For AI solutions to achieve lasting value, businesses need an iterative strategy paired with experimentation, robust engineering practices, and guardrails.
Whether you think next-generation AI heralds an exciting new world for humankind or sows the seeds for its destruction, few business leaders can afford to ignore it. But in this febrile environment, it can be hard to plot a course that neither falls foul of the hype nor misses the opportunity entirely.
You need only look at the breakneck advances being made in generative AI to appreciate how fast the field is moving. OpenAI’s ChatGPT was released publicly in November 2022. The updated version, based on the GPT-4 large language model, offers a step change in capabilities to the extent that some Microsoft researchers gushed that it showed the “first sparks of artificial general intelligence.” A slew of kindred tools, from Midjourney and Stable Diffusion to Voicebox, are pushing the boundaries of what user-friendly AI tools can do.
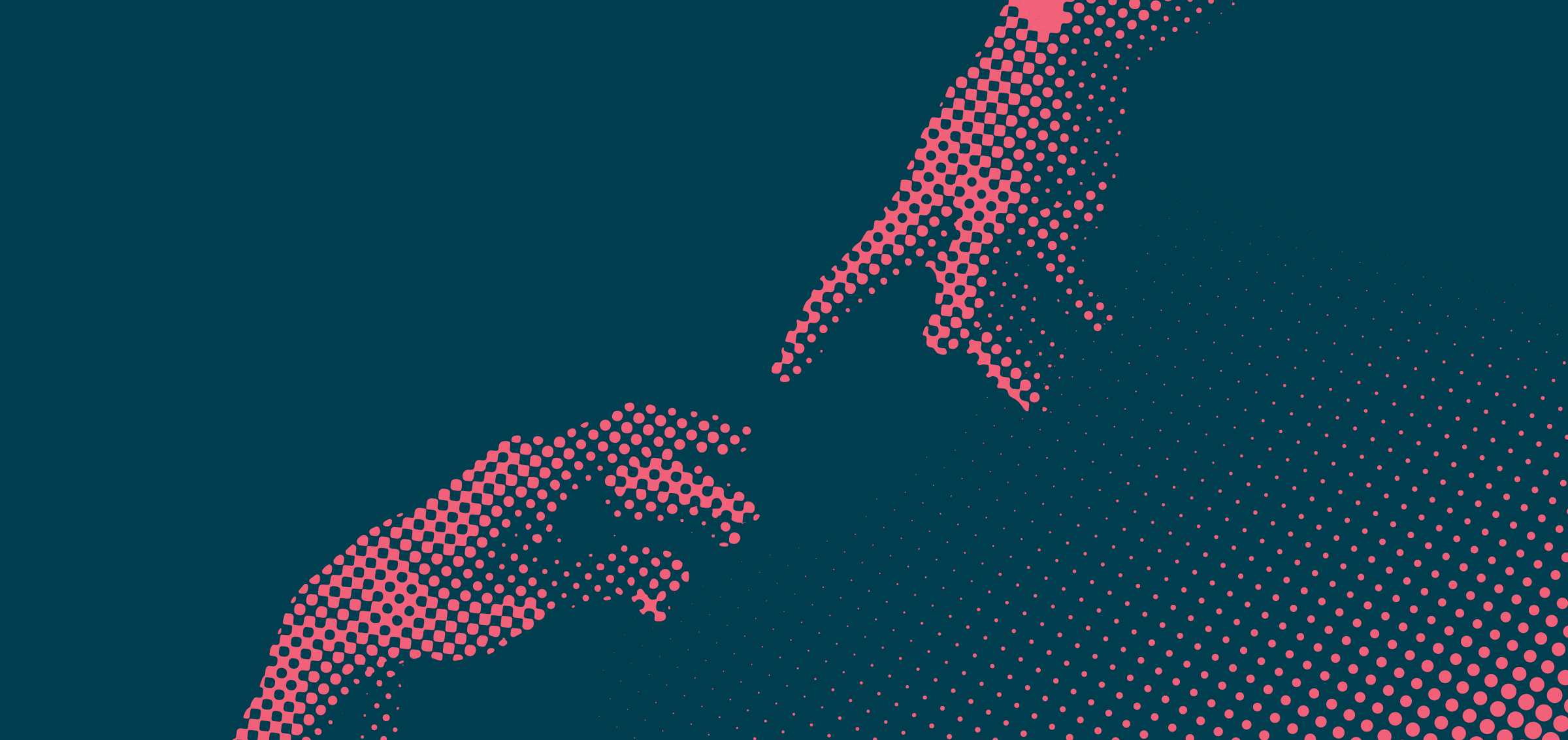
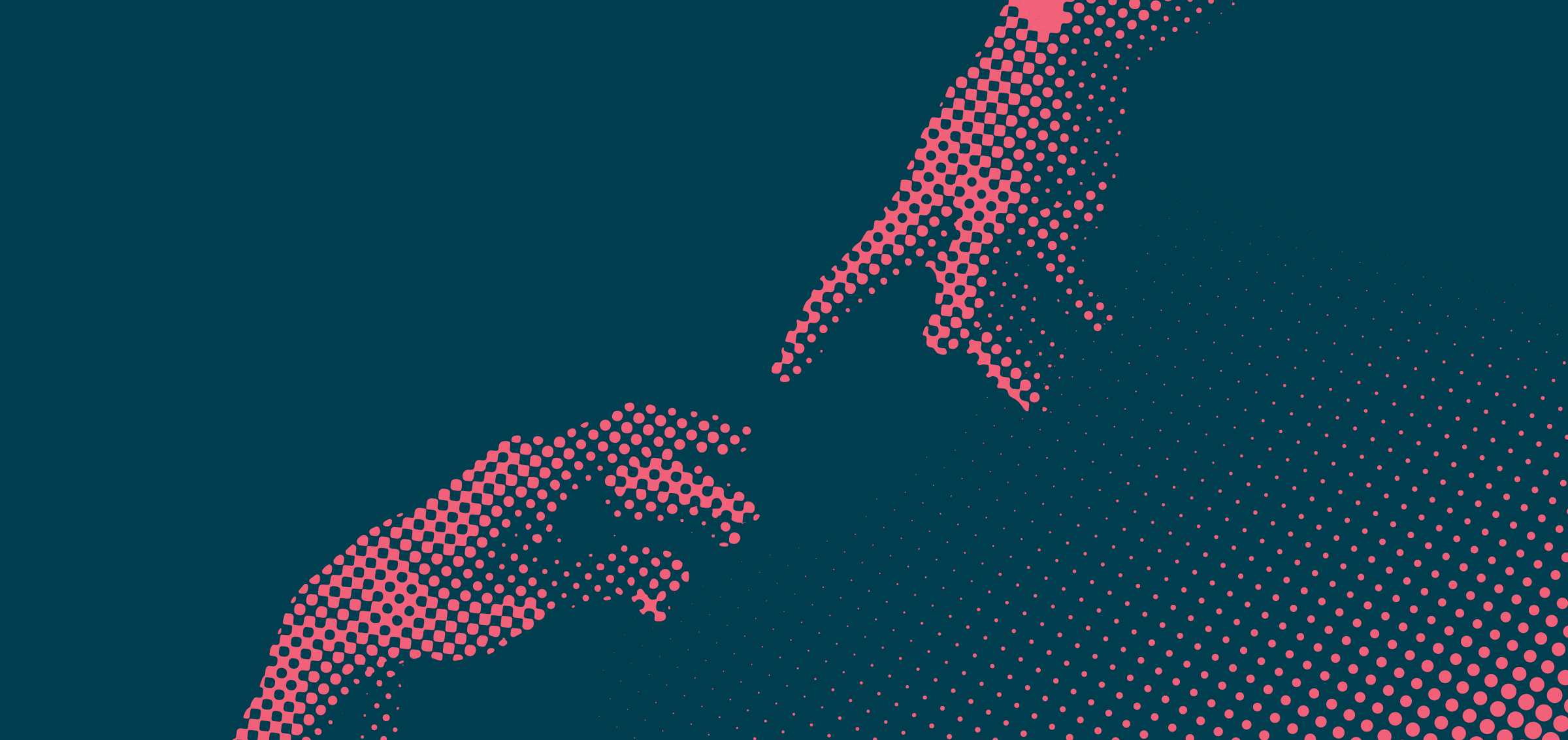
Every business needs to explore the AI opportunities that are opening up — yet, they don’t need to buy into such hyperbole to appreciate these opportunities.
Relentlessly refresh to stay ahead
AI is already pervasive. Many organizations are leveraging it to glean insights from the oceans of data they have access to. But this period marks a sea change, catapulting AI forward in the user experience. The real challenge facing today’s business leaders is this: How can a business capitalize on AI capabilities today while planning for its future trajectory?
For many enterprises that means rebuilding your AI strategy to account for the likely evolution of generative AI (GenAI). It’s already easy to see GenAI’s potential to impact functions such as information technology, customer service, sales and marketing, and product development. But every function — indeed every industry — can expect to be reshaped by AI. No business leader can afford to be caught off guard: To maintain market share, or even outpace the competition, your AI strategy needs to be constantly refreshed. Otherwise, you risk decreasing your operational effectiveness. Worse, products and the market might change in a way that doesn’t allow your business to keep up.
To be effective, your AI strategy needs to first appreciate that AI is not a specific tool but an approach that can be embedded into any number of applications, processes or potential solutions. Increasingly, the thing that gives an organization an edge is the ability to integrate AI into the business. Your ability to build or consume solutions isn’t necessarily going to be your differentiator — instead, it’s your ability to integrate these solutions into your processes and products.
And your AI strategy must be continuously updated to reflect the changing landscape. At Thoughtworks, we’ve taken our learnings from continuous delivery practices and applied them to AI, so that clients make fast feedback a central tenet of their strategy. This enables them to identify where they’re gaining value or pivot when tech advances open up new fields.
Benefits of AI continuous delivery practices
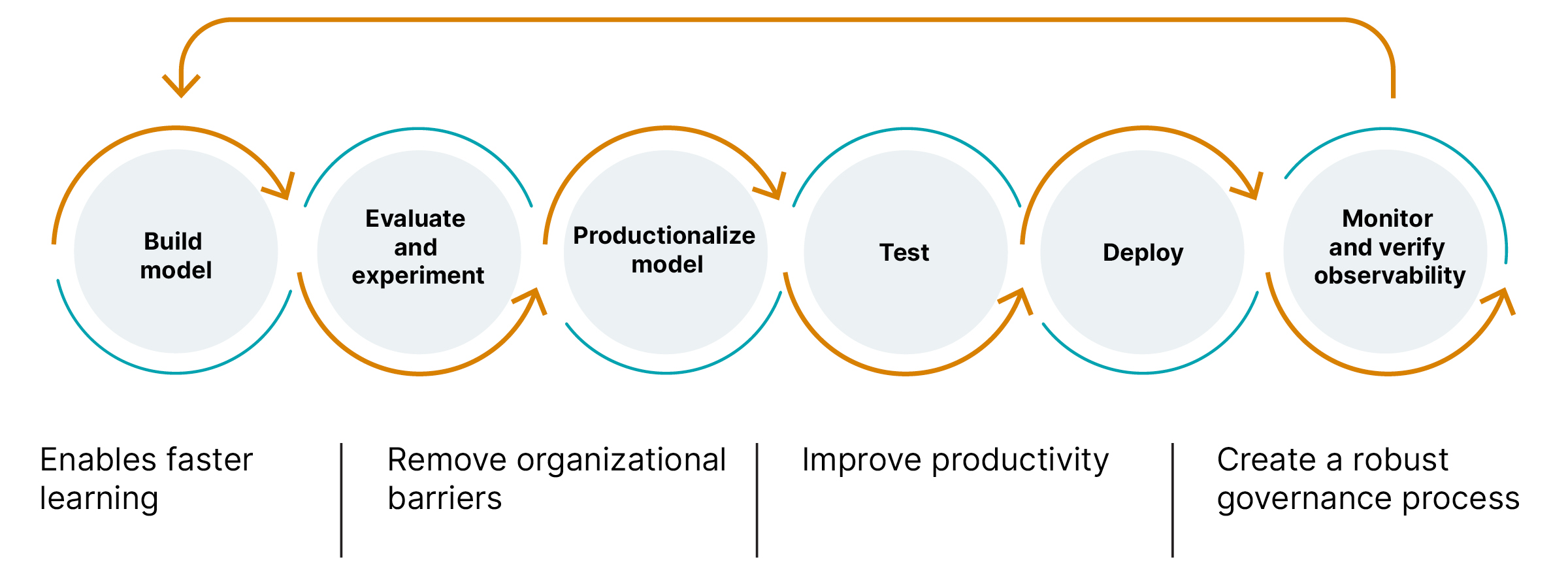
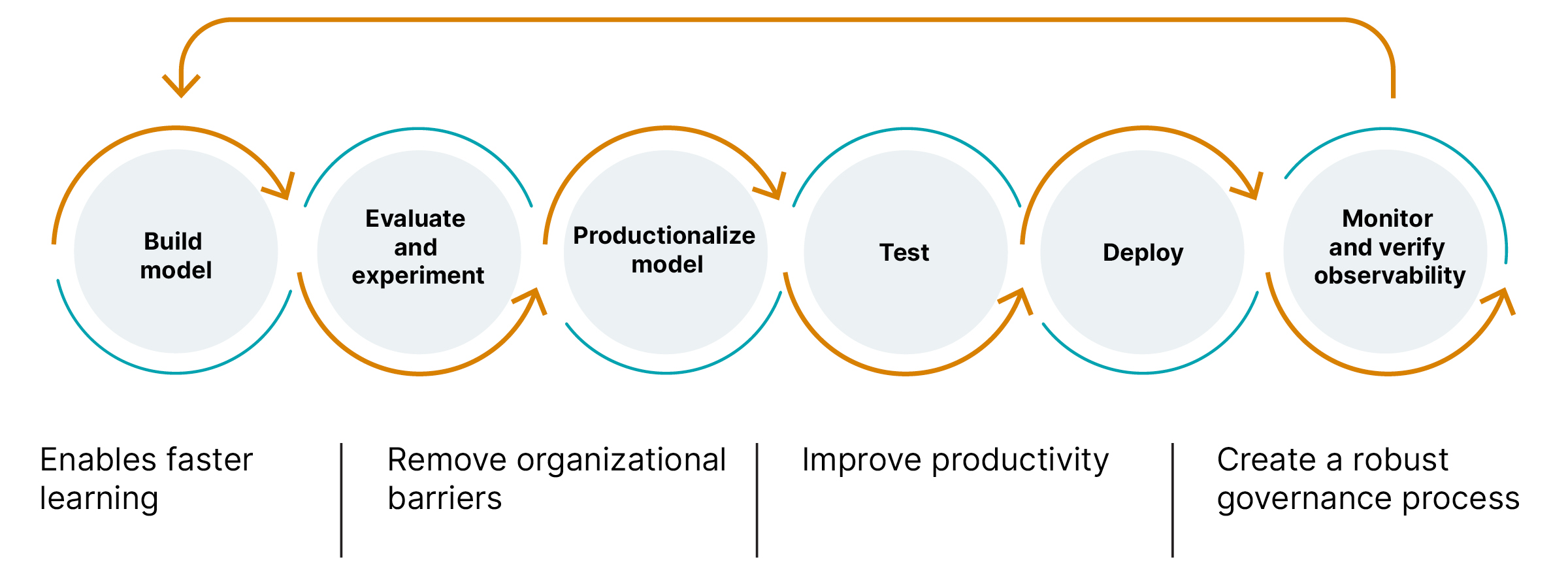
Don’t starve on low-hanging fruit
Given the huge advances we’re seeing in AI right now, it’s not surprising that many organizations have pockets of experimentation spread throughout their operations. At Thoughtworks, we think that’s a good thing: Experimentation can rapidly identify use cases with serious potential for your organization.
But there’s also risk: If you don’t plan from the outset how you intend to scale successful proofs of concept — and embed them in the business — there’s a real chance that you’ll only pick off the low-hanging fruit. To avoid this, you should identify opportunities across the business to leverage the same solution or approach multiple times.
It helps to plan to leverage AI for things beyond mere efficiency — like improving ideas. Sure, ChatGPT might help you create that business proposal in record time, but why not look at how GenAI can help you generate ideas, refine designs for products and services, or understand your strategic options?
For one of our clients, one of the world’s leading snack food producers, AI is supporting elements of recipe creation, which is a historically complicated task given the dozens of possible ingredients and ways to combine them. By partnering product specialists with AI, the organization can generate higher quality recipes faster. The organization’s system has reduced the number of steps needed to develop recipes for new products from 150 (on average) to just 15. Now, it can more quickly delight customers with new products and new experiences to keep them connected to the brand.
Notably, AI does not work in isolation but rather augments skilled teams, providing guidance and feedback to further improve outcomes. This is a hallmark of successful AI solutions: They are ultimately designed for people, and a multidisciplinary team that comprises domain and technical expertise as well as a human focus, to enable organizations to get the most value out of them.
Guardrails matter
When thinking about how to get the most from AI, your AI strategy should also consider the appropriate guardrails.
As solutions become more sophisticated — and embedded more frequently and deeply into software, products and day-to-day operations — their potential to allow people to make mistakes increases, too. One common antipattern we see is when humans become unintentionally over-reliant on fairly stable AI — think of the developer who doesn’t check the AI-generated code, or the Tesla driver lulled into a false sense of security by the car’s autopilot features.
There needs to be careful governance parameters around usage of AI to avoid that type of over-dependency and risk exposure.
While many of your AI experiments might produce exciting ideas to explore, you need to be mindful of the tools that underpin them. Some AI solutions are not built following the kind of robust engineering practices you’d demand for other enterprise software. Carefully think about which ones you’d be confident deploying into production.
It helps to test AI models in the same way you would any other application — and don’t let the rush to market cloud your judgment. AI solutions should be supported by the same continuous delivery principles that underpin good product development, with progress made through incremental changes that can be easily reversed if they don’t have the desired impact.
You will find it helps to be up-front about what you consider to be a “desired” result — it may not only be financial metrics that define your success. Depending on your organization’s context, productivity and customer experience might also be important considerations. You might look at other leading indicators, such as your team’s awareness of the potential of AI and their comfort level in exploring, adopting, or deploying AI solutions. These factors can give you confidence that your team is on track toward improving any lagging indicators of customer experience, productivity, and revenue. However you approach it, you’re more likely to succeed if you’ve identified those metrics at the outset.
Finally, for all the bluster about the threat AI poses to people’s jobs — or even to humanity at large — you’ll do well to remember that it’s your people who will be using the technology. Consider the human side of change, where you strike a balance between encouraging people to adopt and innovate with AI while remaining sensitive to the problems it can present. You might, for instance, want to introduce guidelines to protect intellectual property in models that draw on external sources or privacy, where you may be using sensitive customer data. We often find it’s better to give our people a say in where AI augments their work. They know, better than anyone, where it can have the most impact.