The hype around generative AI has been so extensive that it can be easy to lose sight of where it can really add value. This can easily lead us into pessimism — and although it’s important to be aware of the limits and risks of artificial intelligence, for business leaders, it can make it difficult to identify exactly where AI can add value. Is it just too risky? Is it really all just industry hype?
Of course, hype is par for the course when it comes to the tech industry. However, at Thoughtworks we’ve been working on a project that demonstrates how generative AI can have a significant impact that potentially enables truly transformative change for millions of people: Jugalbandi. In this article, we’ll explain what Jugalbandi is as well as some key lessons that we think are essential for any business exploring how they might leverage generative AI.
What is Jugalbandi?
Jugalbandi is an AI platform that underpins a conversational UI for hundreds of welfare programs provided by the Indian government. However, it’s more than just a chatbot — built using a combination of large language models (LLMs) such as GPT-4 and Indian language models, it enables voice-to-voice interaction with digital services in 11 different Indic languages (with the plan to increase the number of languages supported to 22 in the future).
This is hugely significant for people across the country. Due to linguistic diversity as well as large levels of illiteracy, it was previously challenging for many citizens to gain access to services and support they’re entitled to. With Jugalbandi it is possible for many citizens — even in more remote and rural parts of the country — to access information about services, such as funding support in industries such as farming, in their native language.
The impact of the project has been acknowledged by Microsoft CEO Satya Nadella. "The rate of diffusion of this next generation of AI is unlike anything we've seen," he said, “but even more remarkable is the sense of empowerment it has already unlocked in every corner of the world, including rural India."
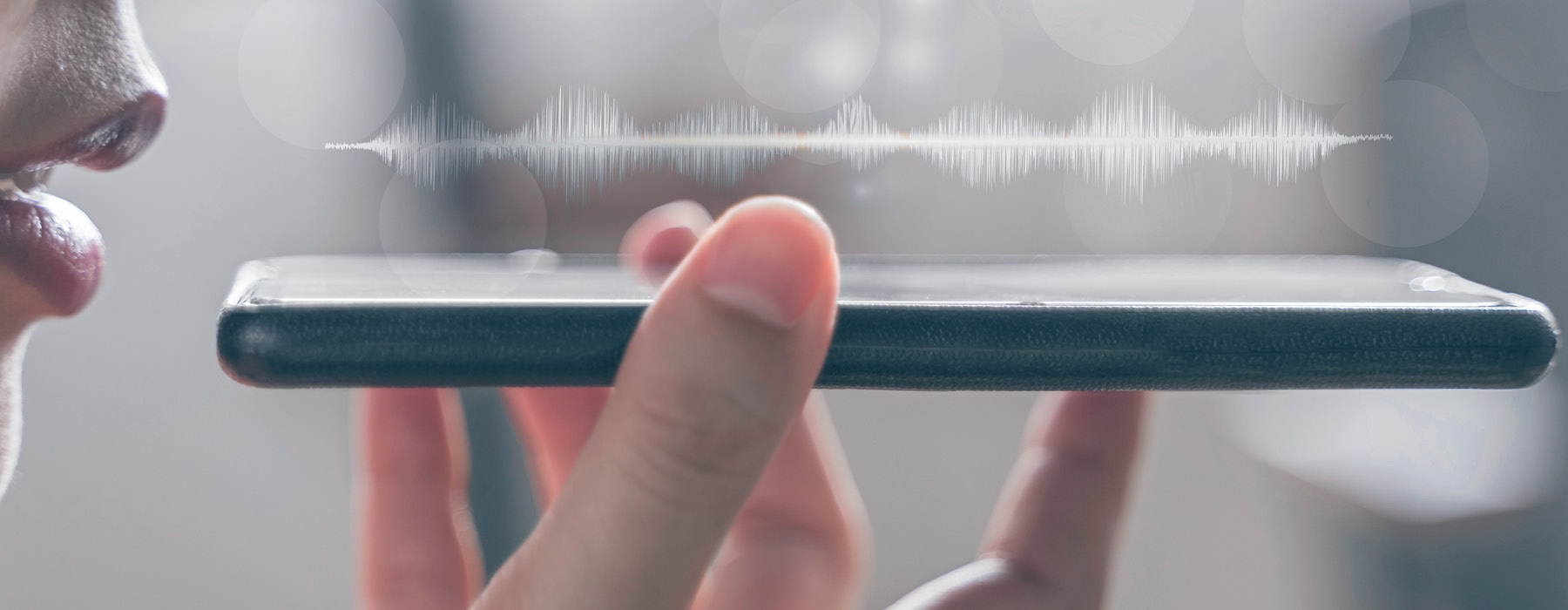
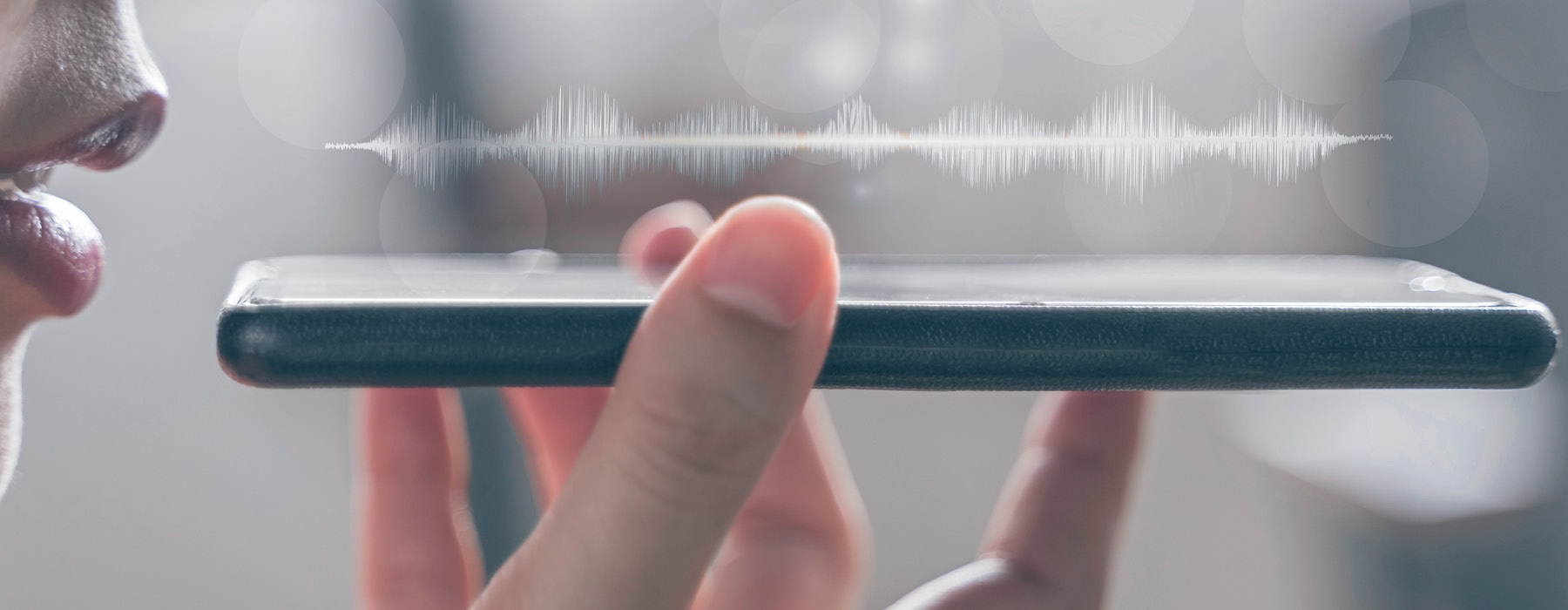
As important and impressive as the AI at the core of the Jugalbandi project is, it would be wrong to see it as transformation driven purely by technical innovation. In reality, the AI systems that power Jugalbandi are one component in a wider piece of work that requires local and domain knowledge, a strong relationship with institutional partners as well as a sensitivity to the needs of end users — ordinary Indian citizens.
Understand the problem and get close to local needs
Perhaps the most important lesson of Jugalbandi is the value of getting as close as possible to local needs. If we hadn’t done that, we could easily have built something ostensibly impressive and technologically innovative, but with little impact on end users.
We were able to do this partly due to our knowledge of some of the challenges facing Indian society, but also because we had such a strong partnership with institutions that had an acute — and long-running — awareness of civil society problems.
This is what ultimately led us to see the challenge not only as one of linguistic diversity but also one of literacy and the importance of oral interaction. It would have been easy to lapse into a bias towards text interaction, but by working closely with partners in the Indian government, it became clear that a different approach was essential.
It was for a similar reason that we developed the chatbot for WhatsApp. While there are many ways this service could have been delivered — we could have built something that runs in the browser, for instance, or even built a brand new mobile app — the extent of WhatsApp’s penetration across Indian mobile users meant it made sense to meet users where they are.
This means the barrier to entry for using a Jugalbandi-driven chatbot is exceptionally low. Anyone who uses WhatsApp to record and send voice messages — as many people do in India, especially if they lack certain literacy skills — can immediately start interacting with the tool that we’ve built.
The lesson for business leaders here should be obvious: cutting-edge technology can be incredibly exciting, but if you’re not putting the needs of your users first and working to solve their challenges, even the most ostensibly forward-thinking innovations will be useless. Spending the time exploring a problem-space and getting to know local needs is essential for ensuring AI initiatives have a substantial impact.
Designing the AI system to respond to those needs
It’s not simply a case of layering social awareness and understanding on top of a robust technical foundation; in reality the relationship between the social and the technical parts of Jugalbandi is symbiotic. Our technical implementation had to evolve according to the needs of users.
One of the major challenges we faced in the project was tackling what are today widely described as ‘hallucinations’ — LLM outputs that have seemingly been imagined by the system, information that sounds plausible but is, in fact, completely fabricated. These were particularly problematic in the context of Jugalbandi. Given the tool was intended to give people access to essential information about welfare schemes, if the chatbot offered inaccurate information to users, that could seriously hurt their ability to access schemes designed to support them. It would also ultimately undermine the whole project; trust would deteriorate and all our work would be wasted.
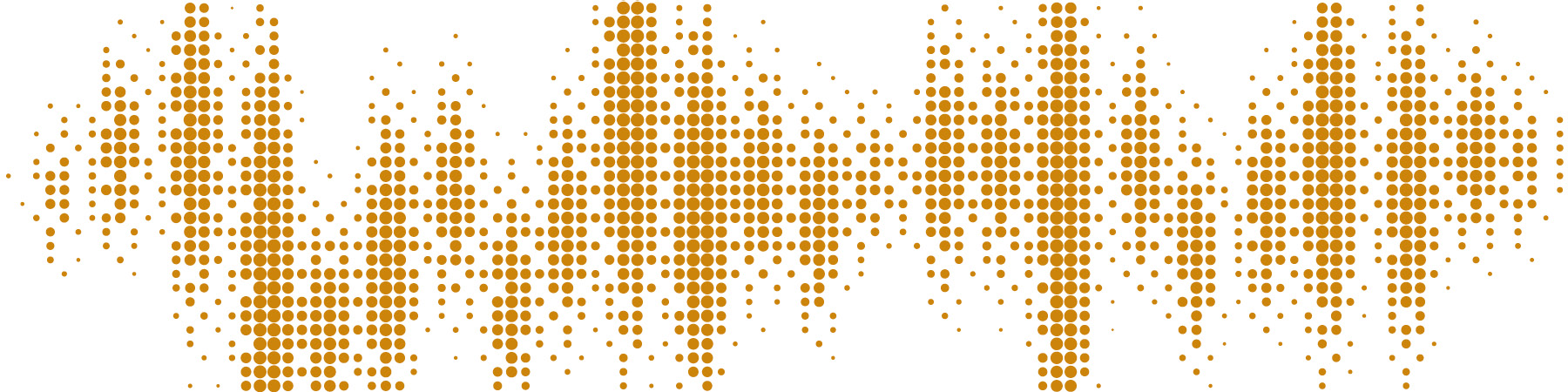
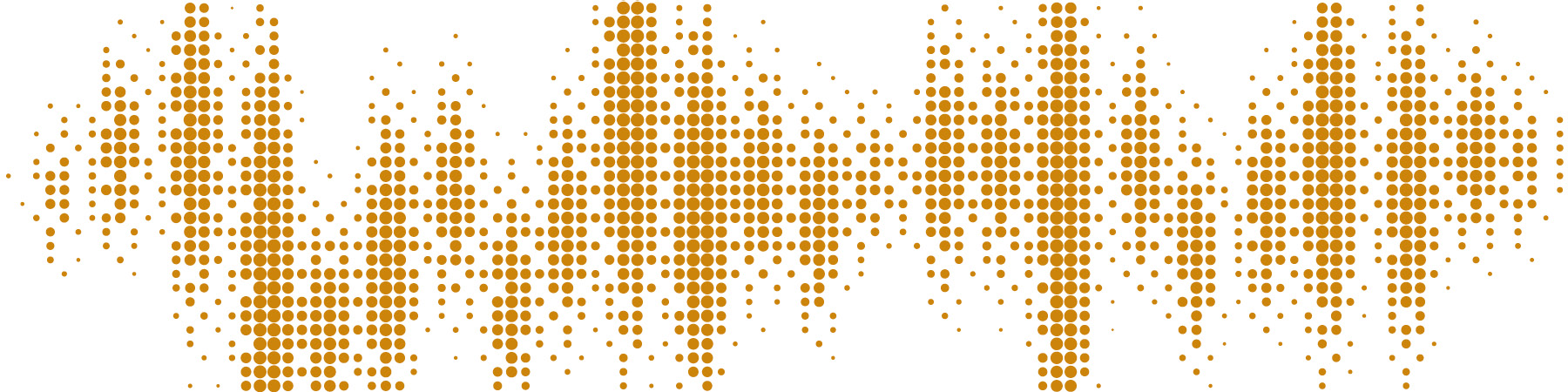
To tackle this issue, we spent time thinking through the ways we might minimize the risk of hallucinations. A useful technique here was something called retrieval-augmented generation, a way of ensuring the LLMs that form the foundation of your generative AI system are underpinned by distinct facts found in a distinct data set (or corpus). However, we wanted to go further to ensure what we were building was as reliable as possible for users; as a result, we developed a further iteration of RAG, to something we termed Post-RAG. Post-RAG is a layered approach to retrieval-augmented generation, which breaks down the various parts of the infrastructure (all the way from the UI through to the LLM) to ensure greater control and improved accuracy of the chatbot’s outputs.
This isn’t the place to go into the technical details of Post-RAG, but you can learn more about it here. What’s key is that our technique allowed us to even more tightly define the knowledge boundaries of the LLMs used by the Jugalbandi chatbot. That means the chance of hallucinations is kept to a minimum.
The work we did here won’t be relevant to every implementation of generative AI. Indeed, in some projects, hallucinations might even be more valuable than fidelity to a knowledge base — this is particularly likely to be true if you’re using generative AI for creative work or ideation.
The lesson, then, is to adapt and evolve your technology solution to meet the needs of the project. You can’t just insert ChatGPT into a problem and expect results. Indeed, if you’re working with sensitive personal information, you’ll have to think outside of existing public products — you may need a more bespoke and self-hosted approach to LLMs. Whatever challenges you’re trying to solve, be open-minded about the technical approach needed.
Build on your learning and explore new applications
Although the Jugalbandi chatbot for Indian government schemes is the most notable tool developed by the project, we’re also expanding the scope of our work and applying what we’ve learned to other domains. At present, our focus has been on the legal sphere; this maybe isn’t that surprising given this is a field known for large amounts of dense information.
Our current projects include something called Judges Virtual Assistant (JIVA), which will help judges find legal information in dense archives of documents more quickly and easily than ever before, and Justice Access for Grievance Redressal using Intelligent Technology (JAGRIT). The principles behind these initiatives will build on everything we’ve already done, but make no mistake; we do not view this as simply a case of applying the same tool to a different problem — we’re spending time exploring some of the challenges and adapting our existing technology to the needs of a new domain and new challenges.
The lesson for business leaders here is that it’s important to be open to further applications of AI. You don’t necessarily need to think big at the start; having a singular challenge on something specific can provide a fruitful foundation not just for future exploration but for real, tangible impact in other areas.
A template for effectively leveraging AI
Jugalbandi is a unique project. However, there are still important lessons for enterprises wishing to explore the possibilities of AI (generative or otherwise): think local, put user needs first and be both technically and socially responsible to change. These points aren’t just applicable civil society technology projects: they’re crucial to any organization using AI, even those with commercial interests.
Indeed, these lessons may be even more important in a commercial context. Paying attention to what’s required to ensure AI initiatives have real impact isn’t just a question of social responsibility: it also means you’re doing what’s necessary to get the most value for your investments in cutting-edge technology projects.