Technology Radar Vol 32
The information in our interactive Radar is not available in your preferred language.
Thoughtworks Technology Radar is a twice-yearly snapshot of tools, techniques, platforms, languages and frameworks. This knowledge-sharing tool is based on our global teams’ experience and highlights things you may want to explore on your projects.
Each insight we share is represented by a blip. Blips may be new to the latest Radar volume, or they can move rings as our recommendation has changed.
The rings are:
Adopt. Blips that we think you should seriously consider using.
Trial. Things we think are ready for use, but not as completely proven as those in the Adopt ring.
Assess. Things to look at closely, but not necessarily trial yet — unless you think they would be a particularly good fit for you.
Hold. Proceed with caution.
Explore the interactive version by quadrant, or download the PDF to read the Radar in full. If you want to learn more about the Radar, how to use it or how it’s built, check out the FAQ.
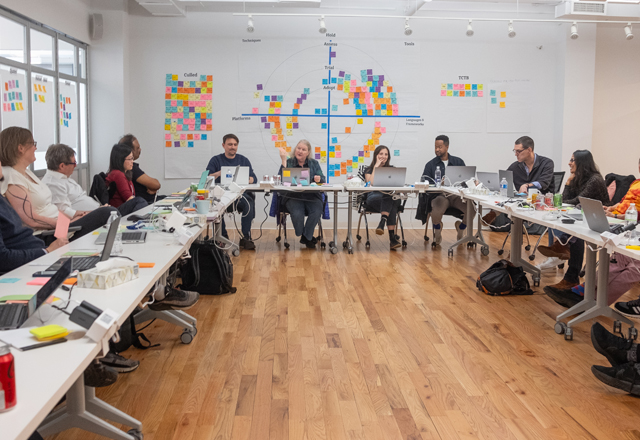
Inside the Technology Radar is a short documentary that provides a fresh insight into all things Technology Radar.

Themes for this volume
For each volume of the Technology Radar, we look for patterns emerging in the blips that we discuss. Those patterns form the basis of our themes.
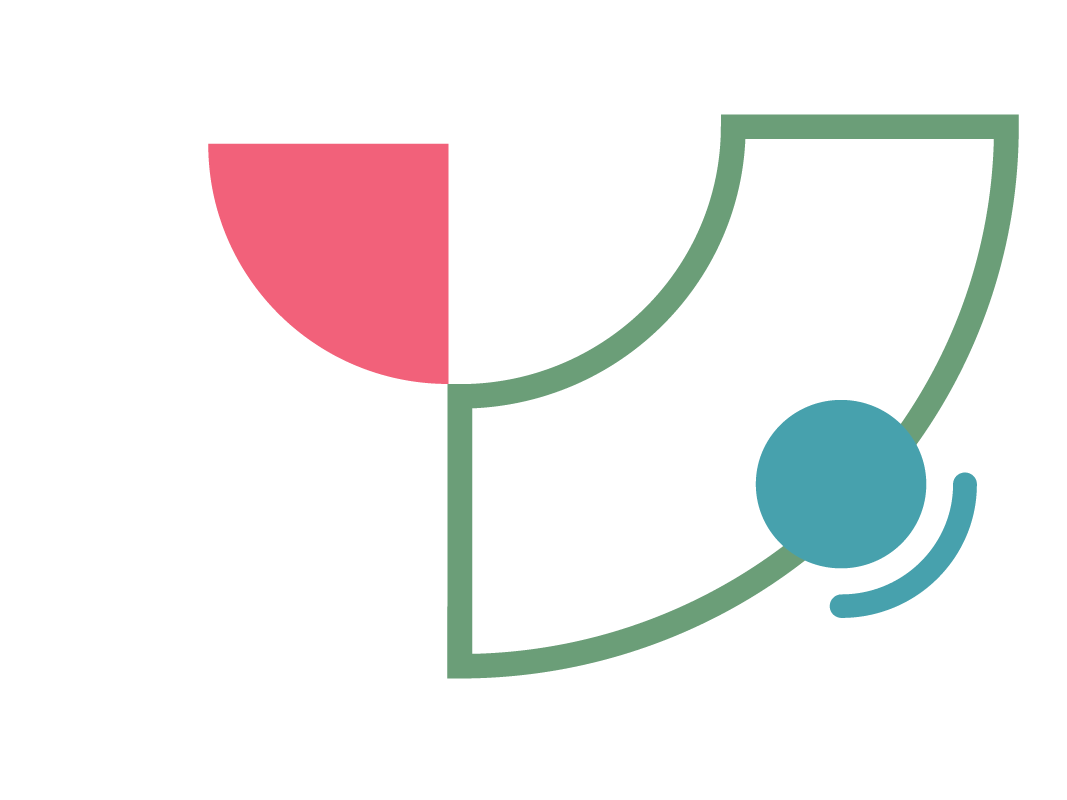
Supervised agents in coding assistants
Two of our themes highlight the rapid innovation in generative AI, and one of them is about the accelerating capabilities of coding assistants. More and more of these tools allow developers to drive implementation directly from an AI chat within their IDE — a mode also called "agentic", "prompt-to-code" or "chat-oriented programming (CHOP)." In this approach, AI assistants go beyond answering questions or generating small snippets; they navigate and modify code, update tests, execute commands and, in some cases, proactively fix linting and compilation errors. While we remain skeptical of coding agents that promise fully autonomous development of large tasks, we’ve seen promising results with this supervised approach, where developers still guide and oversee the agent's actions. Cursor, Cline and Windsurf are leading this trend in the IDE-integrated tools space, with GitHub Copilot also advancing. Agentic assistants such as aider, goose and Claude Code are terminal-based alternatives. Despite these advancements, we remain cautious about how this increases complacency with AI-generated code, as in spite of some very good results, we still see a lot of need for steering and vigilance in the code review. With great power…
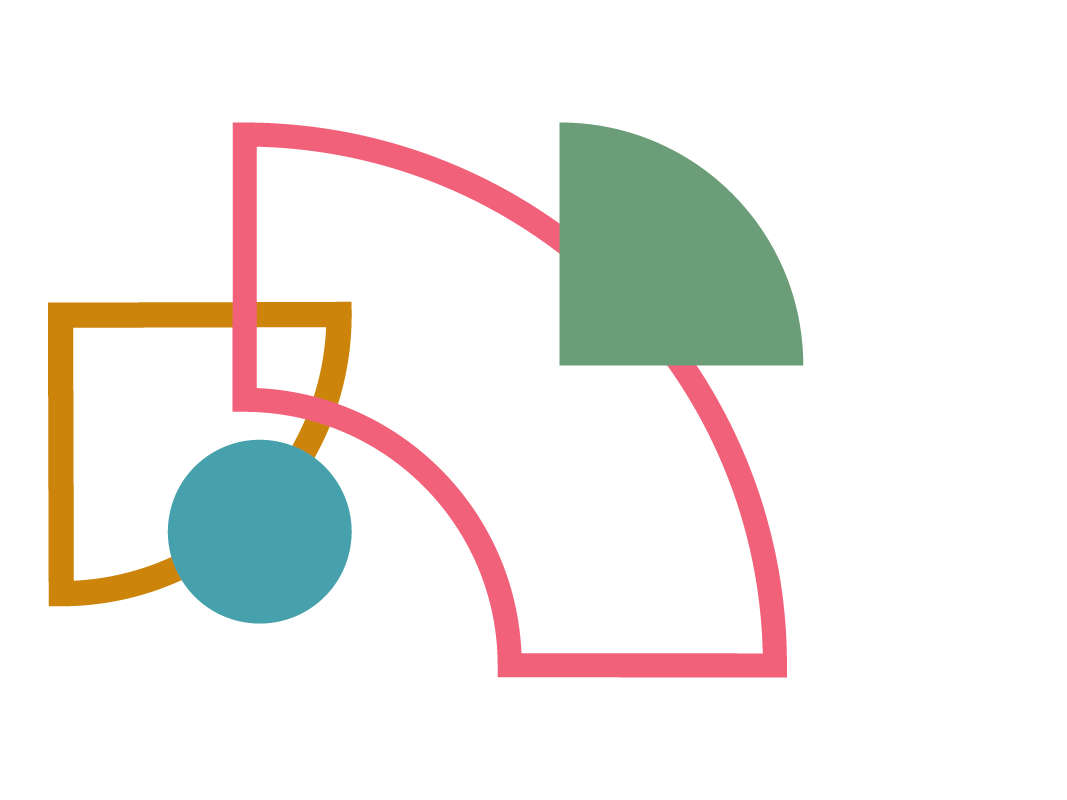
Evolving observability
We’ve seen significant movement in the observability space, driven by the growing complexity of distributed architectures. While observability has long been essential, it continues to evolve alongside the rest of the software development ecosystem. One emerging focus is LLM observability, a critical piece in operationalizing AI. We’ve seen a surge in tools for monitoring and evaluating LLM performance, including Weights & Biases Weave, Arize Phoenix, Helicone and HumanLoop. Another trend is the integration of AI-assisted observability, where tools leverage AI to enhance analysis and insights. Additionally, the increasing adoption of OpenTelemetry is fostering a more standardized observability landscape, enabling teams to remain vendor-agnostic and more flexible in their tooling choices. Many leading observability tools — such as Alloy, Tempo and Loki — now support OpenTelemetry. The rapid innovation in observability tools demonstrates growing industry awareness of observability’s importance, creating a cycle where evolving practices and technologies reinforce each other.
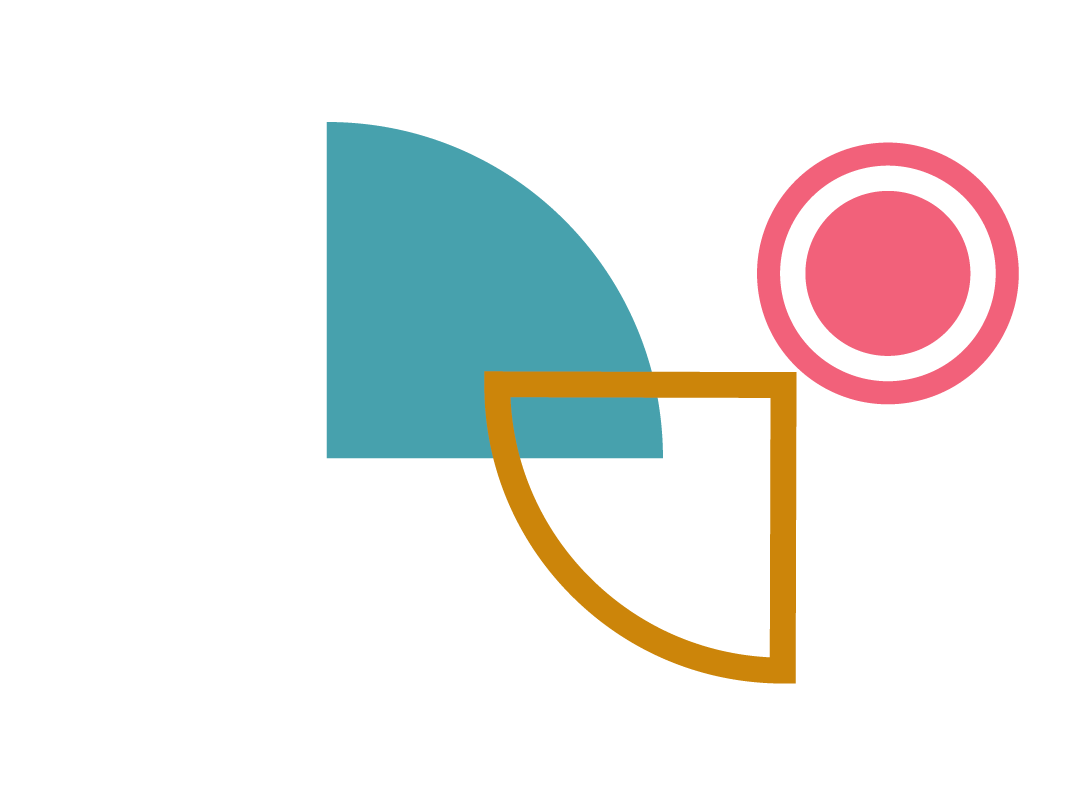
R in RAG
We expect different aspects of the generative AI ecosystem to evolve at varying rates, and in this edition of the Radar, we see this happening for the R in RAG (retrieval-augmented generation). One of the key interactions with the LLM black box is customizing the prompt’s inputs to generate relevant and useful responses. The growing need for effective retrieval in RAG has led to the emergence of new tools and techniques featured in this edition. For example, we discussed corrective RAG, which dynamically adjusts responses based on feedback or heuristics; Fusion-RAG, which combines multiple sources and retrieval strategies for more comprehensive and robust responses; and Self-RAG, which avoids the retrieval step altogether, fetching data on demand. We also highlighted FastGraphRAG, which aids understandability by creating human-navigable graphs. Based on our team's discussions and nominations, the R in RAG is a hot topic and evolving rapidly.
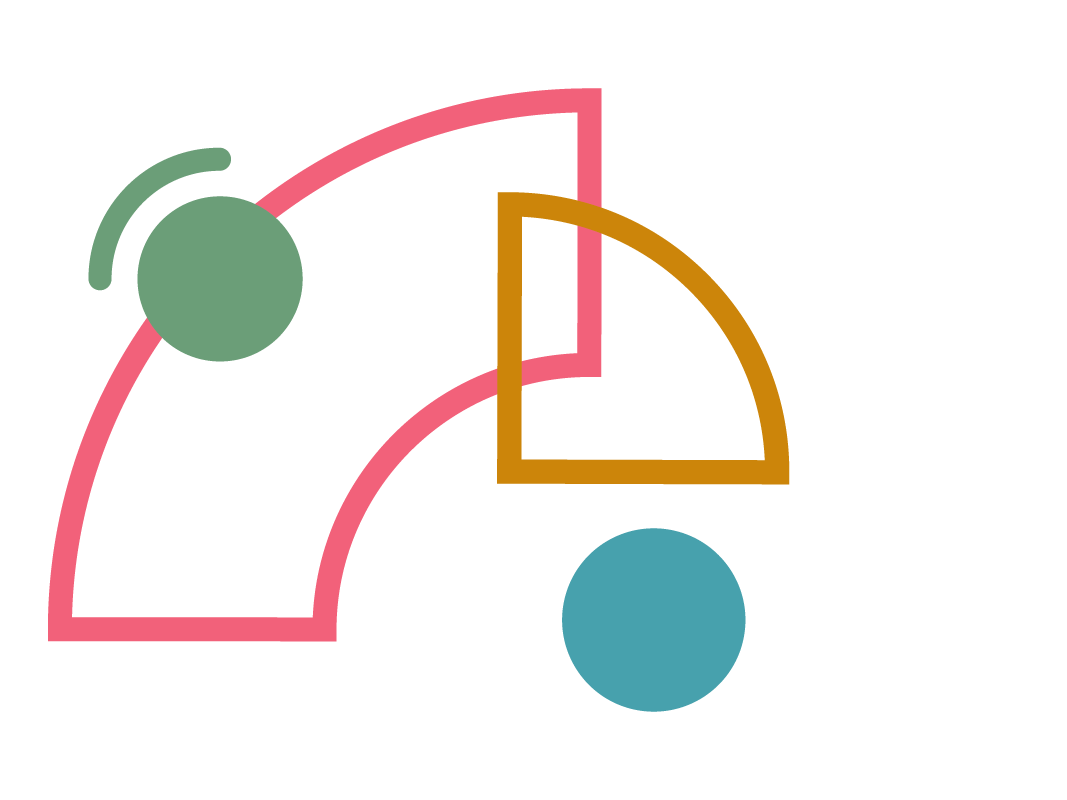
Taming the data frontier
Big data has long been a key concern for the industry, but conversations around this edition of the Radar centered not so much on size but more specifically on handling rich and complex data. With the increasing presence — and importance — of unstructured data in the enterprise, ensuring data is effectively managed and packaged so it can be successfully leveraged for everything from AI applications to customer analytics is today vital for businesses.
This is reflected in many blips: from vector database tools to analytics products like Metabase, it’s astonishing how much of the software ecosystem is being driven by what we want and need to do with data. It’s not just about tooling though — in this edition we noted data product thinking, a framework that encourages teams to apply the principles of product thinking to the analytic parts of their ecosystem. The emergence of data product thinking is, to some extent, a result of the ongoing challenge of properly leveraging data (something that’s been talked about for years, well before the rise of AI) — the fact that it has found its way into the spotlight (and our Radar conversations) demonstrates that the need for data discipline is now as great as it has ever been. Without it, organizations may struggle to innovate and could well be at a commercial disadvantage in the medium and long term.
Featured Insights
Contributors
The Technology Radar is prepared by the Thoughtworks Technology Advisory Board, comprised of:
Rachel Laycock (CTO) • Martin Fowler (Chief Scientist) • Bharani Subramaniam • Birgitta Böckeler • Bryan Oliver • Camilla Falconi Crispim • Chris Chakrit Riddhagni • Effy Elden • Erik Doernenburg • James Lewis • Ken Mugrage • Maya Ormaza • Mike Mason • Neal Ford • Ni Wang • Nimisha Asthagiri • Pawan Shah • Selvakumar Natesan • Shangqi Liu • Vanya Seth • Will Amaral
Subscribe. Stay informed.
We publish articles related to Technology Radar throughout the year