Mondelez, which employs AI to suggest new product recipes that are then shortlisted and vetted by human taste-testers. “What's really cool about this approach is that far from replacing human creativity, these algorithms serve as catalysts,” says Ali. “They're able to spark new ideas that human minds haven't considered yet. They’re not perfect, but no one expects human creative experts to come up with great ideas 100% of the time.”
Even when ideas come easily, pre-GenAI “it still took a lot of effort to visualize and communicate them,” says Wang. “You had to be rigorous about prioritizing because you were limited in how many ideas could feasibly be taken to the next stage. But now you can move some of that heavy lifting of visualizing ideas to GenAI, creating low, medium, and even high-fidelity wireframes, at almost zero marginal cost compared to what it would take for a human. That way, you can keep that divergence part of the ideation process open longer, spend more time really considering all the alternatives – and in interrogating those ideas, you might come up with something that's even better.”
By enabling rapid prototyping and interface design, GenAI makes it easier to evaluate ideas before teams invest too much in code. This creates efficiencies that, in turn, pave the way for benefits in later phases of the product development journey like launch and marketing.
“It doesn’t just reduce the burden of operational tasks; product engineers and product professionals can focus more on working with the customers, talking to them, going to the market, figuring out real problems,” says Xiong. “Byproducts like customer service analytics or an SEO plan can be produced by AI, so people can focus on the number one priority, which is customer benefit and realization of customer value.”
However, use cases can also depend to an extent on the industry. Those where content generation is directly connected to revenue and which don’t face much in the way of regulatory constraints – such as gaming – “can be rapid and high-maturity adopters who apply GenAI tools at every single step of their product lifecycle,” Xiong says. Sectors where intellectual property and privacy issues are more at the forefront, and the consequences of disinformation potentially devastating – like financial services – are likely to have to adopt a more measured approach.
ii. Best practices and principles; balancing the AI and human roles
Companies tend to overestimate the effort involved in using newer GenAI tools due to presumptions stemming from a lack of familiarity with the technology – like the mistaken notion it requires advanced skills, or vast amounts of high-quality data.
Unlike classic machine learning models, which require good data input to be trained and have traditionally been hard to scale because of data quality problems, GenAI tools are capable of filling in blanks and creating data of their own. This enables organizations to start augmenting their development process without massive data investments.
Take, for example, Genius, Figma’s auto-complete design assistant, a copilot that develops an understanding of the designer’s product design system and recommends possible solutions as they build out an interface. Jira, a popular project management tool, is another example of GenAI’s low barrier to adoption. Its Atlassian Intelligence AI copilot functions like a ’virtual teammate,’ helping summarize meeting outputs and define workflows.
The real challenge to GenAI fluency is “the need for a change in design mentality,” says Xiong. Designers tend to follow tried-and-true principles, which might be based on environments that are becoming less relevant – much as how UIs were still being tailored for large screens as the world was shifting to mobile.
“In future, we’re going to have fewer graphical user interfaces (GUI) like a form submission from one system to another one, because large language models will dominate the information transaction between systems ,” Xiong explains. “As transactional applications may now be zero-interface, the question arises of how you generate and validate ideas, and design solutions for that.”
Wang points out that making the most of GenAI depends on learning how to interact with it in an optimal way.
“It takes time to learn how to talk and work with GenAI and make it a true creative thinking partner,” she says. “One tactic is to get it to ask you questions and to give it more context to create a better list of ideas, or asking it to think about what has to be true for an idea to be successful, instead of asking it for direct answers. Some of that interaction might feel unnatural, but to get a better outcome, it's important to ask better questions.”
With the availability of GenAI as a copilot, it’s also vital to understand its limits. Humans still have a much bigger role to play in defining the problems the business is trying to solve with AI throughout the product development cycle, which requires a deep understanding of business goals.
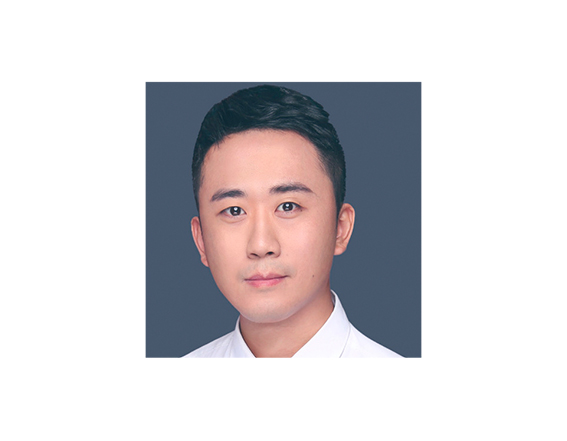
“Design is not just a one-time job; it’s people spending time to find the optimal solution, adjusting along the way and using different tools to do that. Going through the customer journey again, doing iterations, is not as easy as people think. You still need human beings, all the expertise, experience and knowledge we have. [AI] is just a tool and shouldn’t be presented as the solution to all problems.”
Zichuan Xiong
Head of Generative AI Product, Thoughtworks
“Some people have the fantasy that they can feed things into a prompt engine and get good results, but it’s only good designers that know how to translate ideas into words, and words into products,” notes Xiong. “Design is not just a one-time job; it’s people spending time to find the optimal solution, adjusting along the way and using different tools to do that. Going through the customer journey again, doing iterations, is not as easy as people think. You still need human beings, all the expertise, experience and knowledge we have. [AI] is just a tool and shouldn’t be presented as the solution to all problems.”
“While synthetic users – users that are built on historical models and patterns of real customers – can be deployed to assist with certain design tasks, they have their limitations,” says Wang. “For one, we don't always know about the biases and issues with the data that goes into them, or if the results are really insightful for understanding the problem that we are trying to solve. Ultimately, humans are still better at understanding what other humans need.”
Human input is also highly valuable in areas where GenAI may have knowledge gaps, such as product validation.
The knowledge base some tools are built on “may not include the latest events, or the latest regulatory changes,” Wang says. “[GenAI] also doesn't know what competitors have been doing recently. Humans need to come in and use domain knowledge to validate AI’s outputs.”
“Validating results requires human product designers to learn the end-user knowledge and work with end-users to verify that the generated content is valuable, consistent, predictable, and bias-free,” agrees Xiong.
In essence, according to Ali, there are three levels of maturity or collaboration with AI – AI informing product decisions, AI augmenting product decisions, and AI automating product decisions.
“You have to look at your product development value stream, break it down into where you make key decisions, and then ask yourself the question: Should we be informing this product decision with AI? Should we be augmenting? Or should we be automating?,” he says. “The answers will be different for every company.”
AI collaboration maturity model
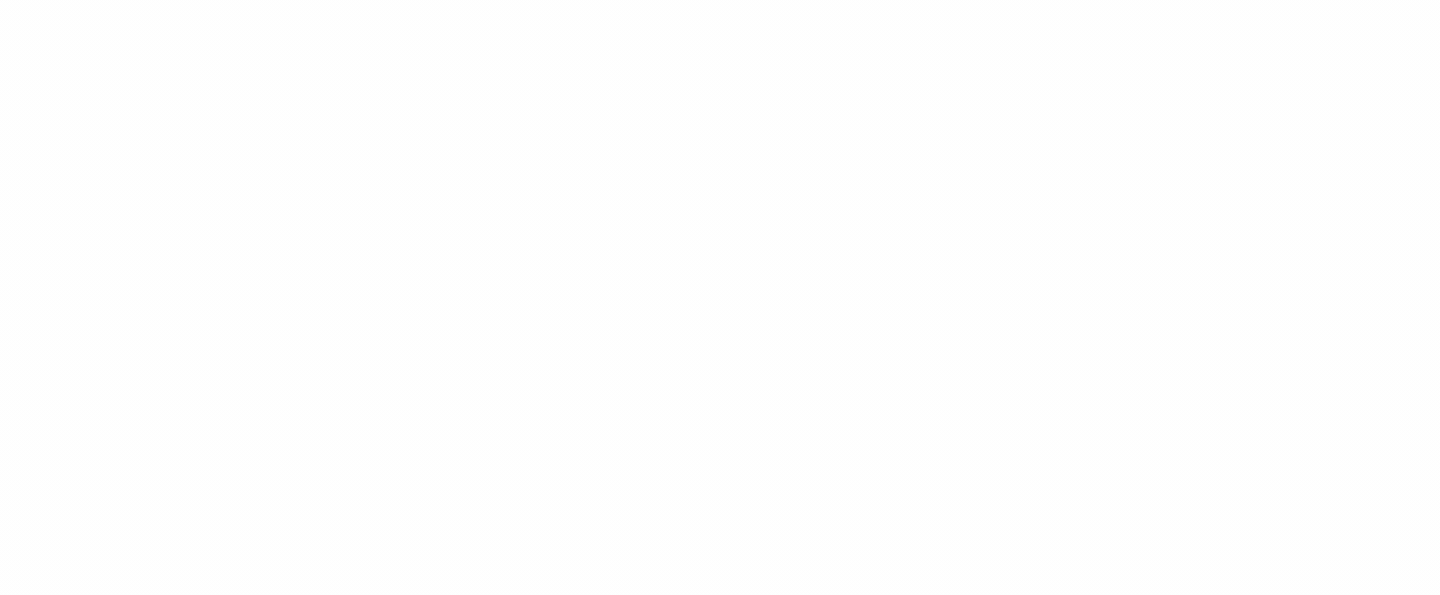
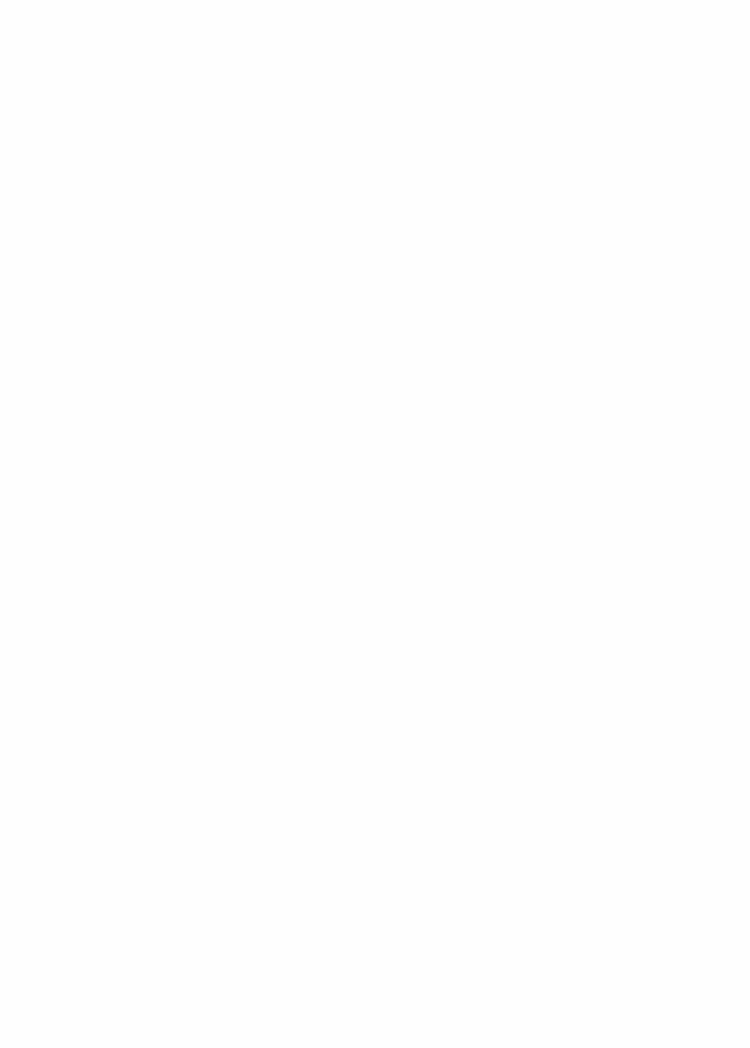
AI’s ability to uncover and recommend insights that inform the human decision-making process can prove useful in situations such as A/B testing, but organizations should pause and consider the tradeoffs before making decisions based purely on AI-generated results.
“You might want to preserve human judgment and creativity in areas like testing because it’s one of your differentiators, and AI is no replacement for it,” says Ali. “A really good example of this is talking to customers. AI will eventually be able to talk to customers and gather more data at scale. But do you really want to do that? Or do you want to build a relationship with customers and involve them in the process? It's not necessarily a good idea to automate something with AI just because you can.”
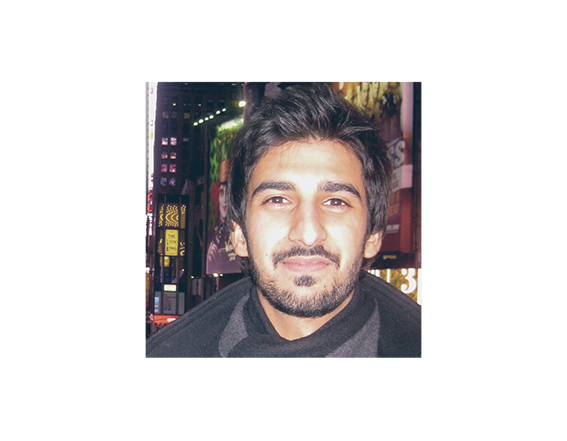
"AI will eventually be able to talk to customers and gather more data at scale. But do you really want to do that? Or do you want to build a relationship with customers and involve them in the process? It's not necessarily a good idea to automate something with AI just because you can.”
Farooq Ali
Principal, Product Strategy & Delivery, Thoughtworks
Despite these considerations, there is no denying that AI is altering the way humans fit into the product development equation.
“The marginal cost of creating a first draft is approaching zero, and our roles are evolving from creators to creative directors,” Ali explains. “We're going to be spending a lot more time directing the AI, curating its output, and actually iterating and collaborating with AI. Though the ultimate creative direction will rest with humans, the speed and volume of those ideas and implementation will naturally demand we do a better job of curating.”
This means the ability to work with AI will essentially become part of everyone’s job description in the future.
“While there are organizations hiring specifically for prompt engineers, all members of the product development team should explore how to collaborate with AI and learn prompt engineering to get the best out of it,” Wang says. “With a combination of good critical thinking, problem-solving, and linguistic skills, the product manager, designer and tech lead can all be prompt engineers.”
In this new scheme of things, the involvement of domain experts will become even more consequential.
“We've always advocated for the business to be deeply embedded in our cross-disciplinary teams, because it lowers the cost of communication, and ensures we are building the right thing,” Wang says. “An understanding of the business domain is important for improving the quality of the problems that we are asking AI to help us solve, and therefore, the inclusion of business domain expertise is more critical than ever.”
In addition to providing the necessary business validation, domain experts may now be able to boost their involvement in the creative process. “There’s a democratization of design taking place where the bar is being lowered for the skills that you need to actually participate,” says Ali.
According to Wang, “a multidisciplinary team of people who understand humans, people who understand technology, and data, and people who understand the business will enable organizations to get the most out of AI. At its core, it's about understanding these different contexts.”
“Depending on industry, access to legal and regulatory experts outside of the product development team is also important to ensure compliance with relevant laws and regulations, such as GDPR, or rules governing the provision of financial advice,” she adds.
“New generations of product designers should be open, brave, and visionary to invent new design principles, try new tools, and use the tools to shape new design behaviors,” says Xiong. “Design eventually is a social activity that evolves together with how human society evolves.”
iii. Avoiding negative consequences
As organizations explore new creative horizons with GenAI, it’s becoming more important to build awareness of the new risks it presents, and find ways to mitigate them.
Xiong notes as GenAI gains multimodal capabilities, its speed and efficiency may be mistaken for authority, making it more difficult to discern reality from AI-generated hallucinations.
“There is the potential of a huge domino effect when in the near future, among hundreds of AI agents networking together, one agent makes a mistake, and the mistake is sugarcoated by another, which will create a series of downstream consequences,” he explains.
“If you’re using AI in decision making, you should be able to explain why the AI recommended a certain decision,” Ali points out. “And the reality of the current models is that they're very opaque. We know how we build them, but we don't know how they reason.”
AI’s struggle with explainability can be compared to how humans arrive at an answer, notes Wang.
“Maybe it was based on experience, maybe it was based on our intuition,” she says. “Using GenAI in the product development process, we'll meet very similar challenges where we will arrive at a conclusion that we don't really know how to explain. In that case, the key consideration is whether we can challenge the conclusions from GenAI.”
IP and copyright considerations are also contributing to organizations’ hesitation to adopt and implement AI in product development.
“While it's tempting to use off-the-shelf AI products and models, we’re not allowed, from the clients’ perspective, to take a bunch of interview material, customer data and put them into an external tool until it's gone through proper vetting – and by that time the project's already finished,” Ali notes. “Even though an AI tool provides some capability, you have to factor in some time to understand where the data is going and how it’s being used.”
Precisely because GenAI is trained on external data that’s easily available to everyone – including competitors – it makes the case for organizations to incorporate proprietary data into AI models where possible. Data also needs to be vetted for bias – which is not limited to public data but can extend to the organizations’ own resources.
“Your customer data could be skewed towards one demographic over another,” Ali points out. “When you are in that situation, you may need to make deliberate decisions to augment your data, whether it's with synthetic data, or by going out and doing deliberate interviews with customers of other demographics.”
“Research and testing with real users continue to be the most important parts of the product development process,” Wang says. “That includes testing with diverse groups to identify potential biases that have been introduced by using GenAI.”
In the vast majority of consumer use cases, Xiong says, governance issues will pose a far bigger challenge than ethical lapses.
“The governance challenge isn’t governing AI; it’s about governing AI-generated content,” he says. “The existing operating system to do content reviews, the editorial process – think of compliance, privacy, security, scanning everything – once GenAI adoption goes up, that workload will go up as well.”
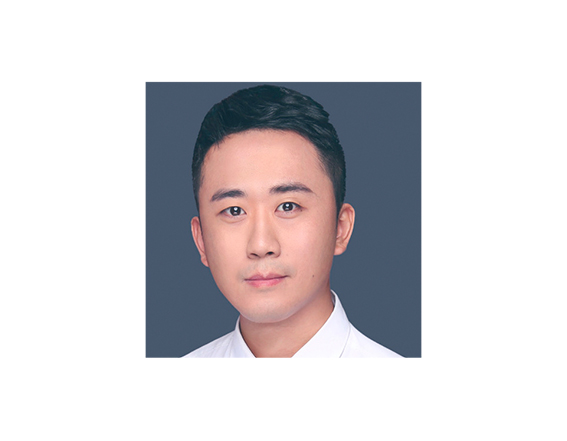
“The governance challenge isn’t governing AI; it’s about governing AI-generated content. The existing operating system to do content reviews, the editorial process – think of compliance, privacy, security, scanning everything – once GenAI adoption goes up, that workload will go up as well.”
Zichuan Xiong
Head of Generative AI Product, Thoughtworks
The good news is AI also has the potential to solve some of the governance problems it creates. “For example, you could use your compliance or content policy as input for a large language model that can then conduct content reviews,” Xiong explains.
The most effective guardrails for AI governance are embedding ethical and responsible AI principles into the culture of the company, including how it builds customer experiences, notes Wang. Some of these are outlined in a collection of tools, methods and frameworks Thoughtworks has created to help organizations navigate the complex but essential sphere of building responsible tech.
Deliberate steps can also be introduced into the product development process to ensure greater adherence to responsible AI practices.
“GenAI is expanding the possibilities in and accelerating the product development process,” says Wang. “But by intentionally introducing friction, for example, making it a default practice for humans and AI to explore the possible negative consequences of a solution or why the solution wouldn't work, we can make more considered and deliberate decisions.”
As with any form of innovation, “borrowing ideas from others is a natural part of the human creative process, although it becomes more pronounced with GenAI,” she adds. “The key is to build on top of the ideas from GenAI instead of taking them at face value or using them as is. That's why humans will still be incredibly important in the whole product development process.”
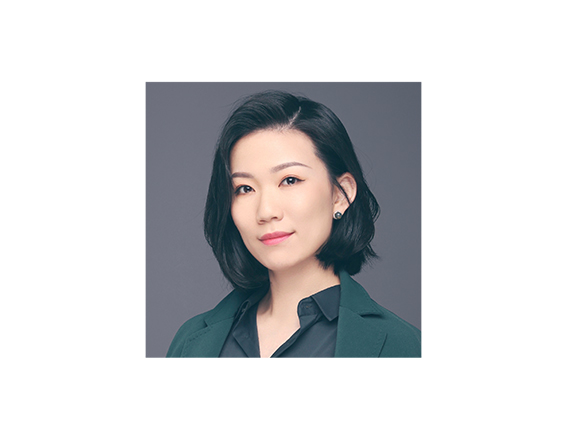
“The key is to build on top of the ideas from GenAI instead of taking them at face value or using them as is. That's why humans will still be incredibly important in the whole product development process.”
Rujia Wang
Head of Customer Experience, Product and Design, Thoughtworks
iv. How the use of generative AI will evolve
GenAI’s evolution and growing presence are expected to transform not only product development, but the nature of applications themselves and the roles of the people who create them.
“If a large language model is adopted throughout the entire product lifecycle, there’s a potential to merge roles, so you don't really differentiate the designer versus the product manager versus the business analyst,” says Xiong. “You won’t need different roles to translate business requirements into product specs, or product specs into a user story, or the user story into an interface. In the future, you can use models to translate requirements from different formats, to navigate the space in ambiguous language to connect the problem (business) and solution (design) domains.”
At the same time, we can expect to use fewer applications in future. “The reason we have so many is because of the limitations of software itself; every software solution has a closed scope, and when you want a new scope, you have to create it,” Xiong points out. “With traditional software products, the scope of work is shrinking, but there are new possibilities with the building of AI applications – which, if you’re a project manager, argues for focusing on that space.”
Organizations attempting to create a more AI-driven workforce will have to look beyond traditional software development and start giving product development teams more opportunities to build and experiment with innovative AI solutions.
“Being able to interact effectively with GenAI will soon be a bare minimum skill that you expect, just like when Google came out, you had to eventually learn how to search,” says Ali. “You're going to see domain experts work more closely in product development, designing prompts that interact with the system to build an AI-powered application. Stretching that out, I see interdisciplinary convergence, the culmination of data science, behavioral economics, even philosophy, and other fields coming together and being more involved in product development.”
Design methodology, too, will have to change to keep pace with these advancements.
“In design thinking, you have three big circles – the viable, the desirable and the feasible – but with AI, fair use and responsibility will need to be added to that,” says Xiong. “You also have to upgrade your existing service blueprint design as a methodology, because AI is going to be a new user. You’ll have to design the AI as you're designing the human agent, and try to design a relationship between the AI agent and the human agent so they’re working together to execute a job and help customers. When you design that way, you don’t think of AI as a system; you think of AI as an intelligent individual.”
Another exciting area that’s emerging is simulated prototyping. “This is old hat for manufacturing; because they’re dealing with physics, it's a lot easier to generate designs that you can test against different criteria,” says Ali. “The problem in building software is you're building for humans, and being able to model humans is a very difficult problem. Also, just because you can generate a lot of ideas and prototypes, doesn't mean you can evaluate them really rapidly too. Once people figure out how they can rapidly evaluate through simulation and simulated testing of ideas, it’s going to open up a whole new world in terms of generating new features and product ideas.”
Wang sees both divergence and convergence in the way GenAI is applied across industries.
“Industries that are more data rich may have more opportunities to transform because they will have more powerful models, and GenAI models will become more specialized in particular industries to be able to solve problems better,” she says. “But overall, every single industry will be affected in some way. I prefer to be an optimist and say that they will be transformed for the better. It’s also our responsibility as product designers to create that future as well and not just wait for it to happen.”
In the larger scheme of things, GenAI’s potential to draw more people into the development process, break the boundaries around their roles, and exceed previous limitations on scale and speed, brings with it the prospect of enhancing the organization’s collective ability to make effective choices around the products it creates.
“A lot of product decisions right now are centralized within the product team,” notes Ali. “We’re already starting to see the decentralization of data, but when you start seeing the decentralization of intelligence, you’re going to see distributed decision-making take place – strategy not being something that's just top down, but spread all the way from senior leadership to the customer service representatives, in the choices that they make.”
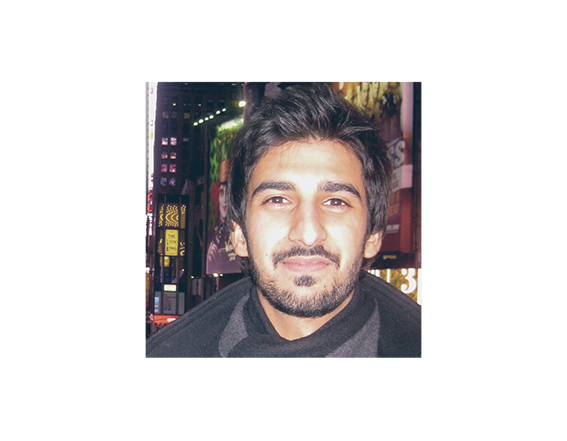
“A lot of product decisions right now are centralized within the product team. We’re already starting to see the decentralization of data, but when you start seeing the decentralization of intelligence [enabled by GenAI], you’re going to see distributed decision-making take place.”
Farooq Ali
Principal, Product Strategy & Delivery, Thoughtworks
Perspectives delivered to your inbox
Timely business and industry insights for digital leaders.
The Perspectives subscription brings you our experts’ best podcasts, articles, videos and events to expand upon our popular Perspectives publication.