Generative AI (GenAI) is forecast to become a $1.3 trillion market by 2032 and represents one the biggest and clearest opportunities for organizations across a huge range of industries. But when you’re building and implementing your own GenAI use cases, success is far from guaranteed.
Between 60% and 80% of all AI projects fail. There are a lot of different factors that contribute to or cause AI project failure, but the most common we’ve encountered are organizations leaping in to embrace GenAI without first establishing an AI-ready data foundation, or articulating how GenAI will integrate into their workflows to create value for employees and customers.
Generative AI demands highly capable data platforms
To generate valuable, reliable outputs, AI models require significant quantities of discoverable, high-quality data. For many organizations taking their first steps into this space, that’s where things get difficult.
It’s a fresh area of digital opportunity, but one that requires organizations to tackle many of their underlying data challenges before it can be fully exploited and used to create business value. Practically, adopting GenAI requires teams to do five key things:
1) Align their data and AI strategies: If GenAI is about to become one of the most demanding use cases for your data, your data strategy must evolve to support it. Strategies should be aligned to ensure the right data is gathered, processed and held in the right ways to fuel your models and apps.
2) Embrace data product thinking: Generative AI is, as its name suggests, a generator of valuable outputs. But, it’s also a consumer of data. So, it’s very useful to start managing and treating your data as a product, and evolving your products around the needs of the apps consuming it.
3) Automate as much as possible: Getting AI models into production can be a lengthy and complex process. MLOps can help you automate training, deployment, monitoring, and feedback cycles to help you start generating value from GenAI quickly, and in a repeatable way.
4) Create repeatable, auditable processes: Responsible and ethical AI use demands total process transparency. By building repeatable, auditable processes, you can streamline AI governance, and ensure that models are explainable, and your operations stay compliant with emerging regulations.
5) Build robust data platforms: Every organization using GenAI needs a data platform that’s equipped with the right capabilities to ensure that the right data of the highest quality is available and delivered in the right way.
Let’s focus on that last point and explore how teams can build data platforms with the capabilities to support their generative AI goals.
The 4 levels of data platform evolution for generative AI
The key to successful GenAI projects is ensuring that your data platforms have the capabilities required to support and enable it. Broadly speaking, the capabilities your data platform needs will depend on the depth and sophistication of your GenAI plans.
To illustrate that, we can break down data platform evolutions for GenAI into four levels.
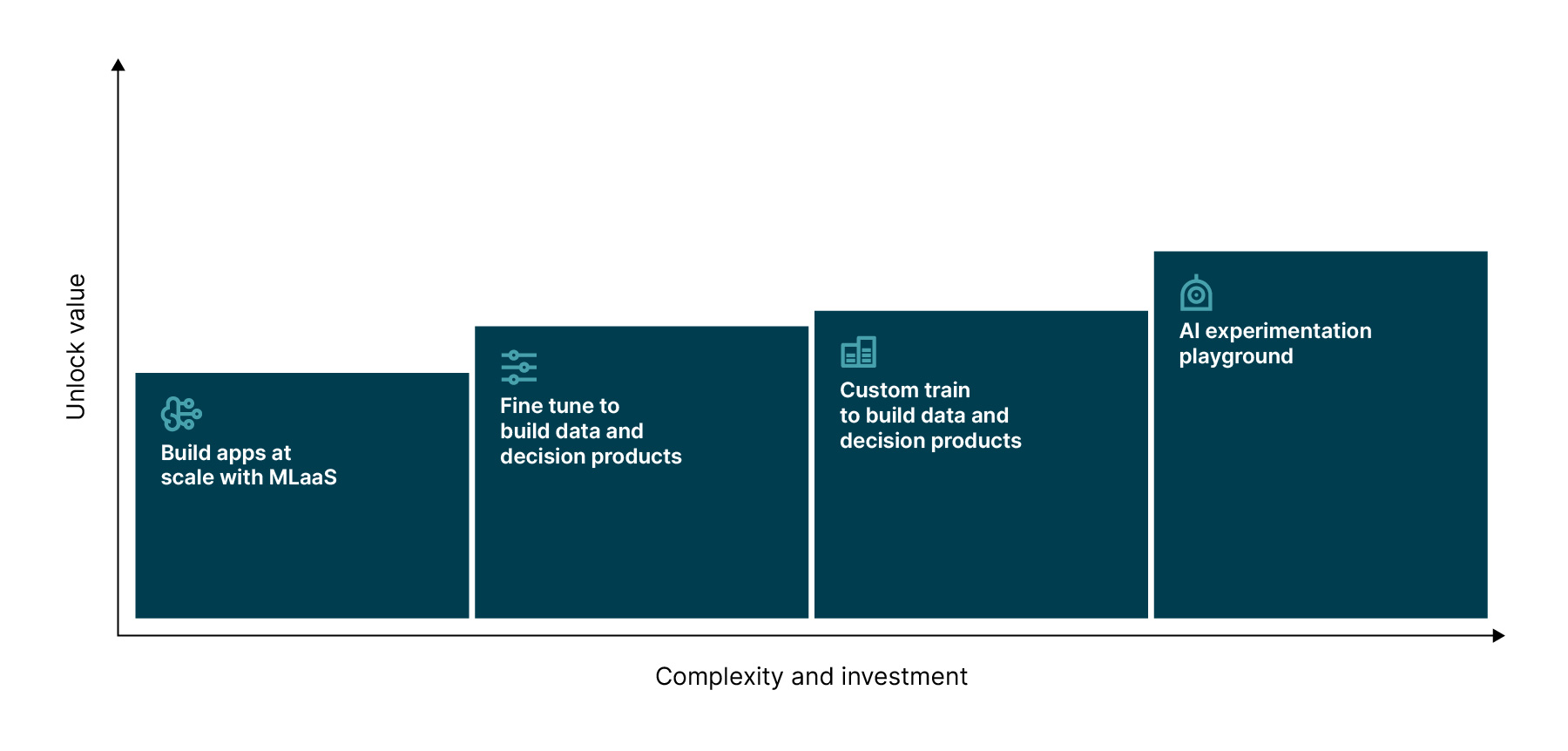
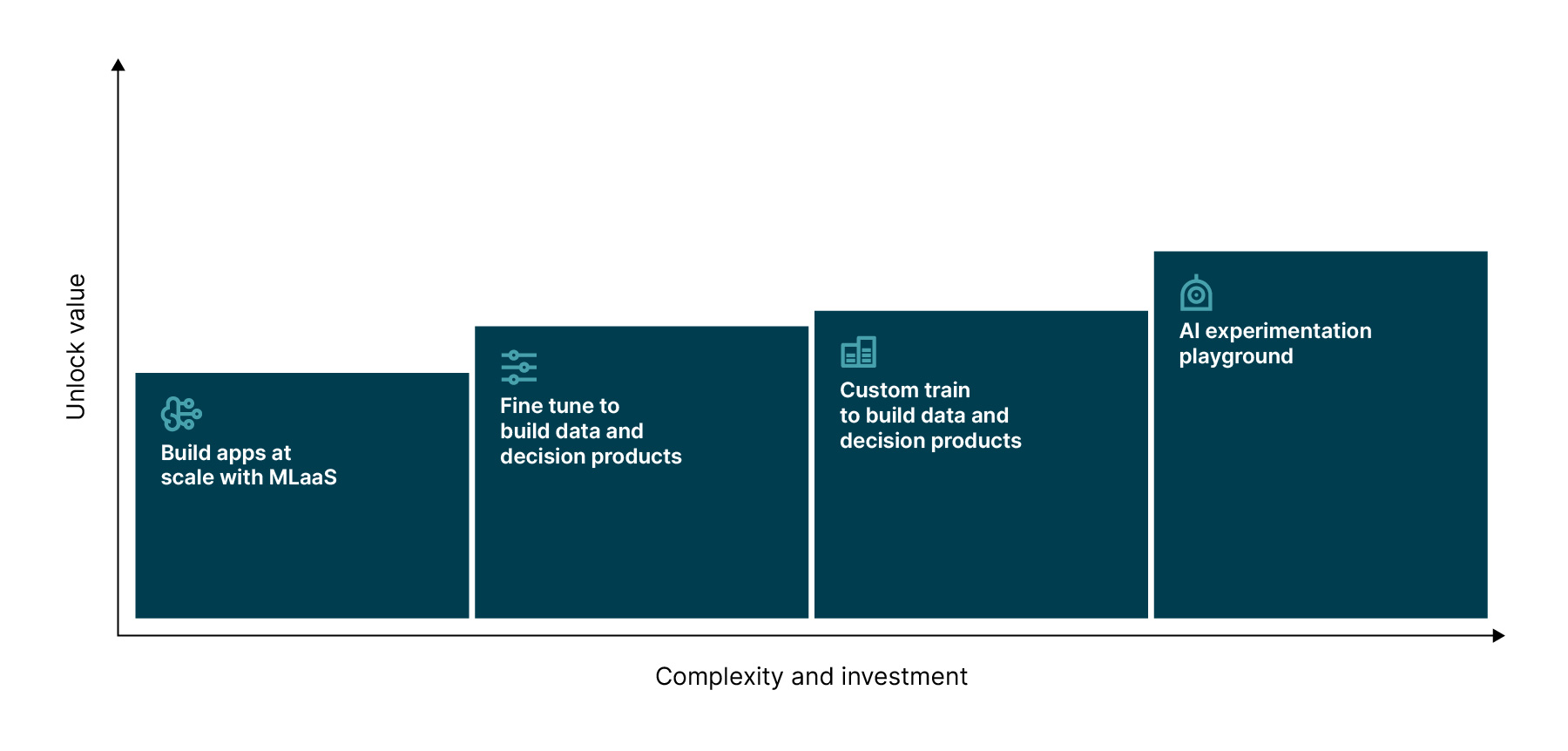
Level 1: Building apps at scale with SaaS LLMs
As the generative AI market has grown, numerous entrants have emerged, offering SaaS-based Large Language Models (LLMs).
For most organizations — we estimate around 80% — these models and tools are sufficient to build GenAI apps that are aligned with their business strategy. They can improve and streamline internal processes, and present a rapid route to enterprise adoption.
Even at this level, it’s important for teams to ensure that they have the capabilities in place to continuously improve generated outputs and reduce hallucinations. Retrieval Augmented Generation (RAG) can help you create enriched prompts by adding contextual information from trusted sources. That trusted source generally comes from embeddings. And to manage embeddings, you’ll need to use Vector Databases.
By building embeddings as a service within their data platform, each domain can fine tune embedding models to ensure they’re perfectly aligned with their specific GenAI use cases.
Level 2: Fine-tune pre-built SaaS or open source LLMs with enterprise domain data
If SaaS or open source LLMs can’t achieve what’s required straight out of the box — due to reasons like exceeding maximum context window size per prompt — organizations have the option to augment and fine-tune those models. However, because this approach depends on their own enterprise data, it demands a significant step up in data platform capability.
To support fine-tuned GenAI models and applications, organizations must view and manage data as products — in line with product thinking — and ensure their data products are Findable, Accessible, Interoperable, and Reusable (FAIR).
They’ll also need to develop a robust compliance posture, and ensure they have the right processes, guardrails, and transparency to use data and AI responsibly. That means keeping all data products and processes explainable.
In the same vein, high levels of data privacy and security must be upheld, to prevent data being compromised or tampered with in ways that influence the outputs generated by AI applications.
The final technical requirement at this level of evolution is to apply MLOps to enable the effective productionizing and continuous improvement of LLMs at scale.
Level 3: Pre-training open source model architecture to build a bespoke model
If an organization has very niche AI requirements, fine-tuning existing models might not be enough to support them. Those teams will need to consider training an open source AI model from scratch using their own enterprise data.
In addition to the capabilities required to fine-tune SaaS-based models, organizations that want to train open source LLMs using their own data to create bespoke GenAI apps will need vast quantities of FAIR enterprise data.
To gain that scale of data, most teams will need to evolve their data platform to support the generation of synthetic data, to bulk out their existing data products and enable imitation learning.
With models generating outputs purely on an organization’s enterprise data, teams will need to enable extensive testing, evaluation, and validation processes. Data platforms must be equipped to understand data quality at deep levels, so that teams can robustly evaluate how trustworthy the outputs are, and continuously improve them over time.
Level 4: Building capabilities for continuous AI experimentation
If you want to move from building point apps to embedding GenAI in your organization at a fundamental level, you need to look beyond individual models and apps, and evolve your data platforms to build an environment for continuous AI experimentation.
Platform capabilities can help you create an environment where every domain and team is empowered to pick the right GenAI app or tool for all use cases — effectively building your own model marketplace. This enables organizations to build an AI-first culture, and continuously mature their use of GenAI and ML.
At this level, organizations and their data platforms need the capabilities to support the training and running of diverse model types, including GANs, VAEs, LLMs. Crucially, they also need the ability to monitor performance accurately at scale, to help detect hallucinations and bias, and maintain explainability and transparency across all enterprise AI use cases.
That’s a significant undertaking, and demands significant operational, organizational, and data platform evolution. But to gain the maximum value from GenAI and embed it at a fundamental level, it’s a necessity.
Take the first step to generative AI success and data platform evolution today
We have numerous diagnostics and frameworks that can help you better understand your needs. We also offer clinics to help you assess your generative AI readiness specifically, or evaluate how to get greater value from your data overall. Let’s talk.
Disclaimer: The statements and opinions expressed in this article are those of the author(s) and do not necessarily reflect the positions of Thoughtworks.