New AI-driven approaches to dynamic pricing have shown to be effective and generate increased revenue compared to traditional approaches. Organizations that aren’t up to speed with these new technologies are at risk of seeing diminishing returns due to pricing inefficiencies while their competitors continue to grow market share.
At Thoughtworks we have helped our customers to apply AI-driven dynamic pricing with proven results. For example, we helped Forenom — a Nordic serviced apartment company — to achieve a 13% increase in revenue per available room for locations where AI-driven dynamic pricing was used.
Real-time dynamic pricing with online reinforcement learning
The more dynamic and real-time the price is the more there is increased revenue potential. To get more business, the key is to match the dynamic price with demand and supply in the market. The price can be lower or higher than normally if the volume and price leads to higher total revenue. With our approach to AI-driven dynamic pricing, which is based on online reinforcement learning, we are able to seamlessly introduce real-time pricing adjustments that accurately mirror the ever-changing market conditions.
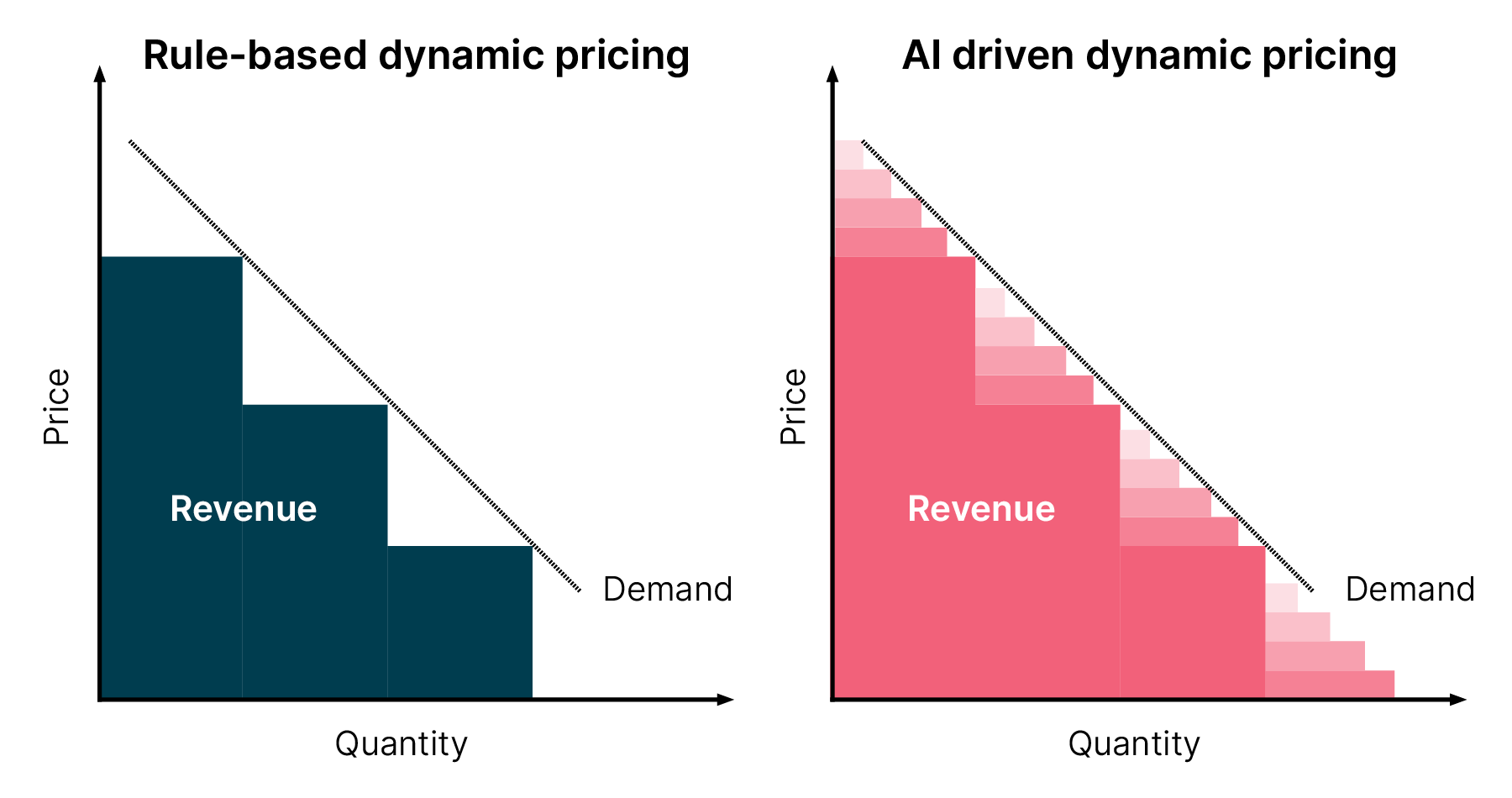
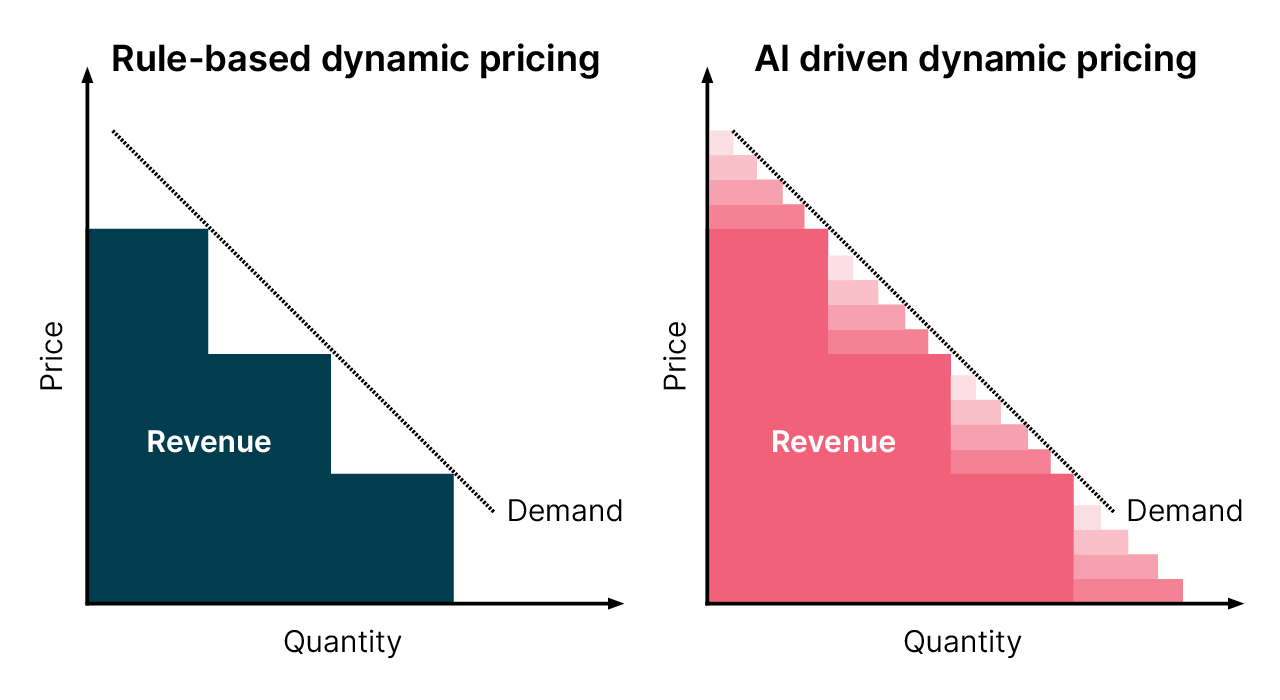
Traditional approaches to dynamic pricing that are based on rules or supervised learning have limitations that online reinforcement learning can overcome. Rule-based solutions rely on static rules that can introduce some degree of pricing dynamics, but they aren’t as dynamic as machine learning approaches. Supervised learning, meanwhile, can learn complex dynamics from historical data, but it isn’t able to adapt to disruptions in the market. Online reinforcement learning is different in that it can rapidly adapt to ever changing price elasticity through rapid and real-time automated price experimentation, avoiding reliance on historical data.
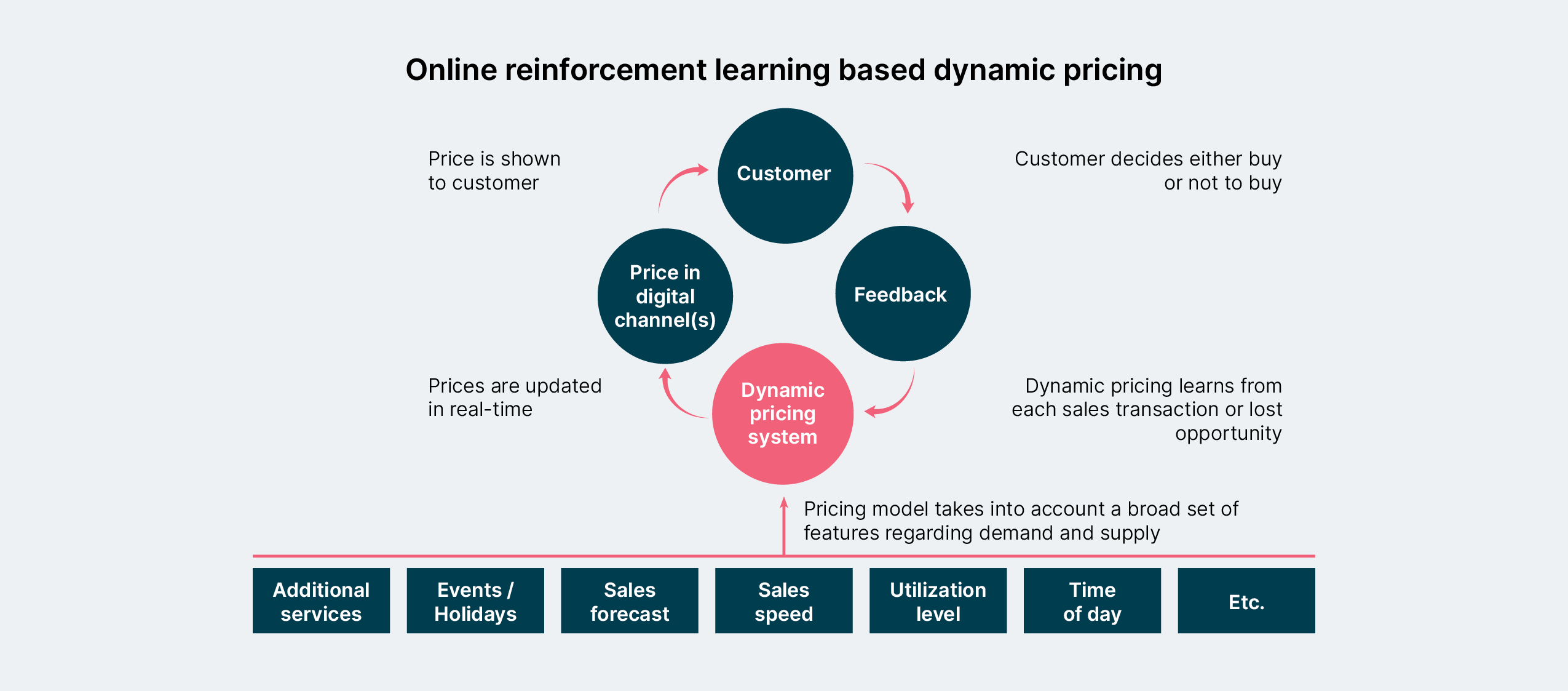
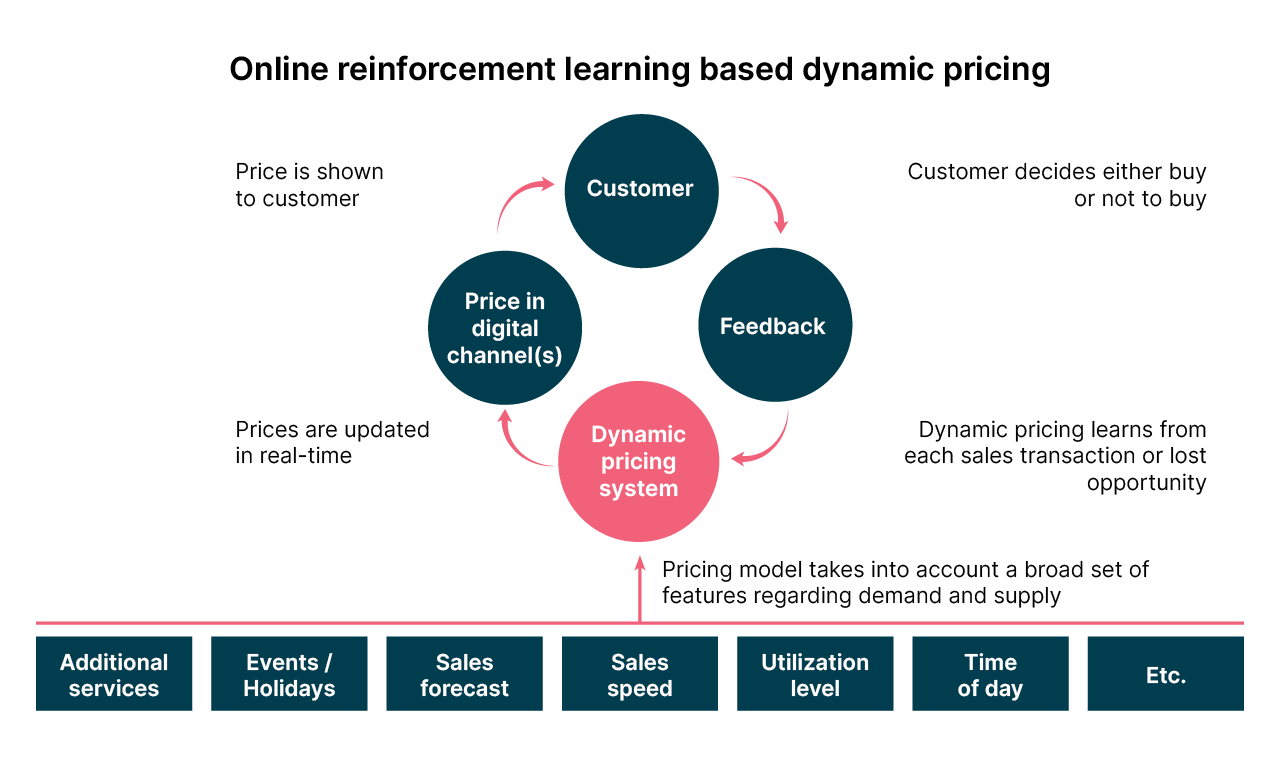
One example of a change in price elasticity is that during 2022 we saw disruption in the supply chain of car components, which led to used car price increases. This was due to decreased supply, since new cars were hard to get or had extended delivery times. This was something historical data could not capture: any approach based on the past would inevitably yield poor pricing recommendations.
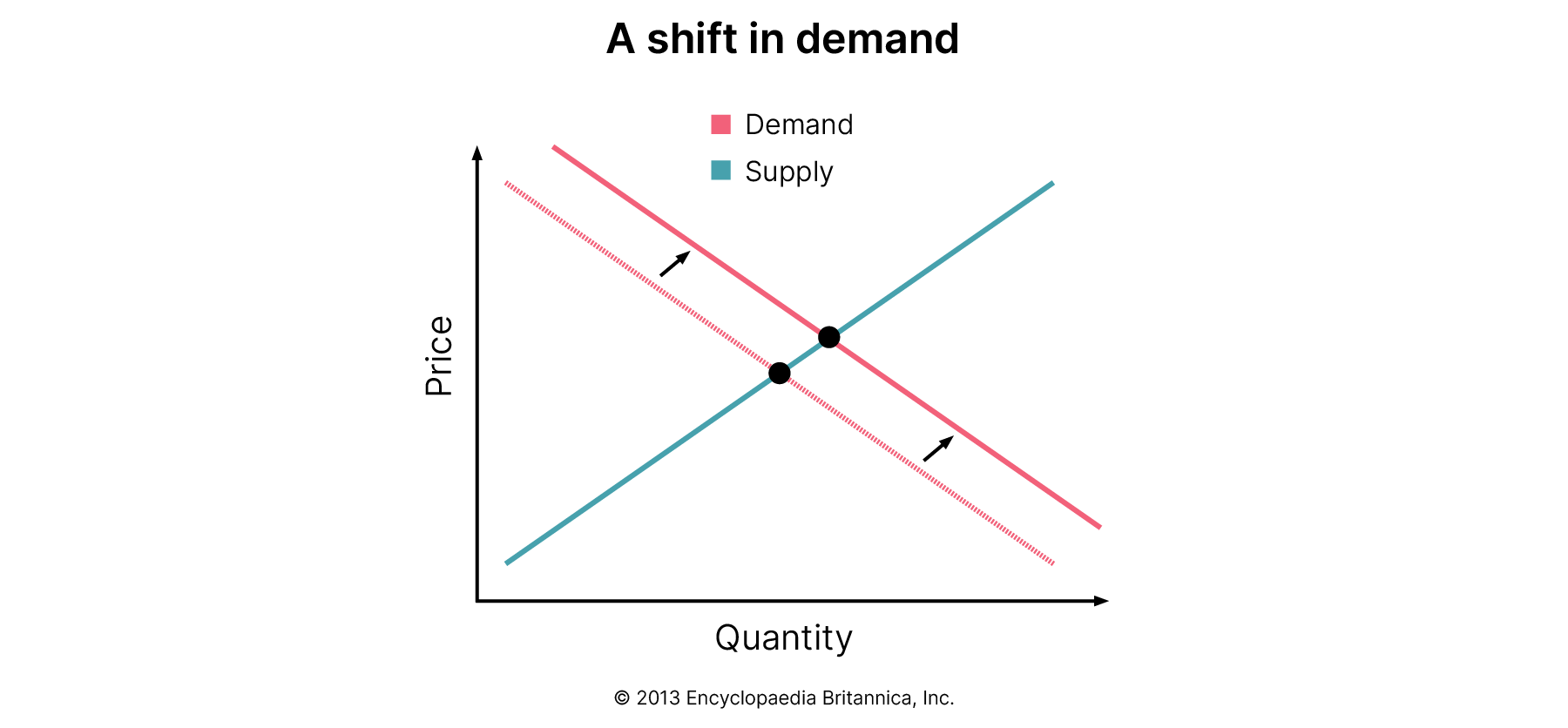
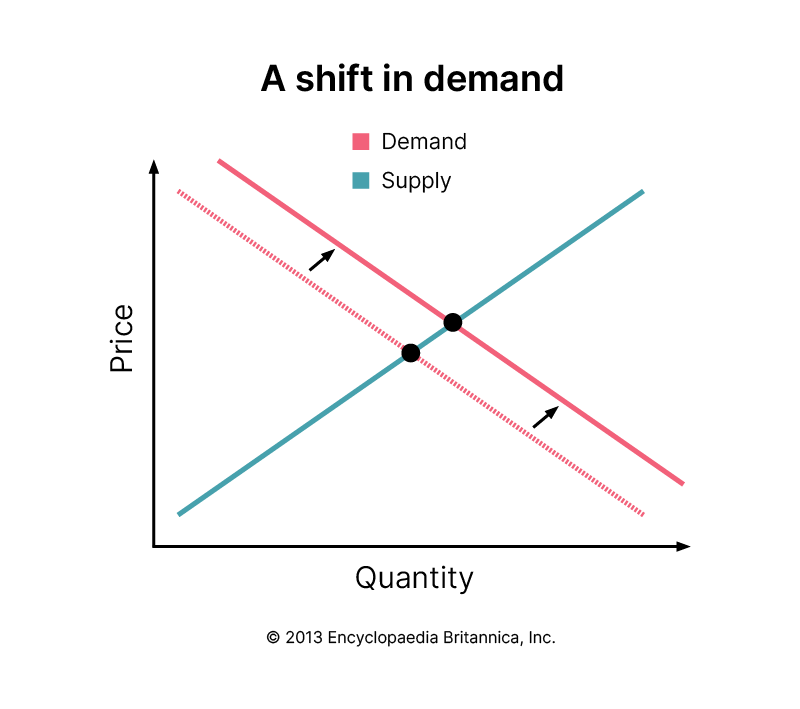
Increased revenue and operational efficiency
In addition to serviced apartments we have proven that the same AI-driven dynamic pricing approach works across industries. We’ve applied the same approach to mobile subscriptions, used cars, car parking at airports, fuel at gas stations, transportation as a service to name just a few. For example we delivered a 12% increase in average revenue per user for mobile subscriptions for a Nordic telecom operator and for car park pre-booking at an airport, a five percent increase in revenue per session compared to the market leading solution IDeaS RMS.
Increased revenue isn’t the only benefit of the solution; it also improves operational efficiency through automation. By using AI-driven dynamic pricing to automate tactical pricing, organizations can spend more time on strategic decisions around pricing, like deciding what new data could improve the dynamic pricing engine or focusing on the customer experience around pricing. Automated pricing also allows growth companies to focus on other growth challenges.
Start small without the need for historical data
Start small with AI-driven dynamic pricing and measure early revenue increases before scaling up to validate further investment. Remember to also use A/B testing to measure the added value of a new pricing strategy on online platforms.
Decide whether to buy or build when choosing a dynamic pricing solution. Purchasing a solution offers quick adoption but can lead to diminishing competitive advantage if competitors choose the same approach. Building a custom solution is key to competitive advantage despite higher upfront costs. Some prioritize a strategic asset for data security.
Use an online reinforcement learning based approach to dynamic pricing. With this approach organizations don’t need historical pricing data due to the nature of the approach which uses price experimentation to rapidly learn the price point in the market. It learns quickly when there is a vast amount of transactions in a short time period.
Perform an AI gap analysis to identify and mitigate obstacles. Organizations may encounter various technical and organizational obstacles when adopting AI-driven dynamic pricing. Identifying and addressing these barriers and root causes that might hinder the development and adoption of AI driven dynamic pricing is key to success.
At a time of increased competition it’s time to upgrade your dynamic pricing practice to a modern AI-driven approach that increases revenue, improves operational efficiency and creates a long-term competitive advantage. Make sure you address potential barriers to development and adoption, remember to start small and measure results to justify continued investments into a scaled dynamic pricing operation.
Disclaimer: The statements and opinions expressed in this article are those of the author(s) and do not necessarily reflect the positions of Thoughtworks.