Imagine a world where devices with a micro footprint have a macro impact. Where compute is decentralized, power-efficient, sustainable and affordable – and has data privacy built-in. Move over big data, machine learning and cloud computing: welcome to the world of TinyML.
Operating at the intersection of edge computing and the cloud, this tiny but mighty technology is here to open up new possibilities for the next generation of machine learning-enabled technology.
The tiny tech with enormous potential
Many people have never heard of Tiny Machine Learning (TinyML). Yet chances are, you’re already using it every day. If you’ve ever used a voice assistant like Siri or Alexa, you have experienced the potential of a tiny piece of machine learning to do one thing very well.
With no constraints, the power of machine learning is almost infinite. But it is also centralized, relies on large models and requires significant power and infinite data. Model sizes keep growing, with billions of parameters. And that complexity comes at a cost — including a growing cloud carbon footprint.
Cloud-based ML is the technology equivalent of a big picture thinker, with broad capabilities. TinyML is the opposite. It focuses on solving small problems that can open up new possibilities across all tech sectors. It is decentralized, which protects data privacy at the source, and light enough on power to run on a battery. It can also be tested and deployed very quickly.
TinyML is the specialist ML, with a laser-like focus. And there are already 3 billion devices that are able to run machine learning models.
For example, voice assistants use machine learning to process language, drawing on vast sources of data in the cloud. But to make sure it’s not always on, something needs to kickstart the process. This is where TinyML comes in. It’s trained to listen for ‘wake words’ such as ‘Hey Siri’ or ‘Alexa’, which then triggers machine learning to process sound into text – and put on your favourite playlist or order your groceries.
The benefits of TinyML
One of the main points of difference between TinyML and other machine learning paradigms is that it can work offline. Its models run on small, low-powered devices like microcontrollers, giving devices and sensors a new level of intelligence without requiring an internet connection. So you could conceivably run TinyML models at the bottom of the Pacific Ocean or on top of Mount Everest — or in remote communities beyond the reach of 5G.
As TinyML is highly decentralized and so close to the data source, processing can be lightning-fast. Low latency supports more immersive experiences in devices that rely on gesture recognition such as gaming and augmented and virtual reality applications.
By processing raw data locally on the device, TinyML systems can improve data security by only transmitting processed information to external systems. This can be very important in certain applications with highly sensitive information, such as sharing healthcare records.
By its very nature, TinyML devices are small and don’t require a large amount of power, allowing it to run on batteries. In many cases, the model size is also very small – for example, ‘wake word’ detection capabilities can work in a model as small as 13 kilobytes in size. That’s about the same size as a low-res profile photo.
By operating at the intersection of physical and digital worlds, TinyML allows us to measure, analyze and make decisions — powering rapid data computation on the go, making the promise of ML more affordable, accessible and widely available.
The challenges of TinyML
Machine learning on billions of low-cost, low-power devices may sound exciting — however, there are a few complexities to consider.
Although the models are small, they are still prone to common machine learning challenges. It’s important to consider the ethical and responsible use of technology, and standard approaches to avoid model bias still apply. Model explainability remains challenging, and training pipelines will become more complex with federated learning and cross-compiling across models.
In addition, decentralized and disconnected devices may never be connected to the internet; this adds complexity when it comes to managing software and model updates. Engineers must ensure their models are the best they can be before they are permanently burnt into the reduced-order models (ROMs) of a device and shipped around the globe. General Motors’ (GM) Road to Lab to Math (RLM) strategy, over 15 years old now, provides some inspiration for dealing with this challenge. By moving more of the development upstream in the process, GM shifted testing from “road to lab to math”, so designs could be tested faster and at a lower cost. In a machine learning context, this means shifting the need for short feedback cycles earlier in the development process. It forces teams to be creative in how they apply MLOps principles around testing and evolving before the design gets locked in.
TinyML's future is full of potential – but as with any new technology, we must be careful with how we use it.
TinyML in practice
While TinyML won’t singlehandedly solve the world’s biggest problems, its potential to improve the way we deploy future technology is exciting, especially when we combine single TinyML components that do one job well to create incredibly capable systems. This is what’s known as Composable AI — bringing edge and cloud computing together in a flexible, easy to deploy and extremely scalable system.
Vehicles are one example where low latency and offline accessibility really matter. In the near future, Advanced Driver Assistance Systems will consist of many small, low-cost sensors that leverage TinyML to ensure cars are safe, while cloud-based ML will provide navigation and user experience.
In agriculture, meanwhile, TinyML could also enable low-cost, low-power devices that could help detect the location of weeds or measure the quality of fruit and vegetables in real time. In manufacturing, it could help with quality control; it’s already helping ensure equipment runs smoothly using motion detection.
You don’t have to change the world — start with fish and berries
At a recent XConf talk, I shared my own experience of experimenting with TinyML. During the COVID lockdown, I built an aquaponics system. This is an ecosystem where fish waste gets turned into nitrogen to help plants grow, while also cleaning the water.
While the system worked well, the pump occasionally got blocked. When it was working properly, you could hear a gushing sound every 10 minutes. While I had never used TinyML before, I thought this would be a great opportunity to start by building a system that could detect sound — the tool could highlight that the pump wasn’t working when no sound was detected. I first deployed it onto my phone to test it, then, once I could see it was working, onto a microcontroller. The entire process took two hours and cost around $30 — and it worked. My fish always have clean water and our strawberries are thriving.
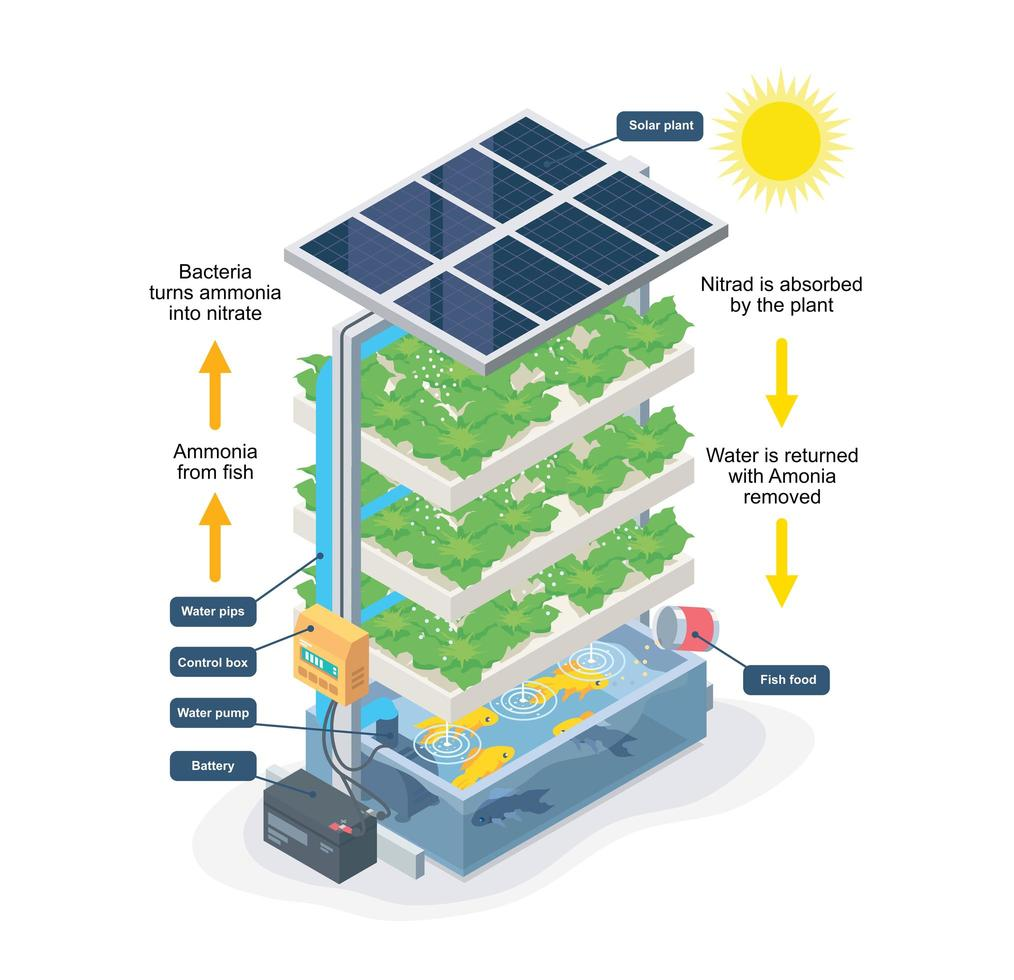
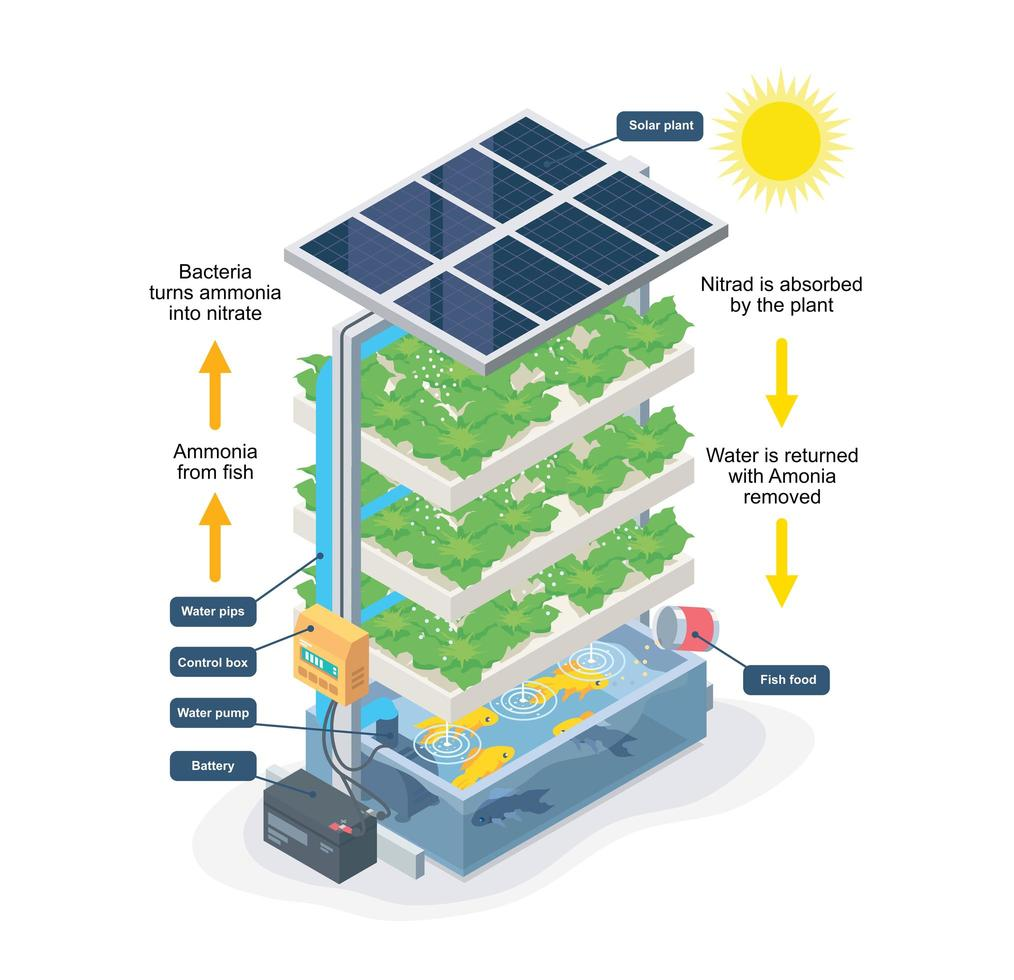
Aquaponic system smart farming isometric by AllahFoto. Image source: Adobe Stock
Sure, that’s just one small problem solved, but imagine the potential to use TinyML to solve greater challenges — such as using fine-grained data to detect the imminent risk of flood or bushfire.
You don’t have to be a hardware engineer or an embedded systems developer to get started with TinyML. It can be fast, easy and cost-effective to unlock efficiency or improve how something works. Free, simple tools are already available, including Edge Impulse, to help you design, test and deploy the technology fast.
Get creative with TinyML
It’s easy to get distracted by the potentially limitless world of machine learning. But when we have limitations and constraints — like model size and hardware —it forces us to think differently about how we would approach a problem.
Think about constraints as creative opportunities. Try pushing things down to devices as much as possible, and teach TinyML to do one thing in a really intelligent way. By combining simple things together, you can create something truly incredible. They don’t even need to be temporary or short term innovations: we can now use internet-free microcontrollers and sensors with enough battery power to run computer vision models and audio processing algorithms for ten years.
That’s what will help us build the next generation of devices and technology. Small yet mighty, TinyML will provide affordable, accessible, secure and seamless experiences – with a lighter footprint.
To learn more about TinyML, read Thoughtworks Technology Radar.
Disclaimer: The statements and opinions expressed in this article are those of the author(s) and do not necessarily reflect the positions of Thoughtworks.