From autonomous vehicles to personalized medicine, from intelligent chatbots to predictive maintenance, AI has become the cornerstone of modern innovation. Yet, as with any transformative wave, not all initiatives succeed. Despite widespread AI adoption, 74% of companies struggled to achieve and scale value from their AI initiatives in 2024, highlighting the gap between AI hype and real-world impact. These failures are not just technical — they're rooted in flawed approaches to strategy, execution and culture.
To highlight these pitfalls, let’s explore the seven deadly sins of AI transformation, a companion piece to seven deadly sins of digital transformation. These missteps reveal why some organizations stumble in their AI journeys and what leaders can do to course-correct.
1. The sin of gluttony: Overindulging to instantly transform and hoarding data without purpose
"People who acquire things beyond their usefulness not only will derive little or no marginal gains from these acquisitions, but they also will experience negative consequences, as with any form of gluttony”. - Ray Dalio
In the race to harness AI’s potential, many organizations fall victim to the idea that “data is the new oil”, triggering a race to amass large quantities of data. However, more data doesn't automatically mean better results. This unchecked over-investment and indiscriminate data hoarding, often results in cluttered systems, ballooning costs and untapped potential. A staggering two thirds of enterprise data remains unused, turning a potential transformative asset into a liability.
This "gluttony" for data and technology can sabotage AI outcomes. Poor data governance, irrelevant datasets, and low-quality information clog pipelines can lead to flawed insights and may prove a disappointing return on investment.
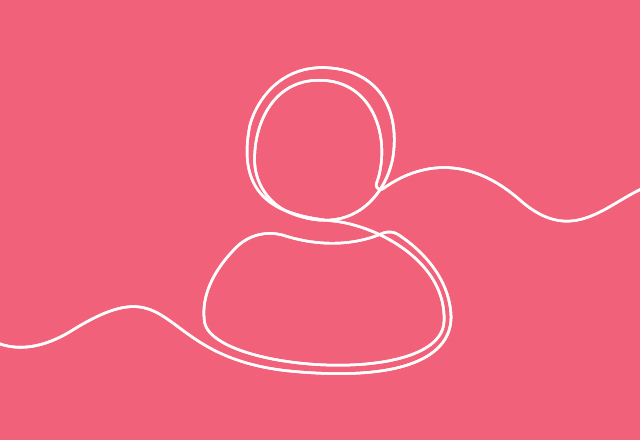
The importance of expert validation
Over $5 billion was invested by IBM Watson for Oncology in acquisitions to build the platform, but a lack of clinical validation and real-world impact led to limited adoption and significant financial losses.
This 'gluttony' for data and technology overshadowed the need for rigorous testing and meaningful outcomes, ultimately leading to limited adoption and a significant financial loss for IBM.
Redemption tip:
Focus on the data you need — not the data you can collect. Adopt a value-driven data mesh approach, treating data as a product and owned by specific business domains. Each data product should be designed to deliver measurable value, such as improving customer segmentation, optimizing supply chains or enhancing predictive maintenance. By decentralizing data ownership and ensuring high-quality, domain-specific data products, organizations can avoid the pitfalls of data hoarding and unlock actionable insights.
Self-learning AI will only ever be as good as the data it learns from, so consistently high standards of data quality and governance are vital. This is critical to avoiding AI hallucinations that can be caused by inaccurate training data, and incorrect assumptions.
Self-serve platforms and product thinking enable faster and more sustainable AI adoption compared to centralised teams.
2. The sin of lust: A narrow focus on AI success at all costs
"The most important thing to do if you find yourself in a hole is to stop digging." ― Warren Buffett
Organizations, in their quest for exponential growth, faster time to market, and a competitive advantage, relentlessly pursue cutting-edge technologies without fully understanding their applicability or potential drawbacks. This is often at the expense of ethical considerations, long-term sustainability and sound business judgment leading to a "keeping up with the Joneses" culture where decisions are driven by FOMO (fear of missing out) or theater, rather than strategic goals or customer-centric needs.
The “organizational theatre” often involves deploying flashy AI initiatives to signal innovation only to develop "cold feet" when these solutions face real-world production challenges. This rushed and poorly planned adoption can result in unrealized value, ethical missteps and a loss of customer trust.
Ultimately, unchecked pursuit of AI can have serious unintended consequences, ranging from data breaches to reputational damages.
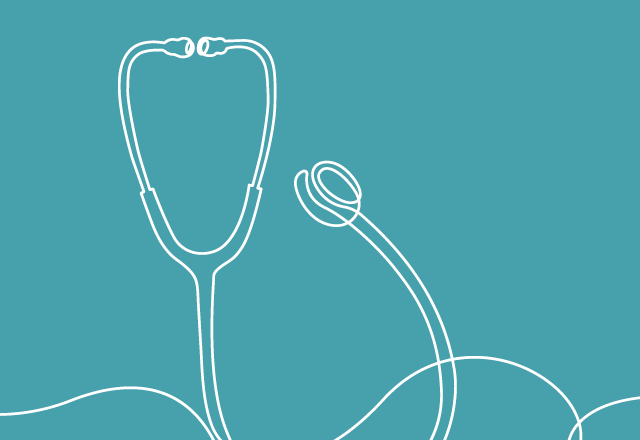
While there has been much fanfare over AI’s ability to aid in medical imaging, some researchers are worried about over-reliance on AI-based image reconstruction techniques to make diagnoses. They warn that these techniques can result in major errors in the final images, potentially harming patients.
Redemption tip:
Define clear use cases: Identify specific business problems where AI can deliver measurable value, such as customer segmentation, supply chain optimization or fraud detection. For organizations aiming to become adept in leveraging AI, the true measure of success lies not merely in automating routine tasks, but in enhancing human capabilities and magnifying the impact of individual contributions within the organization.
Augment, don’t replace: Use AI to complement human expertise, not replace it. For example, in healthcare, AI can assist doctors in diagnosing diseases faster, but final decisions should remain with medical professionals. Embrace augmented AI approaches and focus on complementing human expertise, not replacing it.
Align with strategic goals: Rapid pace of AI development might make it time to revise your AI strategy. Ensure AI initiatives align with long-term business objectives, such as improving customer satisfaction, reducing costs, or driving innovation.
Focus on culture and governance: Cultural transformation and governance frameworks matter as much as technical excellence.
3. The sin of pride: Overestimating competitive edge and ignoring market realities
“Success is a lousy teacher. It seduces smart people into thinking they can’t lose.” ― Bill Gates
Overconfidence can blind enterprises, leading them to overestimate their abilities and underestimate the emerging threats. This misplaced confidence often stems from past successes, causing organizations to ignore rising competitors or overlook critical industry trends. A reluctance to reassess their strategies or innovate further leads to stagnation, leaving them vulnerable to being outpaced.
No company has a monopoly on innovation. The macro environment constantly evolves, with new players finding innovative ways to identify customer needs or distribute products and services. For example, the rise of quick commerce platforms has challenged e-commerce giants like Amazon and Flipkart, forcing them to rethink their strategies. Market leaders often struggle to accept this reality, clinging to outdated models and over-relying on perceived technological superiority.
One of the key reasons for failure is a gap between strategy and execution. Many organizations invest heavily in AI models they believe are "bulletproof," only to face black swan moments when these models fail to account for unforeseen variables. This over-reliance on specific models has been a recurring theme in financial crises, where seemingly robust systems collapsed under unexpected pressures.
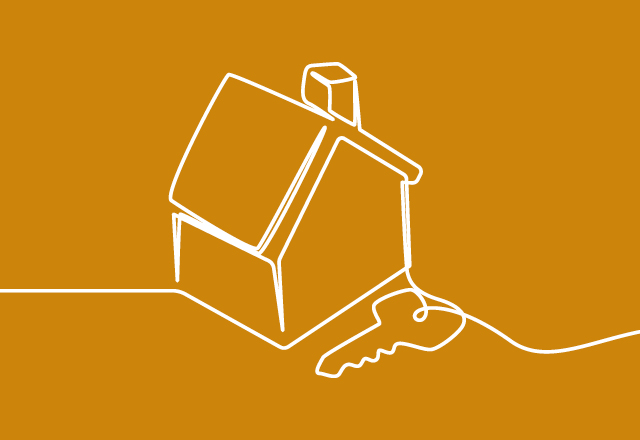
The risks of overconfidence
Most of us can appreciate the impact AI technologies can have. But it's important to remember they're still fallible. For instance Zillow aggressively expanded its home-flipping business, acquiring thousands of homes based its AI-powered valuation models.
But those models failed to account for market volatility, resulting in over $500 million in losses and the eventual shutdown of the program.
Redemption tip:
Cultivate humility: Acknowledge that no model is infallible and that competition will always find newer, better ways to serve customers.
Bridge the strategy-execution gap: Ensure that AI strategies are grounded in real-world execution, with continuous feedback loops to validate assumptions and adapt to changing conditions.
Embrace responsible tech for the AI era: Focus on ethical AI practices, transparency, and accountability throughout the AI lifecycle. Regularly audit models for biases and vulnerabilities, and be prepared to pivot when necessary.
Learn from failures and build resilience: Study past failures, such as financial crises or unsuccessful AI deployments, to understand the dangers of over-reliance on specific models and the importance of building resilient, adaptable systems.
4. The sin of envy: obsessing over competitors rather than customer value
“If you’re competitor-focused, you have to wait until there is a competitor doing something. Being customer-focused allows you to be more pioneering.” ― Jeff Bezos
In the race to outpace competitors, organizations often fall into the trap of mimicking rival AI solutions and engaging in price wars without aligning their efforts with customer needs. Driven by peer pressure and FOMO this approach diverts focus from true innovation, addressing customer problems, and delivering value. The desire to be seen as an innovator or early adopter — earning bragging rights — can lead to compromises in safety, ethics and long-term sustainability resulting in wasted investments in technology that doesn’t benefit end users. This ultimately hinders adaptability and leads to suboptimal solutions for diverse and complex real-world problems.
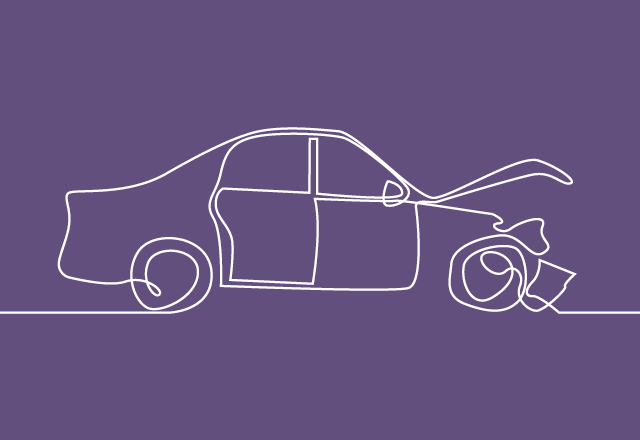
Cruise’s autonomous vehicles: In the race to compete with rivals like Waymo and Tesla, Cruise accelerated the deployment of its autonomous vehicles, but its robotaxis were involved in several road incidents before GM pulled the plug on its program.
Redemption tip:
Spending the time exploring a problem-space and getting to know local needs is essential for ensuring AI initiatives have a substantial impact. We can learn key lessons from the Jugalbandi project on how generative AI can drive transformative change.
Break down AI strategies: Tailor AI deployments to specific business contexts, such as operations optimization, strategic scenario planning or interactive R&D tools.
Resist peer pressure: Avoid rushing into AI adoption due to FOMO. Instead, focus on building robust, scalable solutions that deliver tangible value.
5. The sin of greed: Prioritizing dominance and control over ethics
“If you really look closely, most overnight successes took a long time.” ― Steve Jobs
The sin of greed manifests when organizations prioritize beating the competition over ethical considerations. This greed — whether for market share, cost savings or competitive advantage at any costs — often leads to the deployment of AI systems that propagate biases, violate privacy and erode trust. In the rush to dominate markets or cut costs, ethical considerations are sidelined, resulting in reputational damage, regulatory scrutiny and long-term harm to customer relationships. Organizations may prioritize beating competitors or capturing market share, leading to rushed AI deployments without proper ethical safeguards. The greed for reducing operational costs can result in the use of biased or low-quality data, compromising the fairness and accuracy of AI systems. The focus on immediate results often overshadows the need for transparency, accountability and long-term sustainability.
Consumer concerns about data privacy and misuse of AI, with 81% fearing their information will be used inappropriately and 63% concerned about generative AI compromising their personal data. This lack of trust hinders widespread AI adoption and demands a greater emphasis on transparency, accountability and users to have control over their data.
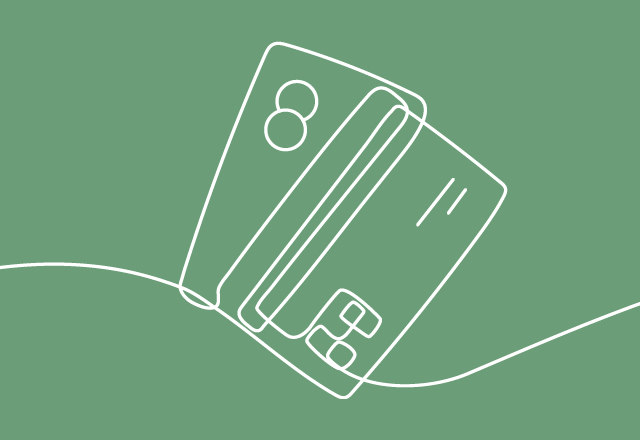
Apple’s AI-powered credit scoring system was supposed to make credit card applications easier. But it angered customers over perceived bias in the process, when the credit limits offered appeared to differ on the basis of gender..
Redemption tip:
Establishing ethical frameworks and guardrails is crucial. This includes addressing potential biases, ensuring transparency, and maintaining accountability throughout the AI lifecycle.
Engage diverse stakeholders: Collaborate with ethicists, regulators, and community representatives to ensure AI systems are fair, inclusive, and socially responsible.
Thoughtworks has developed the AI Design Alignment Analysis Framework, which consists of three lenses to analyze AI systems: technical function, communicated function and perceived function. This framework helps identify misalignment, audit existing systems and guide the development of new systems responsibly, ensuring meaningful transparency and social responsibility.
6. The sin of sloth: Being unresponsive to trends
“If you don't innovate fast, disrupt your industry, disrupt yourself, you'll be left behind.” ― John Chambers, former CEO, Cisco
The potential for AI-led growth and innovation is immense, however, there are a few organizations that may struggle to adapt to changing pace of technology evolution. This is due to a variety of factors, including an over-reliance on legacy technology, over-cautious approach to change and lock of vision of emerging technology trends.
While some competitors are advancing with AI-powered solutions, organizations that take a more measured approach can still find their footing and strategically position themselves for future success.
Despite a significant interest in AI, a majority of organizations (67%) are slow in its adoption. For these enterprises to succeed, a shift towards a culture of experimentation is essential, mitigating fears of failure and nurturing innovative thinking. Prioritizing responsible innovation, balancing compliance with creativity, and modernizing outdated systems will unlock transformative technologies for long-term success. This requires a strategic reassessment to identify and leverage emerging technologies and trends. But the value is clear: by 2026, organizations that operationalize AI transparency, trust and security will see their AI models achieve a 50% improvement in terms of adoption, business goals and user acceptance.
Redemption tip:
Adopt a data-first culture: Treat data as a strategic asset and prioritize its use in driving innovation and decision-making.
Operationalize AI: Thoughtworks advocates for operationalizing AI through managed services to realize long-term business value and ROI, emphasizing the importance of foundational capabilities and continuous enhancement of digital products.
Embrace managed services: Operationalize AI through managed services to build foundational capabilities and ensure continuous improvement.
Balance compliance and innovation: Use regulatory requirements as a framework for responsible innovation, not as an excuse for inaction.
Foster a culture of experimentation: Encourage calculated risk-taking and experimentation to stay ahead of emerging trends and technologies.
7. The sin of wrath: Playing the blame game
Treating AI as a universal tool without understanding the domain.
When AI initiatives falter, the temptation is to unleash wrath upon the technology itself, rather than confronting flawed implementation, biased data or inadequate oversight. This blame-shifting not only erodes trust in AI's potential but also stifles innovation by discouraging honest assessment and learning from mistakes. Instead of deflecting responsibility, organizations must address the root causes of failure to cultivate a culture of growth and improvement.
The sin of wrath often stems from a lack of team alignment, where misalignment between strategists, execution teams and adoption teams leads to disjointed efforts and finger-pointing when things go wrong. Furthermore, a lack of proactive risk management leaves organizations unprepared for the inherent risks of early AI adoption. While missteps are inevitable in a complex digital landscape, the absence of resilience strategies transforms minor failures into major crises, breeding frustration, blame, and a significant erosion of trust.
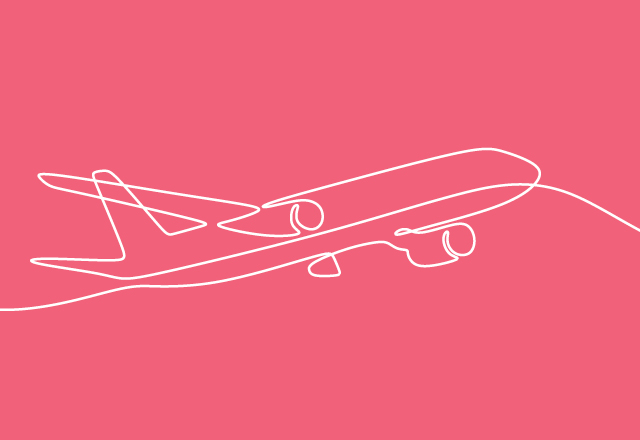
The 2024 Air Canada case serves as a stark warning. In 2024, Air Canada was ordered to pay damages to a passenger, Jake Moffatt, after its virtual assistant provided incorrect information regarding bereavement fares. The chatbot advised Moffatt to purchase tickets and claim a bereavement discount later, but his claim was denied, leading to a legal dispute. Air Canada argued it wasn't liable for the chatbot's error, but the tribunal ruled otherwise, emphasizing the airline’s failure to ensure the chatbot's accuracy. This incident highlighted the dangers of deflecting responsibility onto AI systems rather than addressing flaws in training, testing, and monitoring.
Redemption tip:
- Take accountability and learn from failures: Instead of deflecting responsibility, address the root causes of failures to cultivate a culture of growth and improvement. Regularly review and update AI systems to align with evolving business policies and establish clear escalation mechanisms for AI errors to human support. Accepting accountability and celebrating builds credibility and strengthens AI's role in enhancing customer trust and satisfaction.
- Promote a culture of innovation and open communication: Encourage open communication and collaboration to promote a culture of innovation and continuous improvement.
Avoiding the digital sins: A path forward
To unlock the true transformative power of AI, organizations must actively transcend these seven deadly sins. The path forward lies in: building scalable AI systems; adopting tailored AI strategies that address specific business needs; resolutely focusing on ethical and responsible AI deployment; and operationalizing AI with robust infrastructure that supports continuous improvement.
At Thoughtworks, we've witnessed firsthand how addressing these pitfalls unleashes AI's true potential. It's about embracing a disciplined and thoughtful approach, transforming potential sins into stepping stones toward genuine digital excellence and tangible business outcomes.
Let's collectively ensure an AI journey that is purpose-driven, strategic, and profoundly impactful — shaping a future where AI elevates human potential and drives unprecedented value.
Disclaimer: The statements and opinions expressed in this article are those of the author(s) and do not necessarily reflect the positions of Thoughtworks.