Financial services newsletter
By Nalini Haridas, principal consultant, Thoughtworks
"We cannot solve our problems with the same thinking we used when we created them."
The rise of RegTech around the financial crisis of 2008 was a promise to transform compliance into a competitive advantage. The awaited transformation, however, of risk and compliance in the capital markets has been slower than first anticipated and adoption has been inconsistent. A big driver of the transformation of risk and compliance has been to improve the ROI of compliance. Transformations, however, must do a lot more than that and should be transformational for the individuals working in risk and compliance.
The humans of risk and compliance are compliance officers, controllers, risk officers and financial officers of firms. Regulatory analysts and risk modellers at regulatory bodies are individuals who monitor and control vast amounts of data and processes. They do their work with varied levels of automation, battling the time sensitivities of reporting and braving ever-increasing regulatory requirements. These individuals, who can face personal and legal consequences for errors, make up the departments that safeguard the reputation of firms. A RegTech transformation with the best of technology should not only improve the cost efficiency of compliance, but help organizations rethink their current processes with recognition of the legal obligations of risk and compliance departments.
In this article, we’ll explore three emerging tech stories in risk and compliance, curated across key areas of focus in capital markets: trade surveillance, regulatory reporting and market modelling. With these, we’ll discuss how emerging technologies can support, augment and empower risk and compliance departments in the capital markets. These stories exemplify solving existing problems with new thinking, showcase innovation mindsets and hint at the preparations needed to transform.
Emerging tech story one: Human-in-the-loop and more AI, for trade surveillance
Trade surveillance is extremely complex due to the dual need to monitor trading activity and communication data. Monitoring trades, orders, instructions to trade and communications for a range of asset classes, product types and markets is time-consuming, error-prone and expensive. Communications data retrieved from phone calls, text messages, emails and more is varied, unstructured and hard to analyse in-flight due to the volume and velocity of data. Analysing communications data in parallel to trading data, and combining the two successfully, helps in establishing the motive behind trading activity. Requirements in regulations, such as the Market Abuse Regulation (MAR), are looking at reporting this kind of a semantic understanding of trading activity. In addition to the data complexity, financial institutions are also struggling with volumes; the number of asset classes and markets that the firms operate in are increasing.
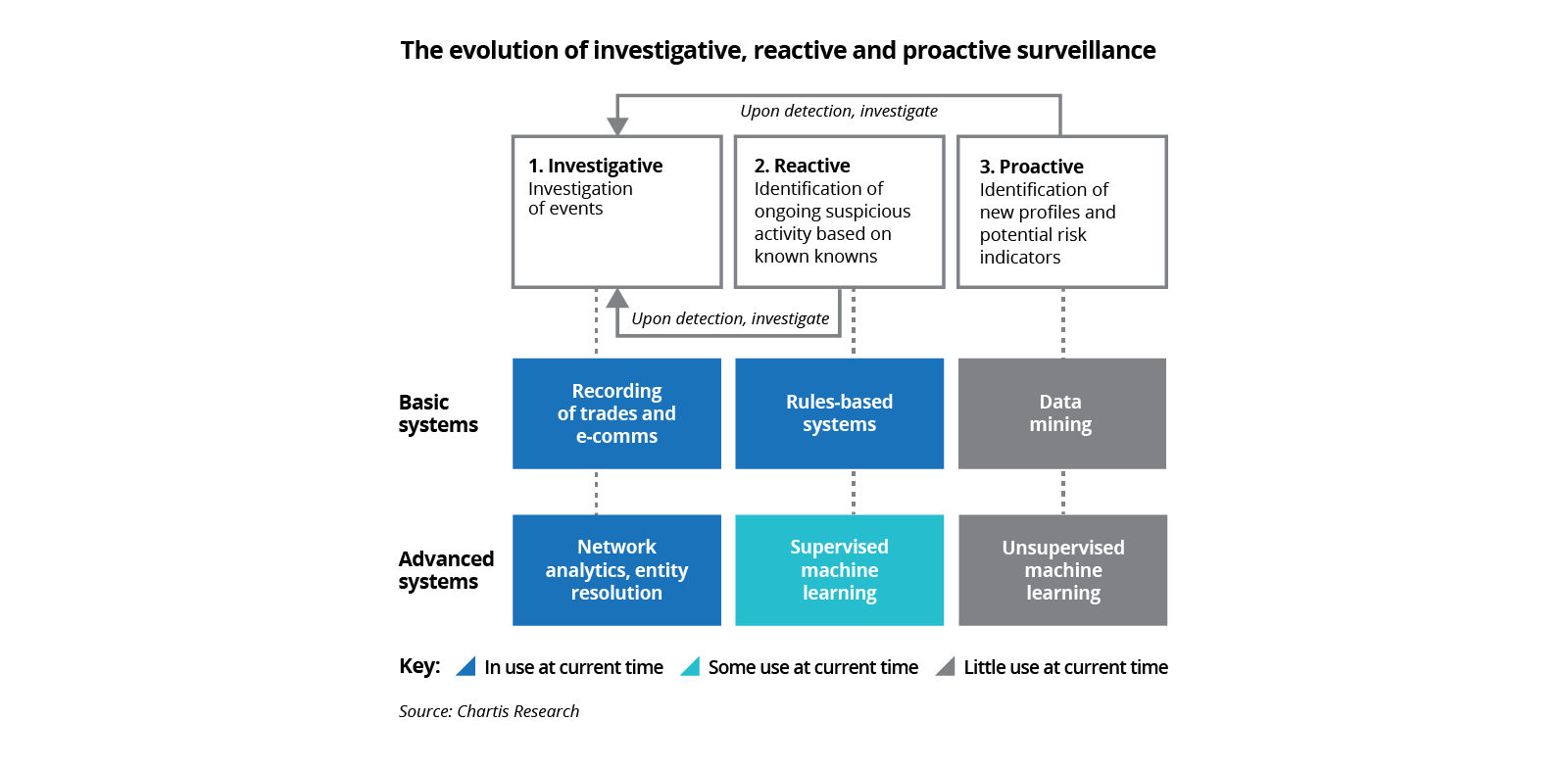
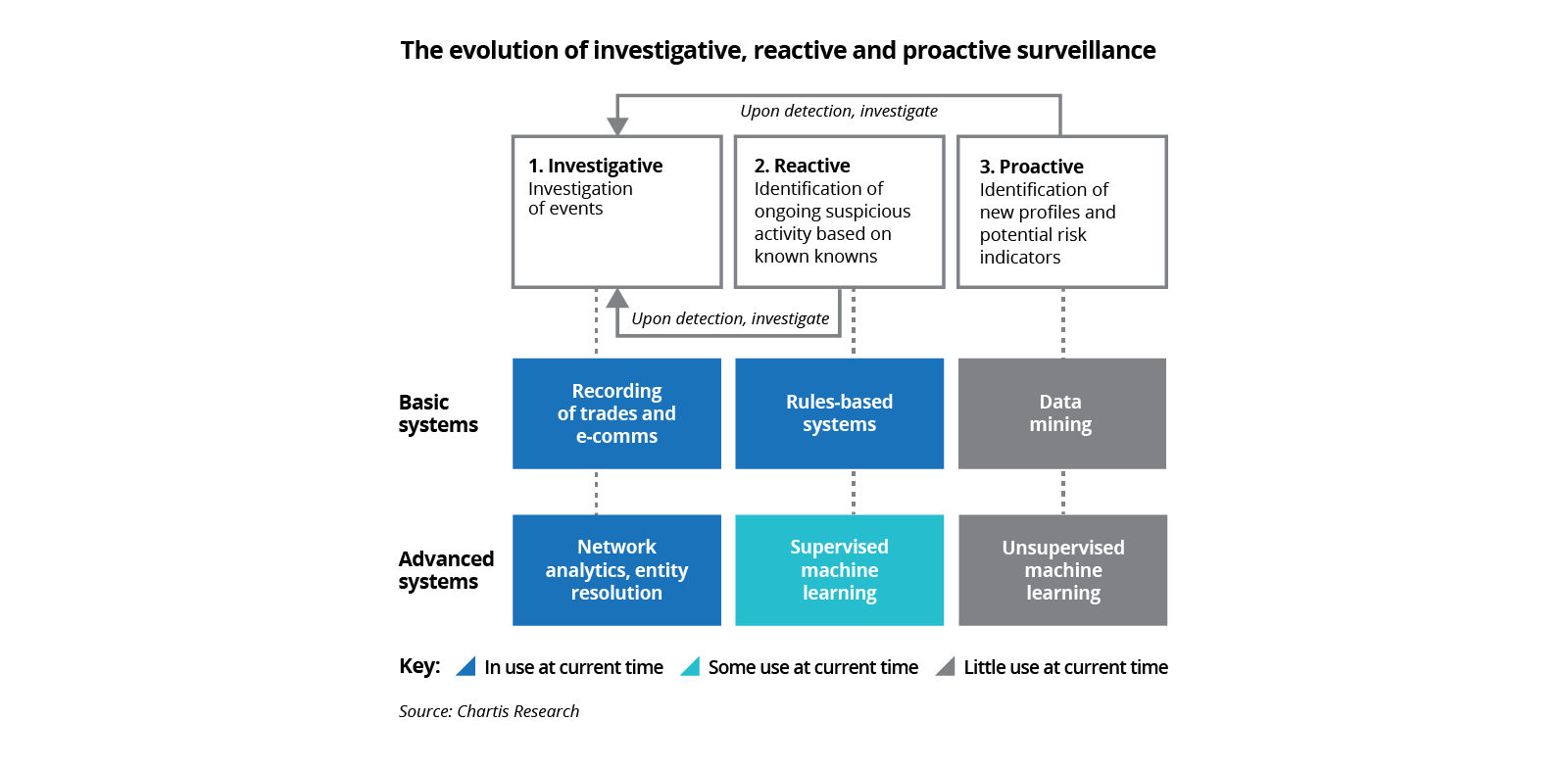
For modern trade surveillance, therefore, there is an enormous data processing problem to solve. Current systems used for trade monitoring are rule-based and throw up alerts for known patterns of behaviour. Supplementing this with AI/ML models is now a widely taken approach for trade monitoring, as recognised in various surveys.
The advantage of machine learning is that it is adaptive and will throw up alerts which are not rule-based and pre-known. An additional benefit is that adaptive learning also significantly reduces false positives, reducing the time and costs for further investigations. However, the adoption of artificial intelligence for trade monitoring has been held back by explainability issues, which make it difficult to foster trust. The need, therefore, is to bring in a process that includes human input at various stages of the monitoring process, and merges technology output with human insight from experts in the risk departments, in a seamless manner.
An exemplar story is that of the Nasdaq project, codenamed ‘Chiron’, launched to incorporate advanced AI techniques such as Human-In-The-Loop for market surveillance. It's an approach that is used to incorporate human input into AI models. By this, employees were able to apply critical thinking, alongside Artificial Intelligence, not only to provide inputs to train the machine learning models but also to validate the output, and add human judgement and experience to what the machine produced. Nasdaq developed its project as a three-party collaboration between the departments - Market Technology, Machine Intelligence Lab and Market Surveillance to successfully bring in human input and validation to its use of AI models.
Our recommendation: For a successful introduction of advanced AI models, it is important to set up cross-functional and agile teams. Additionally, we suggest using a technique like CD4ML to create an iterative process for model deployment and incorporation of human input. This will establish the required feedback loops and aid in successfully incorporating advanced AI.
Emerging tech story two: ‘Straight-through processing’ blockchain for regulatory reporting
The cross-industry body, TISA (The Industry and Saving Alliance), has recently allocated a blockchain contract to a major technology company to build a MiFID II regulatory solution for asset managers and advisors. The new platform, called TURN (TISA Universal Reporting Network), will allow capturing and sharing of MiFID II data using a standardised template, across Europe. There are multiple other examples of the exploration of regulatory reporting using blockchain by capital markets, banking regulators and financial institutions globally. Initiatives such as these bring the concept of straight-through processing (STP) to regulatory reporting and have the potential to massively reduce the cost of compliance.
A noteworthy example in this area is Project Maison, rolled out by the Bank of England and the FCA, in collaboration with a few leading banks. It is a proof-of-concept for regulatory reporting of mortgages using blockchain. The learnings shared by Project Maison are worthy of study and are applicable for capital markets regulatory reporting.
Blockchain, as a technology, enables a distributed ledger and creates a single shared view for all the participating stakeholders. Project Maison created banks and the regulator as nodes on the blockchain. The regulator’s node, in such a permission-based blockchain system, had access to all the data, giving real-time information to the regulator on balances and transactions. On the other hand, the bank’s nodes on the blockchain did not have access to other bank’s data. The project demonstrated that it is possible to move to a near real-time and transparent system of regulatory reporting with data controls and protection. It also indicates that embedded supervision can be a reality.
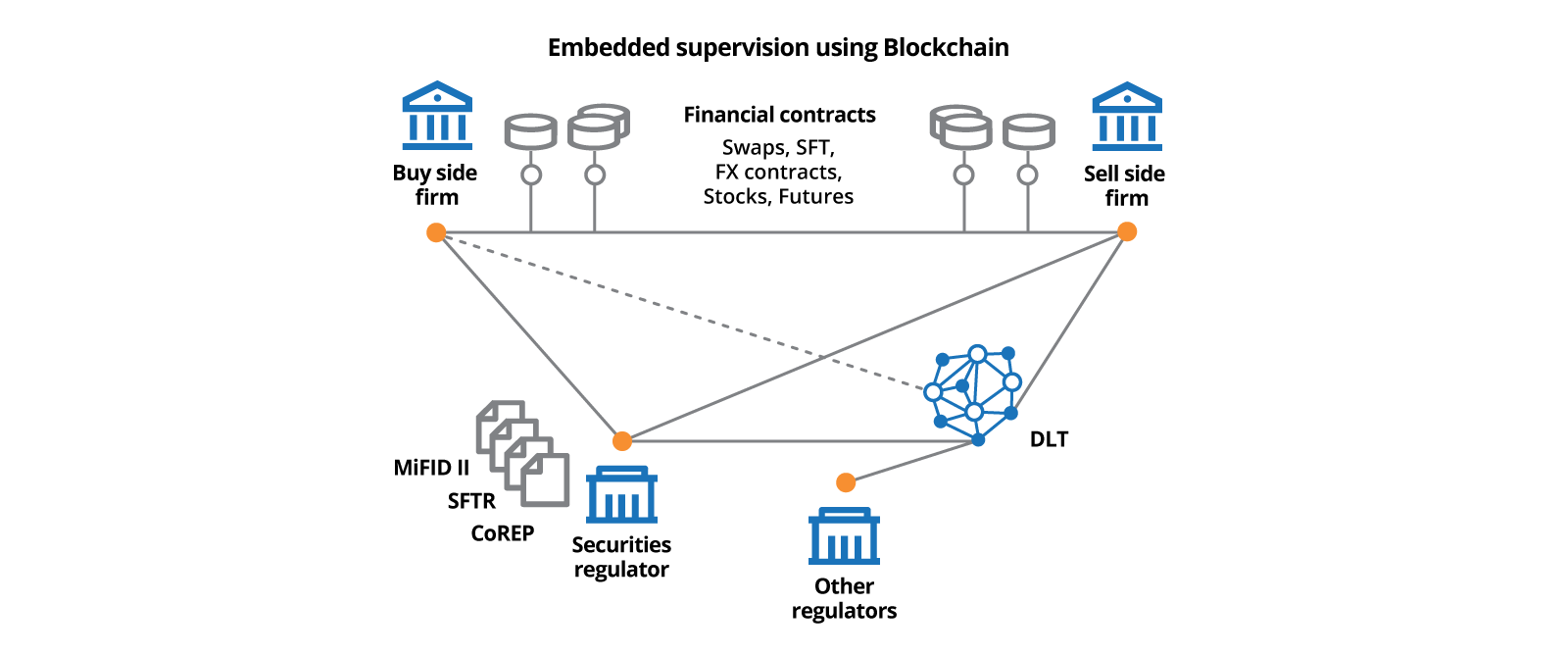
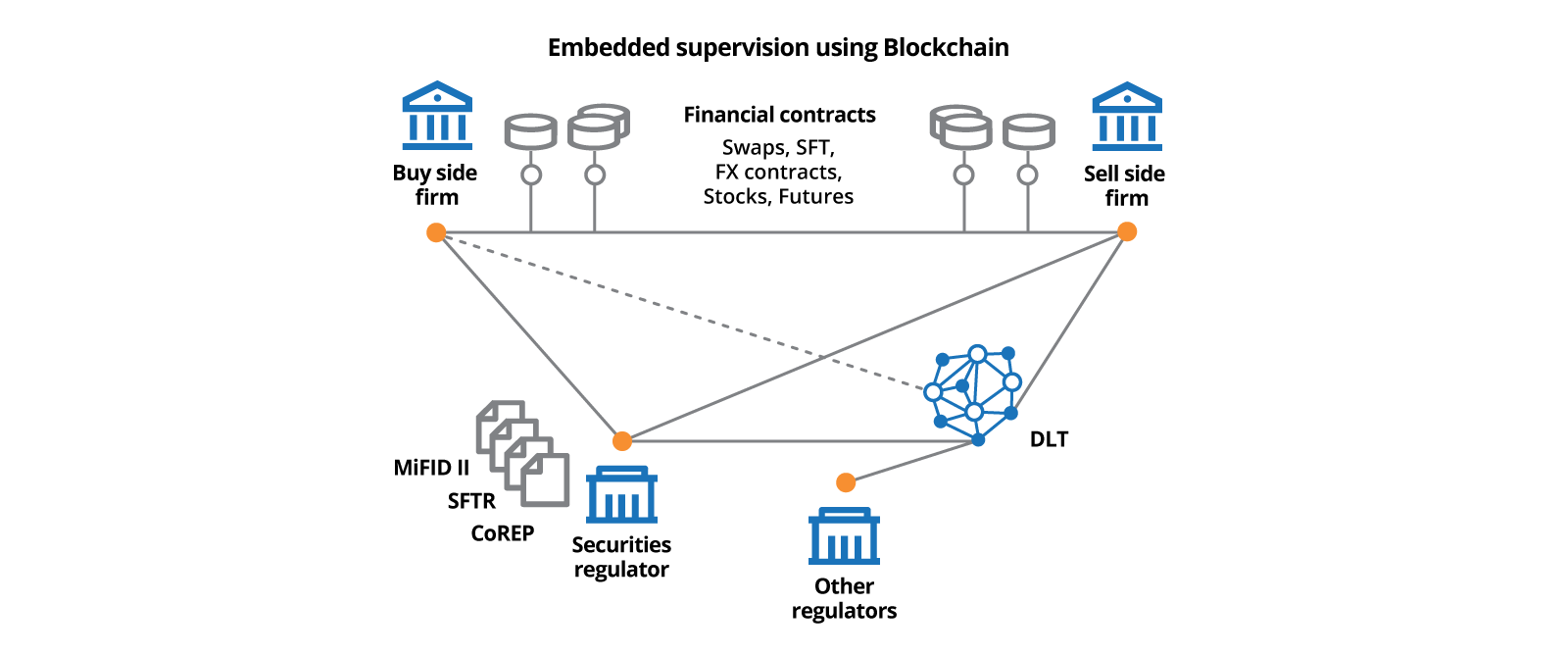
As described in the case study on Project Maison, three major benefits were identified by implementing regulatory reporting using blockchain: enhanced data quality and standardized reporting formats; greater transparency and accountability across product or trade lifecycles; consistent application of rules across all stakeholders of a balance or a transaction. These benefits solve the current problems in regulatory reporting: the need for integrating and standardizing all of an institution’s data, yet having visibility of its lineage. Additionally, reporting close to the source reduces the cost of storing and processing data.
However, the pilot also showed an important fall-out of the use of Blockchain-based process streamlining. It could reduce the ability to do internal checks and controls before reporting to the regulators and remove the opportunity to do internal corrections. Some of the loss of control was related to process shifts, such as loss of control on aggregations and the trade-off between standardization versus discretion in reporting decisions. Although process shifts evolve in a phased manner, it is noteworthy that there is an ongoing regulatory reporting shift from a template-driven to a data-driven approach. To prepare for such a transition, technology can lead by implementing data controls closer to the source.
Our recommendation: To successfully implement blockchain as a solution for regulatory reporting, companies need to build in supporting data controls at the department or desk level.This would mean making additional technology and ownership choices which will enhance the quality and visibility of data at its source. The Data Mesh describes an approach to decentralize data platforms and data ownership, and set up distributed governance of data. A federated data governance architecture will give operational and risk managers quality controls and visibility of data ahead in the chain.
Emerging tech story three: Risk modeling as the emergent effect of micro behavioral interactions
Risk modeling and scenario projections are crucial tools available to risk officers, to model losses and portfolio performances for changing environments and future events. Traditional ways to model scenarios have been to apply parameters that mimic macroeconomic conditions on balances, positions, and volumes. These methods do not account for the ripple effects of market events, or of investor behavioral patterns which impact performance and losses in a non-linear fashion.
Agent-based modeling (ABM) is a technique used for modeling complex systems and gaining a deeper understanding of the system’s behaviors. In this technique, a system is modeled as a collection of autonomous decision-making entities called agents. It helps to uncover the emergent impact on a system caused by the interaction of simulated heterogeneous agents and is good for scenarios where the past is not necessarily a predictor of the future.
Last year, The Banker did a cover story called ‘The New Science of Risk Management’ which highlighted agent-based modeling as a simulation approach, and how a technique that has found a lot of traction in the natural sciences has application in financial services. Not surprisingly, this is an area that has been under research for a lot longer. The Office of Financial Research (part of the US Department of Treasury) published a working paper on an agent-based model, a few years back. The paper describes simulating the vulnerability of a financial system made up of hedge funds and broker-dealers and simulates transactions for a variety of asset classes. Models such as these mimic micro-interactions and human behavior under stress and the impact of these on systems.
New technologies and thought processes empower risk professionals to develop more realistic market models and prepare for disruptions based on a keener understanding of human behavior. Previously, developing simulations using agent-based models was restricted to the computational power available and the number of agents that could be spawned. With computational advances and the ability to process large amounts of simulated agents on the cloud, this bottom-up agent-based modeling technique can scale to a sizable number of agents and output emergent system behavior which is realistic.
Our recommendation: Cloud computing firms are seeing the potential of techniques such as agent-based modeling and collaborating with simulation companies to build this capability. At Thoughtworks, we have developed an open-source framework for building agent-based simulation models for epidemiology using commodity hardware. Our learning is that developing IT infrastructure to process big data on the cloud using commodity hardware, is required for incorporating new risk sciences and new simulation techniques.
Conclusion
Patterns observed from these emerging technology stories can be used to create an approach towards a proactive risk strategy in the capital markets. Some of our suggestions are:
- Build up the needed infrastructure, including computing on the cloud, and help risk departments explore new ideas to understand risk. Doing so can solve processing challenges for new risk modeling methods that mimic human behavior and require large amounts of data.
- Rethink data pipelines and how to serve data from its source. Distributed data pipelines that feed data directly to external entities including regulators are the future and federated data governance which empowers internal stakeholders and gives them early visibility of data issues is possible with the right tooling.
- Use agile thinking and streamlined AI model deployment approaches to incorporate AI technologies like human-in-the-loop.
"It takes less time to do a thing right, than it does to explain why you did it wrong."
Emerging technologies offer the potential to drive efficiencies in risk and compliance through smart use of AI/ML, data-mesh, and blockchain-based techniques; ultimately improving operational excellence, cutting-down costs, as well as empowering the humans of risk and compliance with the help of technology. It’s an opportunity to approach risk and compliance the right way, proactively, rather than treating these concerns as a post facto-activity. It’s an investment that will empower teams and leaders to make the right decisions, at the right times, supported by technology.
If you'd like to discuss your risk management challenges with us, please don’t hesitate to get in touch at contact-uk@thoughtworks.com.
Want to receive our latest banking and finance insights ?
Sign up for the financial services series now. Delivering a fresh take on digital transformation, emerging technology and innovative industry trends for financial services leaders.