Business interest in artificial intelligence (AI) has rocketed in recent years — spending could reach $15.7 trillion by 2030, according to PwC. But there remain lingering concerns that businesses are failing to realize the full value from their investments.
Ever since their emergence, AI, machine learning (ML), and data science have all been surrounded by hype. We’ve been promised technology that will solve our most complex challenges for us and automatically optimize everything from internal processes to customer experiences.
Advances are being made every day that promise to transform virtually every aspect of our lives. But, for most businesses, the reality of the AI and ML use cases they’re deploying looks very different. They’re delivering business value, but they’re typically far narrower, and more targeted in their scope than the headline examples that shape how the public perceives AI.
The AI and ML applications we’re seeing businesses deploy — especially those available in the mass market — are frequently simple automation solutions, turning routine tasks or simple processes into automated workflows.
That’s not necessarily a bad thing, as automation has a role to play in the operation and optimization of almost every business process. But there’s a very big difference between the automation of tasks and the augmentation of human intelligence that many organizations want to achieve.
If business leaders want to realize AI’s full potential and bring truly game-changing use cases that augment human skills to life, a fresh approach to such projects can help: one that emphasizes breaking out of the perpetual hype cycle, asking different questions of their data and capabilities, and challenging supposed ‘best practice’.
Here are three pieces of advice, learned from successful ML projects at industry-leading clients, that can help businesses of all sizes reframe how they think about machine learning, develop higher-value use cases, and see stronger results.
Tip #1: Start with a clear business problem, not data or technology
One of the most common mistakes businesses make with ML projects is using their data or their available capabilities as their starting point, rather than starting with a specific problem they want to solve, and working back to the capabilities required.
On the surface, that seems to make sense. The most sophisticated, high-impact ML models are invariably powered by the biggest and most complete training datasets. But, in those cases, we’re typically talking about colossal, purpose-built, curated datasets, crafted for extremely data-intensive use cases. For the vast majority of businesses, the logic of ‘apply ML where you have the most data to get the best results’ doesn’t hold up at smaller scales.
In reality, it doesn’t take a huge amount of data to solve the kinds of challenges that most businesses want to tackle with AI or ML. In our experience, the most successful projects are built around a specific business challenge, regardless of the volume of available data related to that challenge.
If you use data as your starting point and dive in where you have the most available data, you’re often left with models and capabilities that, while potentially sophisticated, don’t help you solve any immediate business problems. And when you’re not solving real problems, you’re not realizing value — a big reason why so many organizations that experiment with ML retire their projects without seeing a clear return on them.
Creating an optimal airport with Finavia
A project we recently undertook with Finavia — operator of Kittilä airport in Lapland — demonstrates the power and value of starting out with a clearly defined problem you want to solve.
Kittilä is a small airport, with just 12 plane parking spots. For most of the year, traffic through the airport is low. However, around Christmas, demand surges, with as many as 58 flights arriving and departing on the busiest days, 70-80% of which arrive within a four hour period.
With demand and traffic so low outside of those short, huge demand spikes, simply adding resources and capacity isn’t an option. So, Finavia spoke to us to help them optimize how demand spikes are handled using a combination of optimization tools and machine learning.
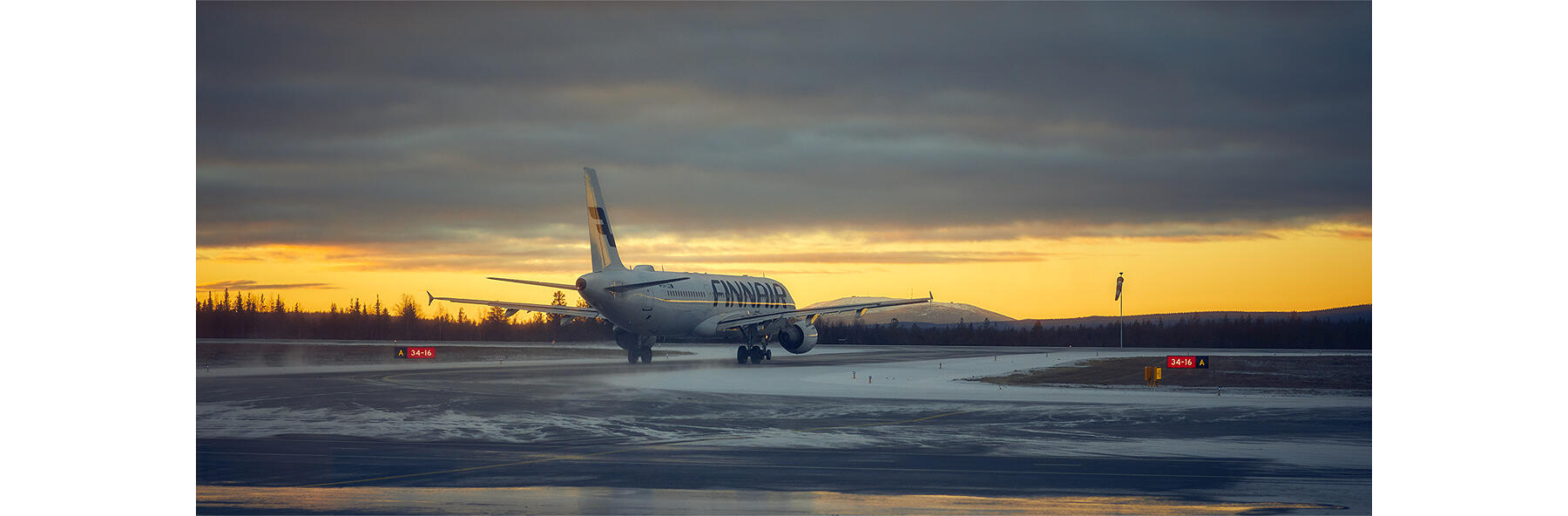
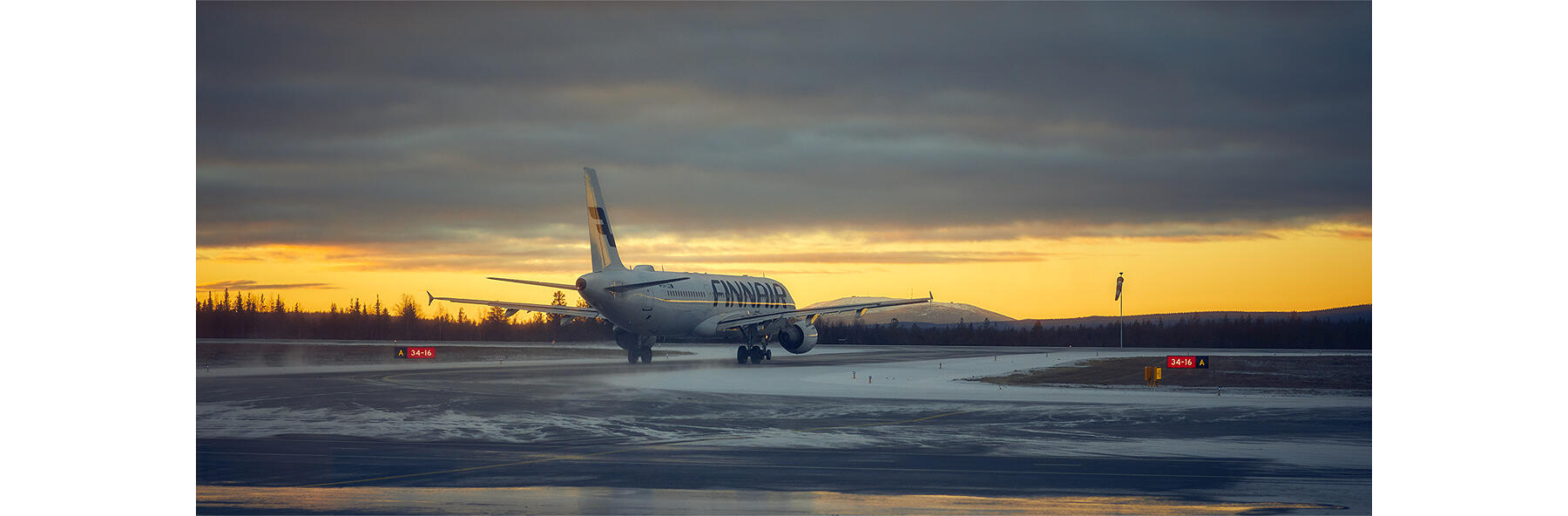
Together, we created an optimization model that uses current and historical flight data to build mathematically optimized parking plans that account for all relevant rules, such as not all aircraft types being suitable for every parking spot. The solution also uses machine learning to predict arrival times and passenger numbers, which are used to optimize resource allocation inside the airport, ensuring that every passenger has a great experience, and can pass through Kittilä quickly and conveniently.
The results have been impressive: Finavia increased the number of flights at Kittilä Airport by 12% in the year following deployment and reduced average airport delays by two-thirds — which resulted in roughly €500,000 in cost savings.
The optimization model brings together data sets that may not have ever been combined otherwise to solve what is undoubtedly the biggest business challenge faced by the airport. If Finavia’s ML journey didn’t begin with a clear mission to solve that problem, it’s the kind of use case that would have been very unlikely to ever materialize.
Tip #2: Use AI to augment human intelligence
The headline outcomes of AI aren’t purely driven by technology — they’re driven by people empowered with that technology.
Even though we associate them with autonomy, both technologies are at their most valuable when they’re applied within human teams and those teams are given the competencies to get the most from them.
It’s important to recognize that artificial intelligence isn’t a replacement for human intelligence, it’s an augmenter of human intelligence.
It’s important to recognize that artificial intelligence isn’t a replacement for human intelligence, it’s an augmenter of human intelligence.
Humans and machines are both very good at very different tasks. While machines are great at sifting through and extracting meaning from massive quantities of data, humans remain consistently better at contextualizing the insights generated by AI models.
They’re better together, and if you want to get the most from your AI and ML investments, that needs to be reflected in your implementation strategy. That means providing relevant training for teams, preparing them for how new AI capabilities will change their working patterns, and evolving processes in ways that suit team members.
Your focus should be on enabling people to use AI capabilities in ways that complement their working patterns and support how they operate, rather than dropping new capabilities on your teams and making them adapt the way they work.
Human and artificial intelligence join forces to create an award-winning whisky
A perfect example of effective human-machine collaboration is a project we recently undertook with Swedish whisky distillery Mackmyra to celebrate its 20th anniversary.
Between different water sources, ingredients, aging processes, and cask types, there are an almost limitless number of combinations of factors that can influence a whisky’s flavor profile. Master distillers can spend their entire lives tasting and tweaking options to create the perfect blend. That’s where Mackmyra wanted to employ the help of machine learning.
First, we built an ML model that used previous recipe data, tasting notes, reviews, and cask information to understand what makes an iconic Mackmyra whisky. Then, we created a proprietary generator-discriminator model that explored new spaces and generated unique recipes that were entirely new, but distinctly Mackmyra.
The recipes generated by the model were then evaluated by a master distiller, bringing the all-important human context and understanding to the process. The master distiller’s final selection then became Intelligens — an ML-designed whisky that has won awards including the American Distilling Institute's Gold Label and Best International Malt Whisky trophies and the world-renowned ADC Silver Cube in Product Design.
Tip #3: View AI as augmentors of your team
As augmenters of human capabilities and intelligence, AI models are functional extensions of your team — and they need to be designed as such.
If your models and use cases are designed in isolation, rather than to align with specific requirements of the teams that will use them, those teams are likely to reject and avoid them.
During the design process, organizations often fail to prioritize important success factors such as ease of use, transparency, and how easy it is for humans to translate the outputs of an AI model into valuable actions. When those factors aren’t considered, the models ultimately fail to deliver their intended value.
This is an area where the teams that design and deploy ML use cases can learn some valuable lessons from HR. In many ways, high-value ML models or AI use cases are like the perfect new hire. Both should:
Integrate well with the team and be easy to engage with
Offer fresh perspectives and capabilities, without being too disruptive to your current processes, goals, or strategic priorities
Solve a specific need, doing something the business doesn’t have the capacity or capability to achieve otherwise
Have a clear roadmap for growth, with a transparent view of what you’d like them to achieve over multiple years
Support and augment the skills already in place in a team, maximizing their effectiveness
Helping innovation flow with AI-enabled R&D
We’ve recently helped several organizations successfully augment their research and development capabilities with AI. It’s a function that AI is very well suited to, largely because it’s very easy for AI to function as an extension of an R&D team because of the ingrained culture of experimentation and learning.
In these businesses, AI isn’t fundamentally transforming R&D processes, it’s simply delivering supporting capabilities that human team members have wanted for years. From crawling through test data to quickly surface the causes of failures, to identifying trends in huge volumes of product data, it’s accelerating processes and giving humans more time to focus on bringing innovative ideas to market faster.
Making AI work for your business
Too often, AI projects start with the misconceived notion that you start by using data to build some innovative tech. In fact, what’s required is a solid understanding of the business challenge you’re trying to optimize — from there, you can build in the human factors that will impact your chances of success; identify what data you have available and the areas where AI tools can augment human smarts.