Something to remember as we are inundated with generative AI tools to aid in our work – “I understand this is a ‘generative’ tool and will generate content that may or may not be factual or accurate. I will use this tool to augment my work and not solely rely on it to do my work.”
Everyone considering generative AI, including Large Language Models (LLMs), should be asked to sign a declaration like what I have shared above, before being enticed by the possibilities of what could be. The objective is to remember the tech is a generative model and not an oracle.
While LLMs have broken a barrier on the frontiers of AI and can be an excellent tool, I believe people harbor unrealistic expectations of this technology. The assumptions about the model’s intelligence and its ability to replace humans are misguided and come from not knowing how the technology works.
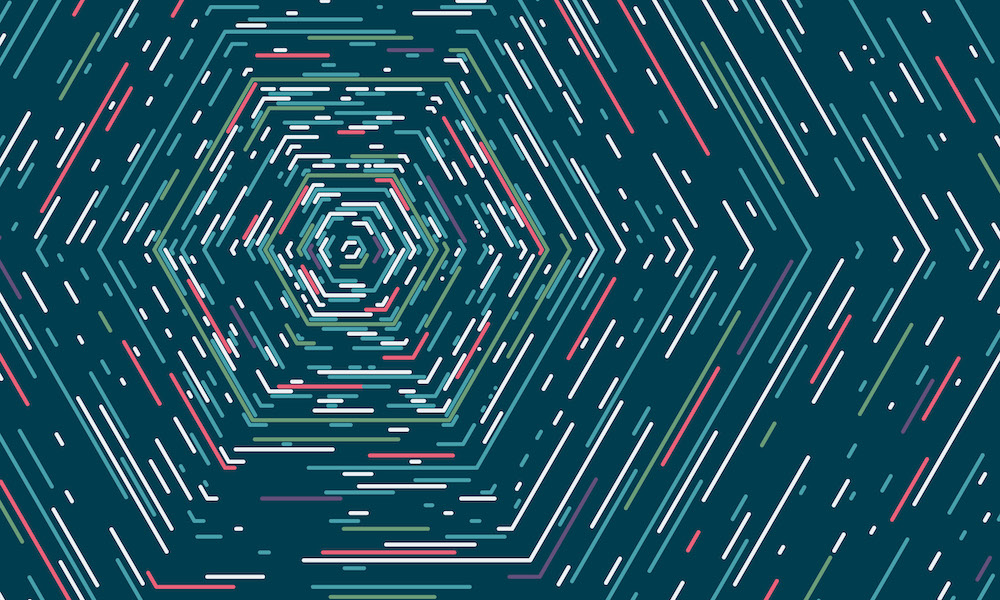
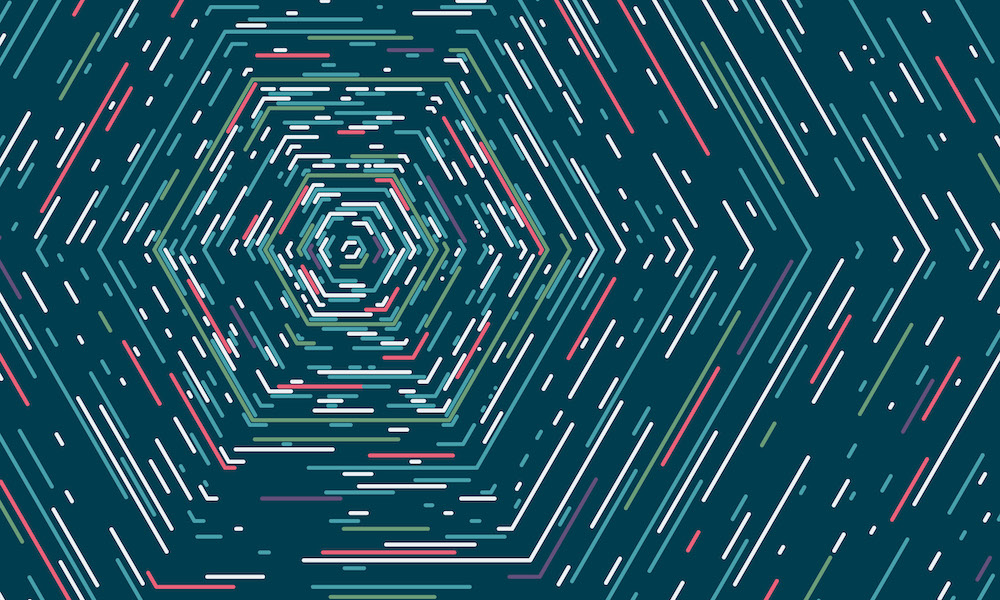
How do LLMs really work?
Simply put, LLMs are algorithms that look at strings of text available on the internet and create a correlation map of ‘what comes after what.’ For instance, an LLM would put the word ‘jumps’ after the words ‘big brown fox’ because it happens in 90% of the text it is trained on. And, believe me, the LLM has seen a lot (of the order of trillions of words)— making them massive models requiring just the model storage space of about 800 GB for GPT3.
Use of generative AI leading to concerns over human psychology
The current era is characterized by instant gratification, decreasing focus and reduced attention to detail. This setting can cause unwarranted consequences if one is not conscious and intentional when ‘consuming/engaging with LLMs’ output.’
Because the results appear coherent and sophisticated and are presented with confidence, people assume it must be correct. I often find generative AI’s responses almost incomprehensible in the first read and they require multiple ‘goings-over’ to understand. Despite this experience, I end up giving the tech the benefit of the doubt. I think, “It must be right because it sounds right, even though I don’t fully understand it.” It’s not just the technology but human psychology at play here too.
The latter worries me and my colleagues. Humans are not adept at reading manuals or instructions. Often, they first interact with the system and then form an opinion based on the interaction. With LLMs, this can be quite deceptive. It is no surprise that there is an explosion of content around Artificial General Intelligence (AGIs) and AI taking over the world.
Tracing the evolution of generative AI’s accuracy
Since its launch, the prevalent ChatGPT has significantly evolved. Now, it has guardrails (for instance, the tool will not answer questions or give responses that can harm a person, like how to commit suicide) and OpenAI is actively using people's feedback to update the models. ChatGPT’s responses to questions have also changed over the last couple of months. For instance, just after its release, the chatbot agreed with me when I ‘convinced it’ that 2+2 is 5. However, today, it respectfully disagrees with me and sticks to the answer. Arithmetics, with its certainty of solutions, is perhaps easier to fix. A similar approach might not always work.
I believe, to address these limitations, the research community should consider Symbolic AI, popular in the 60s and up to the 90s. Symbolic AI approaches models in the way the human brain learns, supported by human-readable representations of problems, logic and search.
Until accuracy and factual responses become par for the course, you must tread carefully in generative AI waters. Here are a few recommended cautious applications of generative AI.
How to use and not to use generative AI
Generative AI is great for creative tasks because — it is known to ‘create.’ In fashion or interior design, image-generation tools can help inspire innovation. While writing a mystery novel, ChatGPT can help with an unexpected twist.
You might even find a great use for the chatbot in composing the perfect email (however, if you are not using the enterprise version, your data is used to improve the model, i.e., your data could be exposed). LLMs are also good at recognizing patterns and making associations. So language translation and text summarization are good use cases.
We would not recommend using it for research because you may get information that is partially or completely made up - hallucination is the popular term used for this behavior. Also, avoid using LLMs for analytical work because they can’t ‘reason.’ Its task is to generate a string of words (which it will based on the data it has ‘seen,’ i.e., been trained with). Most of the LLMs have been trained on the content found on the internet. Hence, there are inherent biases and inaccurate information that could be reflected in the model’s responses. Users are expected to recognize the model's limitations and use their judgment. The responsibility of how the information generated is used lies with the user.
I believe one can compare LLMs to Google as Google might be compared to a library. I have found it most helpful to use generative AI as a ‘pre-google’ for my research purposes. I use it to get pointers which I then Google to find concrete and reliable information on the topic. About 80% of its response content checks out. The rest I attribute to hallucinations. I also use it to summarize information and help me with succinct titles and subject lines.
The best way to think of generative AI models is as a ‘hive mind.’ The tech’s responses are the average/aggregate of the content published on the internet by the population. I would go as far as to equate what's on the internet to the population's thinking, in this context. If the majority of the population thought the earth was flat, that’s what generative AI’s response would be.
Disclaimer: The statements and opinions expressed in this article are those of the author(s) and do not necessarily reflect the positions of Thoughtworks.