Now and in the future, companies will still win in challenging times by achieving better cost positions, delivering superior customer experiences, or controlling an industry standard. Virtually every element of how firms pursue these strategic goals, however, will look quite different. With recent events amplifying the rate of transformation, the digital divide between organizations has never been clearer. Enterprises must develop five key capabilities if they are to survive and succeed. Thoughtworks’ digital fluency model helps organizations define the investment required to become a modern digital business resilient to increasing disruption.
This article will focus on one of these capabilities in particular: Intelligence-driven decision-making.
Why?
Because better decisions enable better outcomes.
Being able to make better, more proactive decisions can provide a significant competitive advantage for an organization. By proactively anticipating and addressing potential challenges and opportunities, organizations can quickly adapt to changes in the market, improve their operations, and enhance their reputation with stakeholders. Moreover, higher-quality proactive decision making helps to reduce the risk of negative consequences and increases the likelihood of successful business outcomes.
Strong decision making can also boost employee morale, as it demonstrates that the organization is forward-thinking, and invested in its success. In turn, this can help to attract and retain top talent, which is essential for long-term success. Ultimately, organizations that make better, more proactive decisions are better equipped to create market opportunities, quickly overcome setbacks & retain talent.
However, building such a capability is as much a change of culture and mindset as it is technology-based. Leaders therefore need an approach that encapsulates this at an enterprise scale. Thoughtworks has worked with a number of organizations to amplify their decision-making abilities. Based on what we’ve learned, we suggest organizations can evolve their maturity by increasing the scope of where decision intelligence is applied, focusing change through three perspectives of the enterprise:
- The decision product: transforming the cognitive workload at the level of individual decisions
- The decision journey: optimizing entire processes rather than individual activities
- The decision platform: empowering multi-scale, multi-timeframe experimentation across the organization.
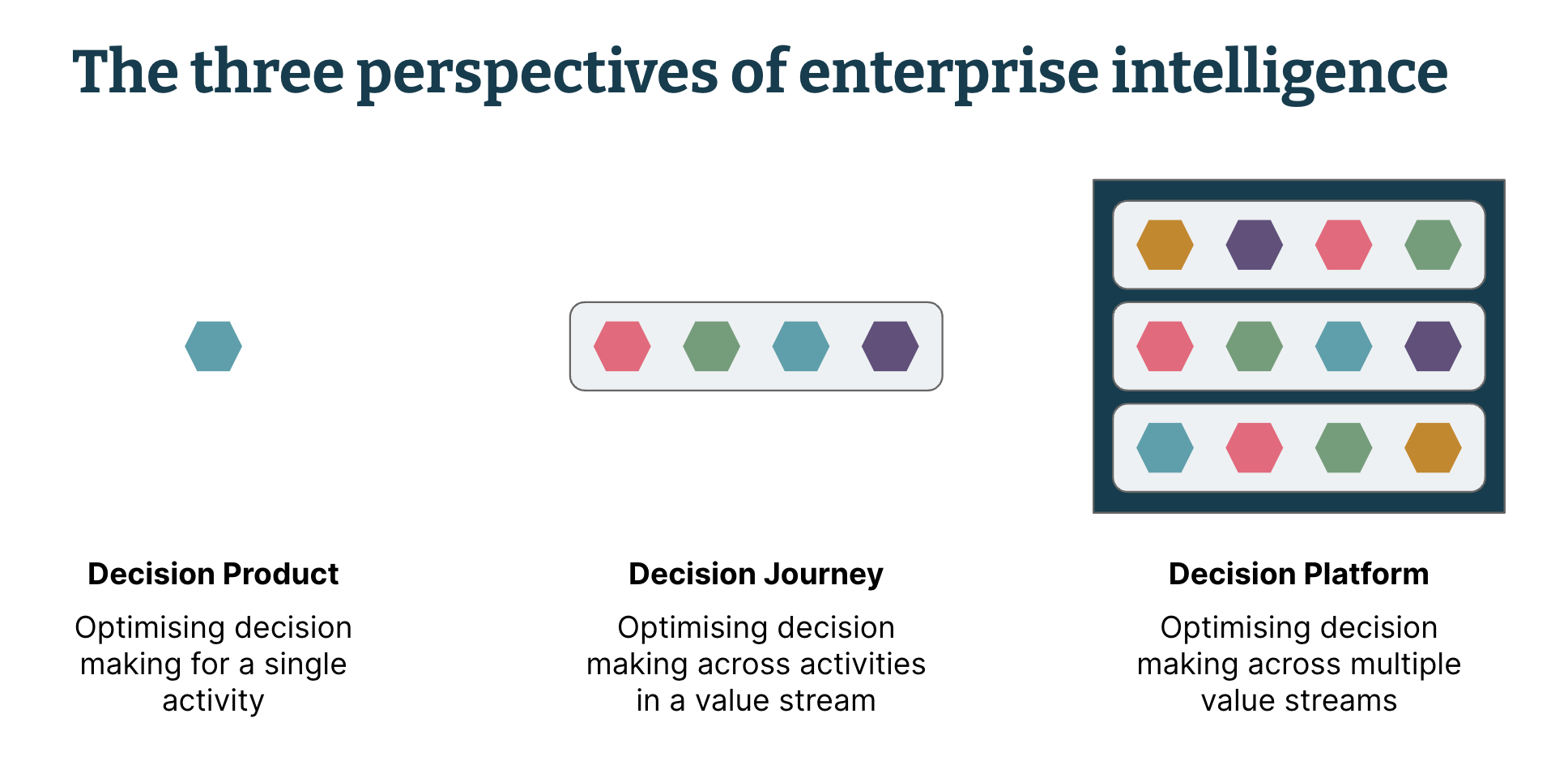
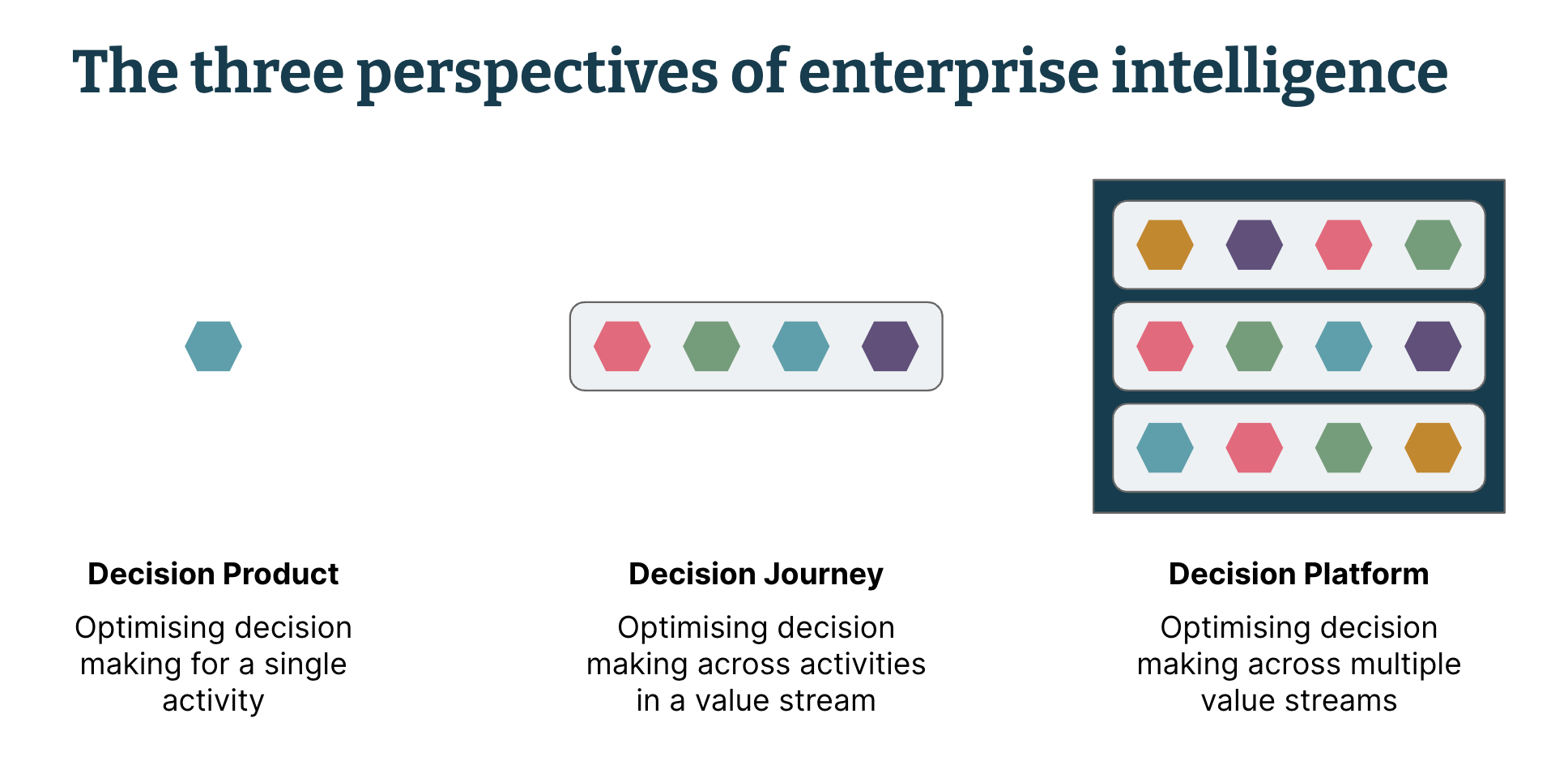
Each perspective describes a balance between targeting local or global optima by varying the decision interconnectedness considered at each level. This wraps up much of the complexity of delivering connected decision making at scale, drawing focus away from describing technologies, towards articulating the outcomes for tangible pieces of work that can be delivered incrementally. Crucially this approach aligns with the EDGE operating model, empowering leaders to build capability through a combination of initiatives from each perspective - de-risking the overall transformation and unlocking the intelligence-driven enterprise to make better decisions, faster and at scale.
Let’s now explore these perspectives and how they should be used.
The decision product
A decision product has a focus at the level of an individual decision or process step and, much like a data product, product thinking is used to deliver on the concept of "data as a product." Unlike a data product, its goal is to transform the cognitive workload of the decision-maker, allowing them to concentrate on higher value activities.
“This means reducing the time people spend tackling today's problems, creating the headspace to think about tomorrow’s possibilities. “
To achieve this, organizations must identify the best balance between human-led and machine-led decisioning. This will differ for each type of decision, with approaches falling into one of three categories:
Analyze: decisions are heavily human-led, with machines playing a supporting role providing descriptive analytics based on past and present data that feeds into a person’s analysis and ultimate decision on how to steer the process.
Augment: decisions are a collaboration between humans and machines. Machines use a mixture of diagnostic and predictive analytics, learning from the past and exploring possible futures, to co-create recommendations and support in their execution.
Automate: decisions are machine-led, and monitored by humans. Machines use prescriptive analytics with real-time feedback loops to make high-frequency, low risk decisions.
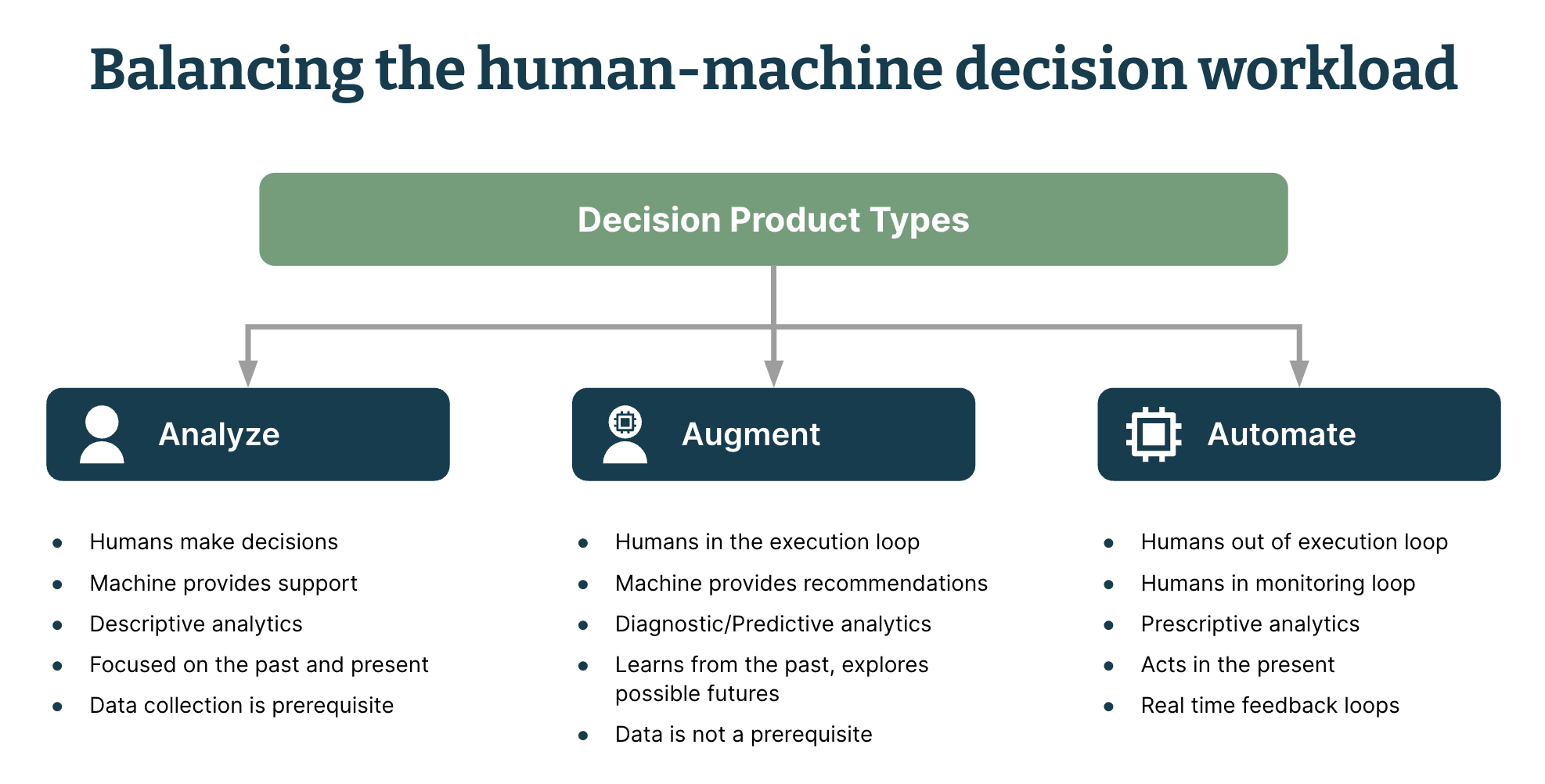
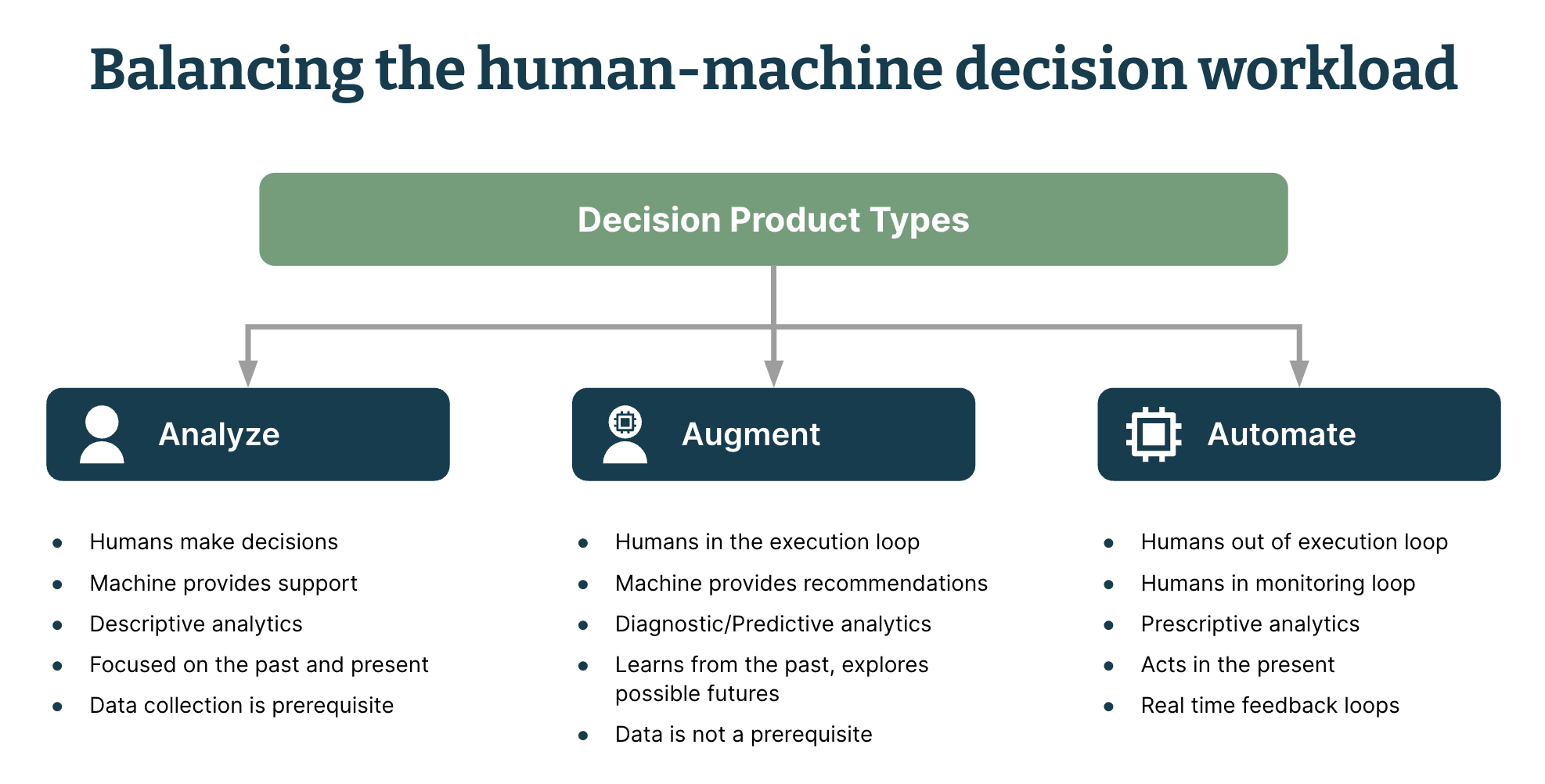
The choice of approach for each decision product must be established from the start and will depend on the pattern most suited to enabling its users. Even with automation, humans are never completely out of the picture, instead moving to a “managerial” role where they curate the possible outcomes and assess performance. In fact, AI should be thought of as a co-worker, and we believe partnering with AI is the best way to apply it. The ultimate situation would be to get to a position where people and machines are learning from and enabling each other.
Dynamic pricing is a good example of this. To be competitive in the world of e-commerce, pricing needs to be dynamic. Brands must be able to respond to changes in demand, perception and competitors in real-time, updating their pricing by the hour, by the minute, or even by the customer. Traditional manual and rule-based pricing systems can’t handle this level of speed and can lead to 5-10% in gross margin being left on the table.
Automating this process by using reinforcement learning (RL) to optimize the price in response to rapid fluctuations in demand can drive up profits. While this automation looks to transform the cognitive load away from manual pricing updates to the more important strategic work of what to sell and when, it’s critical to ensure this is actually the case for pricing teams. If they don’t understand or, more importantly, trust the pricing recommendations then they will continuously reject or adjust them, creating an alternative manual, low-value workload. Ensuring the tooling is designed as a decision product, optimized for use by the pricing team and not just a metric, will ensure successful uptake and increased margin.
The decision journey
Along every business process, there are numerous decisions that need to be made. While decision products use analytics and AI to optimize each of these decisions, they only consider them individually, in insolation. This can lead to competing priorities as each product tries to optimize within its own scope, not considering the wider implications.
Returning to the dynamic pricing example, the algorithm will use feedback from purchases to optimize pricing. If the customer didn’t buy the product at the recommended price then it’s not an optimum price. The customer, however, might have been influenced by other aspects of that process. For example, a marketing product could be running a campaign for an alternative product at a discount - creating competition that unnecessarily drives increases in marketing spend and decreases in margin.
To prevent this, systems thinking can be used to consider the process holistically, creating a journey of decision-making along it to deliver end-to-end value optimization. This will involve striking a delicate balance between striving for the global optimum and local optima. At points along the stream, it will be beneficial to optimize just for the decision in scope, at others, the overall goal will take precedence.
We call this a decision journey - a framework for integrated decision-making across an entire process. Each journey is made up of four constituent parts:
- Process: the sequence of activities that deliver value to a stakeholder. It can be external (e.g. a customer journey) or internal (e.g. a value stream).
- Decision points: the distinct decisions that inform how the process is executed or improved upon.
- Decision products: the collection of intertwined models and tooling improving the decision-making process.
- Data products: the products that surface the data required to make decisions.
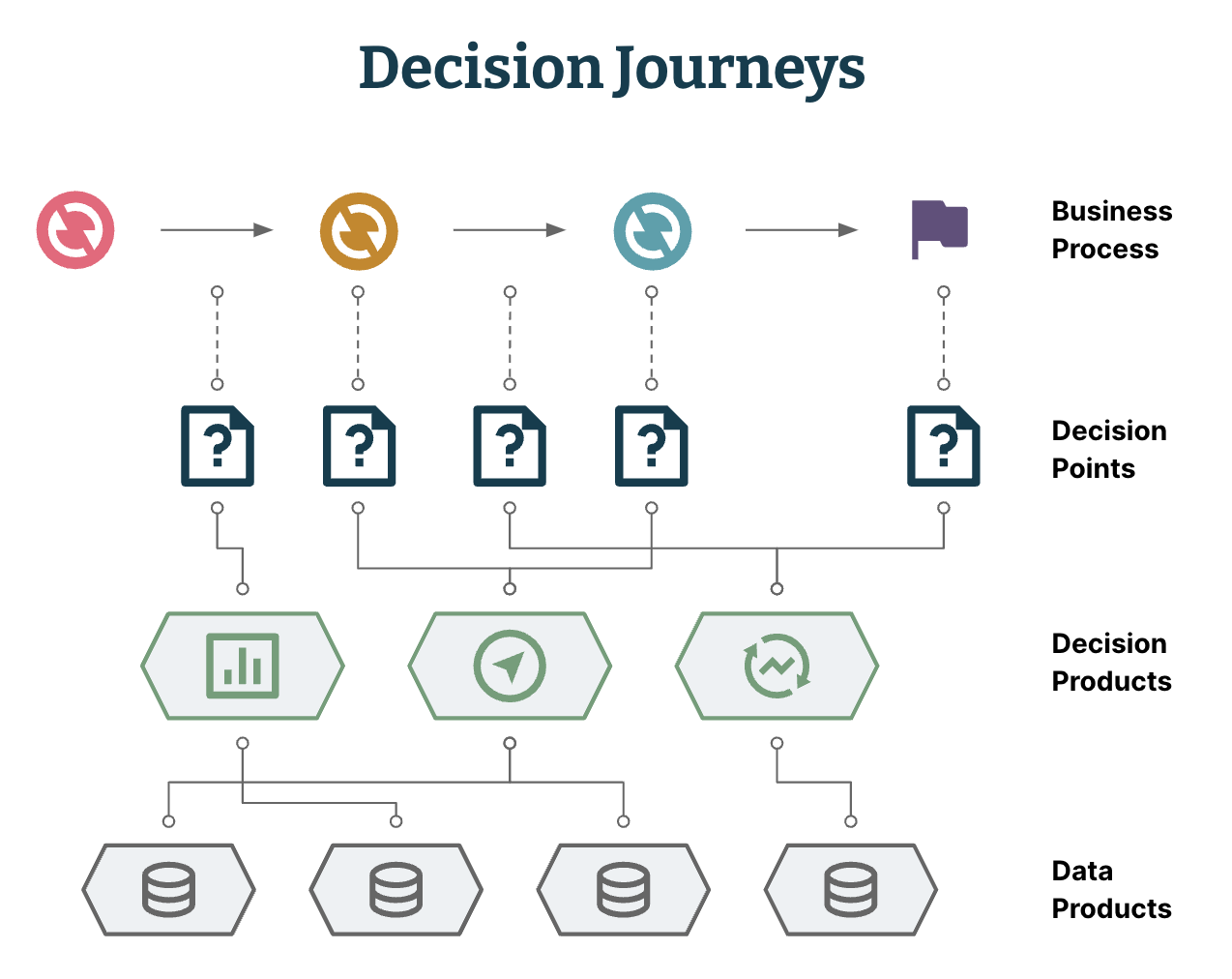
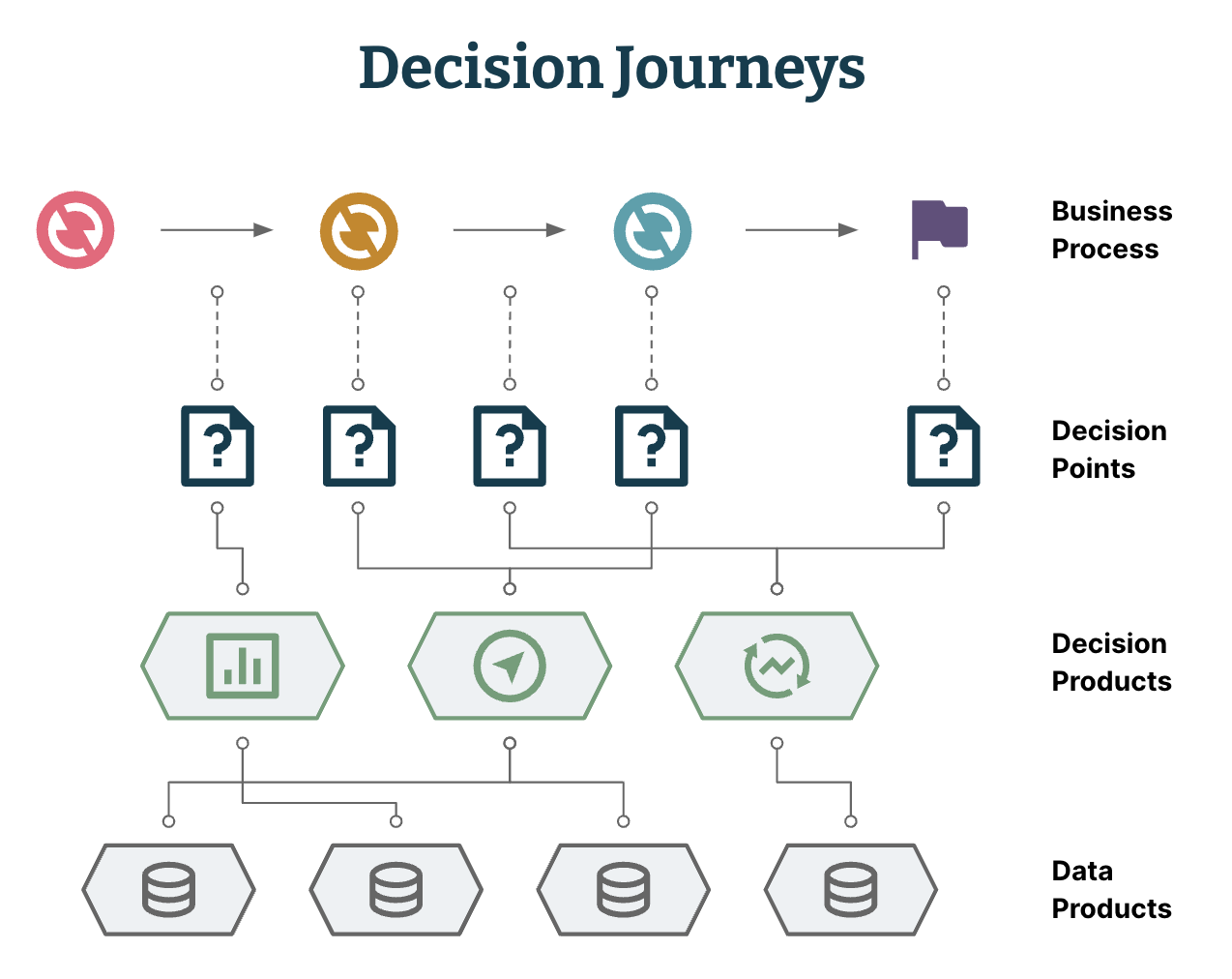
By viewing all these components together, an organization can build decision products for each decision point and connect them to provide an overall goal across the entire process. This means not only sharing insights from each decision product across the process, but ensuring the impact is explored and accounted for at a global level as well.
As an example, we can look at the decision journey for a sales funnel. The diagram below details how the journey would be built up from each of the four complement parts, and the connections between potential decision products. Focusing on the customer engagement section of the process, a major component would be the dynamic pricing decision product explored above. It can be seen taking in insights about the marketing campaigns and channel attribution from the acquisition section as well as customer personalisation preferences and loyalty analysis from retention. The connections between decision products go further; the dynamic pricing product will also share insights back, enabling the organization to understand relationships between pricing fluctuations and loyalty or preferences, and how that affects the overall customer experience.
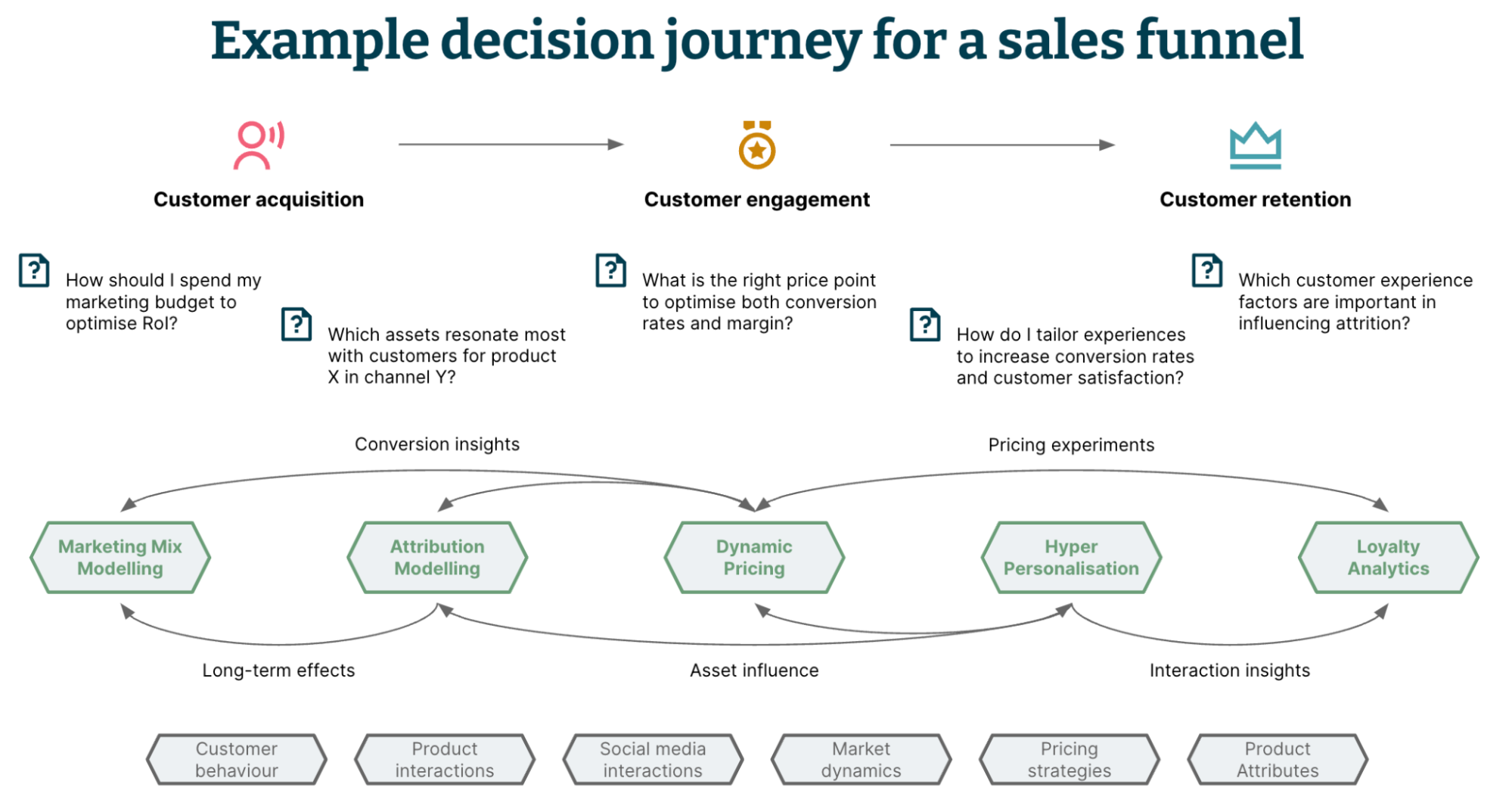
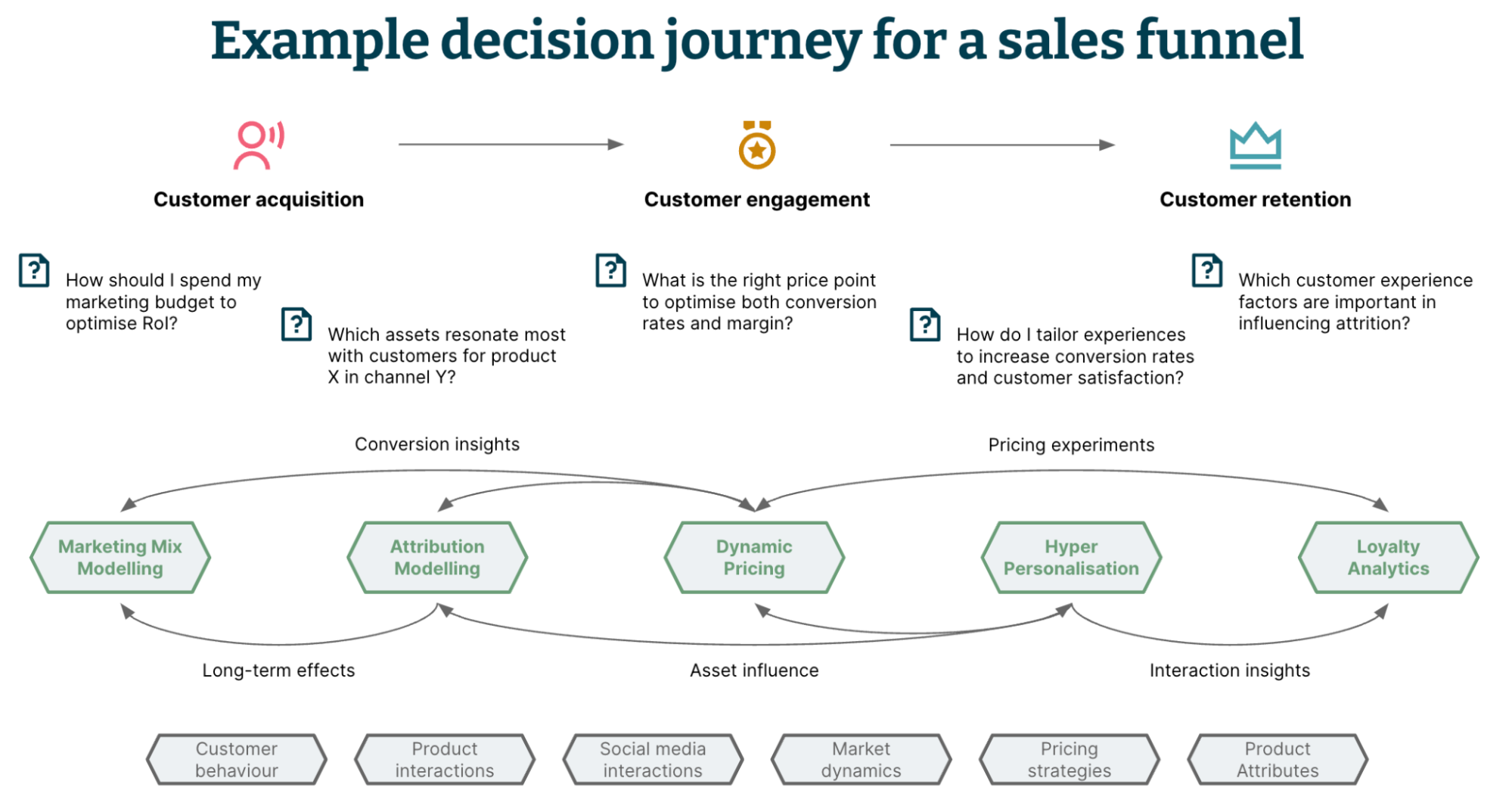
The decision platform
As outlines above, businesses don’t consist of just a single process. They are made up of multiple different, interconnected value streams, all of which work together to deliver the overall goal of the organization. In an intelligence-driven enterprise, each of these streams would have an associated decision journey, sharing insights, and potential decision products, with other journeys. The example sales funnel journey from above would be connected to the manufacturing journey to share demand estimates, the product development journey to support consumer trend predictions or the finance journey to optimize cash flow. This ecosystem of interconnected value streams empowers the generation of multi-scale digital value across the whole business, ensuring resilience and ultimately driving growth.
Achieving this level of connected decision making at scale requires a strategic platform capability; the decision platform. The decision platform should create an ecosystem of technology and business capabilities that enable business domains to experiment with, develop and deploy decision products and journeys with minimal friction. Using approaches such as data mesh and CD4ML,the decision platform enables the creation and management of high quality and self-service data and decision products at scale. It combines modern data platform and ML platform capabilities to operationalize decision products consistently. This means every team can test new ideas and validate learnings easily, paving the way to numerous business benefits such as continuous innovation, agile decision making, insight democratization to name just a few. It’s the ease at which groundbreaking - and often challenging - visions and strategies can be executed and learnt from that defines an intelligence-driven business.
Many organizations will have a significant history of technology investments, resulting in years of built-up technical and architectural debt. This makes delivering such a platform painful and often results in efforts collapsing. Leaders should make extensive use of platforms thinking to accelerate and de-risk the initiative, tying it in with the EDGE operating model and picking decision products and journeys as initiatives to deliver thin slices of capability around.
Transformative change at this scale requires leaders to be able to invest and deliver incrementally. This is the true power of the three perspectives - breaking down the complexities of an enterprise-wide intelligence-driven decision-making capability into deliverable, meaningful initiatives. Each contributing to better, faster decision-making at scale:
The decision product amplifies human and machine decision-making skills
The decision journey removes silos and optimizes decisions across a process
The decision platform enables autonomous, frictionless decision innovations and supports multiple decision journeys at scale
Only by employing these three perspectives can leaders define the building blocks their organization needs to truly survive and thrive in the modern business environment. Winning in this context requires the holistic approach to delivering connected decision making at scale - ultimately unlocking the intelligence-driven enterprise.