Finance is at an analytical crossroad
It was a typical workday, in a typical conference room of a typical bank head office. “How many unique customers do we have, across our bank’s products in this country?” asked the country business manager, at Global Consumer Bank. He thought that was an easy question and that the data science head would have the answer at his fingertips. Attending the meeting were business leaders: the managing director, the country head, the retail banking head, credit cards and personal loans head, home loans head, and the senior country operating officer. Over the next few minutes, a few numbers were tossed around as potential answers.
It soon became apparent that there was no consistent number to be found or that could be agreed upon.
This bank had an elaborate data warehouse. Even though the financial institution’s data warehouse had data such as global customer identification numbers (GCIN), household numbers (HH number), customer identification numbers (CIF number) and account numbers, the leadership was still unable to arrive at the number of unique customers across products. The main pain was: where was the customer data ownership in the business? Why can’t the data platform teams take ownership? Does anyone own this problem domain? The first step in solving any problem is to ask the right question. That’s it: Does anyone own this problem domain?
No? So how did we get here?
The data backbone of most financial institutions are decades’ old. They’re now conglomerations of the oldest bank plus all the mergers and acquisitions that have occurred over time. It is highly likely that the smaller entities brought with them a banking system with different formats for their customers. We don’t just mean the systems, but management philosophies as well. The banking systems were aggregated side by side into the larger corporation. They may be on the same network, but their data is as isolated as two trees in a forest.
To understand this situation, we need to look at the real-life challenges faced by most financial institutions — things that keep them occupied and prevent them from working on fundamental and strategic issues:
Data identification authentication. Your analysis staff has multiple, self contained systems that use different names for customer IDs. This is persistent friction in your organization, a hidden factory of constant cross checks and filtering, every time you need a customer acquisition strategy developed, a customer revenue or margin analysis report, or a customer 360 program launched.
For example, accuracy of the statistics in a report depends primarily on the data selected and its quality. When the selected data are not found across these self contained systems, there is a tendency to substitute surrogate data. This is what affects the report’s accuracy.
Multi-level approval for data. Hierarchical and archaic, many traditional banks still operate using the 70s version of the analytic management philosophy, sans the sensitivity training. Peter Drucker has not won yet. Banking is very dynamic - so why when new data is created due to process or product additions, does it require multiple levels of approvals from various departments in the financial institution? Think of the friction and time to market delays this causes.
By using data across business units and product lines such as credit cards, across retail banking and private banking, a financial institution is able to target client needs more efficiently and proactively. Unfortunately, currently this can require many time consuming approvals from business leaders. Why? For example - during a cross sell program, the approval process is used to try and clear up inconsistencies and different approaches to how revenue would get shared across the business units when a product is sold.
Offline data. Highly paid, limited quantity, specialized analysts in your organization are spending an inordinate amount of time on cleansing, organizing and aggregating data in a manual manner that should be automated. It is almost as painful as balancing your checkbook by going to a local bank branch to have the branch manager fill out your check register. When your analytical timelines are being busted on an ongoing basis, you can bet that your team is performing manual data housekeeping instead of analyzing.
Third party data is used to enhance organisation data and make it more complete for analyses. For example - identifying which areas to target while running a campaign for acquiring High Net worth Individuals for wealth management in Tier II or Tier 3 cities. Third party research data is accessed to identify market size and to help arrive at a size & share of wallet of potential clients. However, this data arrives in completely different formats, with different nomenclature and definitions. Standardizing and translating data into the organisation’s formats is a resource intensive task.
Organizational challenges. Does the analytical group know what data they own and control? Are there any groups that own domain data? For example, is the customer profile information owned by one group of people who manage its content, security and access? If not, that means no one might — and that causes all manner of interdependence and intra-communication between multiple departments and sections within the organization. A spaghetti bowl of communication with possibly no one authority.
Owners of data lead to better completeness and effective usage of data. When owners are not identified, maintaining, documenting, updating, and ensuring completeness is ineffective. For example: a bank’s onboarding operations team reviews a new corporate account form and keys in the details into the core banking system. If over time there have been changes to the corporate client’s demographic details (they moved, new CEO), who in the organisation will be responsible to go back to the client and get the data updated? For an active client, this would be easier managed during validation interactions for a transaction. However, we know there is a huge percentage of customers who are passive or some who are inactive. Updating customer details on a periodic basis will be possible if Data owners are identified.
A deeper analysis typically shows that most of the issues above result from the lack of a business dictionary or glossary. A business glossary is an essential asset produced by an organization during data governance development work. The glossary is a technical and social agreed-upon understanding of key business concepts, business terms and the relationships between them in a domain. It in fact is a key piece in a bank’s business architecture.
In our bank domain example, an excellent reference is an ontology called the Financial Industry Business Ontology (FIBO). FIBO is a conceptual model of the financial industry that has been developed by the Enterprise Data Management Council (EDMC). It identifies objects that are of interest in financial business applications and the ways that those things can relate to one another.
By introducing a FIBO domain glossary, data inconsistencies can be identified and multi-level approvals for data can be avoided, as the data items are preordained in the glossary. Offline data processing can be automated due to a common target data set. Lastly, organizational challenges are minimized by assigning ownership to objects and terms that are introduced in a glossary.
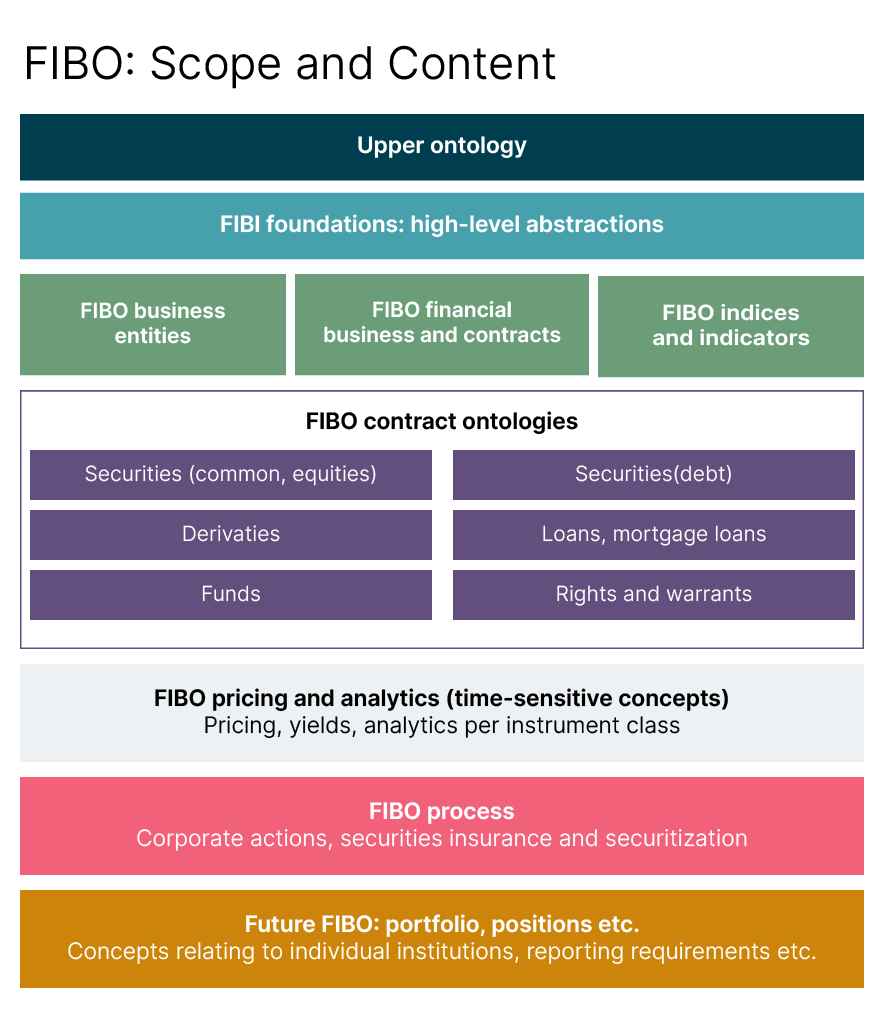
The Financial Industry Business Ontology is organized in a hierarchical structure of financial terms and concepts that are of interest in financial business applications, along with the ways that those concepts can relate to one another.
The top-level directories are called domains, under which you can find business entities, financial and business contracts, along with indices and indicators.
Sub-domains, which include FIBO contract ontologies may include , loans, mortgages, securities and the like.
Below that comes priciing, analytics and yields.
A standardized business glossary is certainly a first crucial step for improving analytics outcomes of financial institutions. But in order to truly accelerate time to value and harness the power of a common data glossary, we must think beyond the current implementation technology of data systems. These are systems and software that use centralized data lakes and warehouses (on premise or in clouds). We must invest in automation and governance: the Data Mesh.
The Data Mesh
At another bank we worked with, ownership of data domains was dispersed and there was no central glossary of terms. They had multiple generations of customer data systems and they used cloud technology. When we finished our first implementation of their Data Mesh, the time to value delivery of analytical business “data products” went from three up to 100 in a short period of time, with future ownership identified and a business glossary governing all the data products.
Notice the change in term from data domain (e.g. customers) to data products. This is also a crucial step, and is responsible for identification of ownership and time to value speed. Simply, treat data consumers as customers. A team creates the data product or data set for a specific customer’s needs. This team also owns the data domain and can produce data products for any other consumer.
Time to value acceleration comes specifically from the mandatory combination of ownership, data automation tools, and a common business glossary so vendor-consumer understanding is fast.
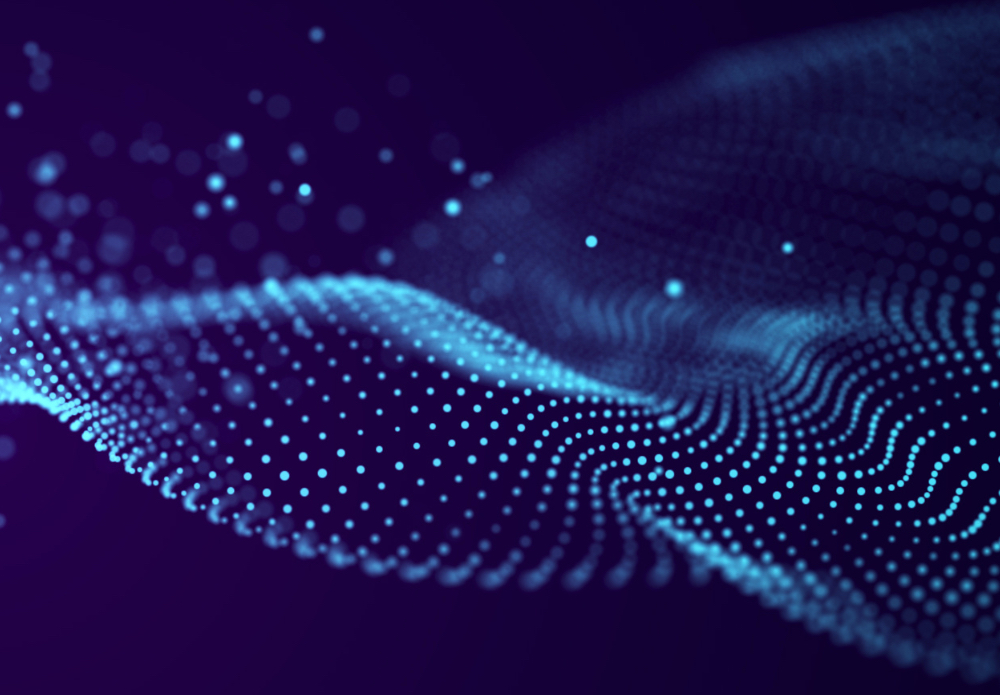
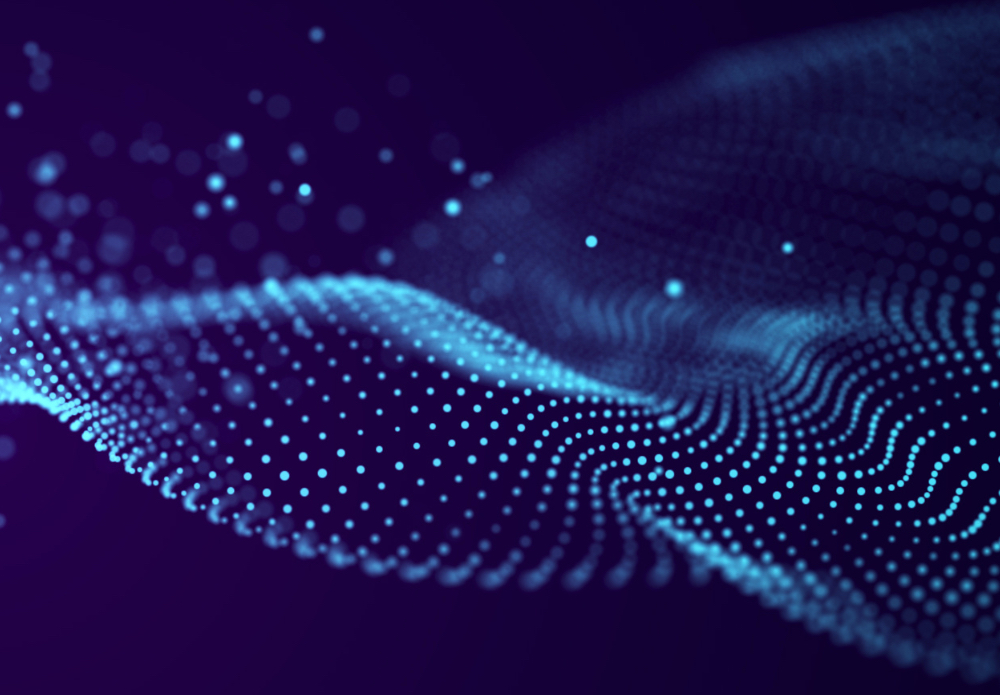
To understand a Data Mesh, change your current mental model from a single, centralized data lake, to an ecosystem of data products that play nicely together. Instead of handcrafting a data lake for everyone, you gather domain-specific users together and ascertain their smaller group needs. Instead of handcrafting bulk data loads, you create an automation pipeline reading from related data sources to produce a data product for that group. Instead of handcrafting data export code, you use a language to auto-generate the data pipeline, to produce the data product, ASAP. These three elements — a domain-specific user group, a generated, automated pipeline, and a data product for that user group — create a node on your Data Mesh.
Data Mesh-oriented cloud development uses well-known data infrastructure tooling (e.g. Kubernetes and Terraform) and is much faster than data lake and warehouse solution development. In our experience, you reduce the time it takes to get valuable insights from quarters and months, to weeks.
For those in the financial industry, this type of approach can support process and operational improvements that result in better prediction of customer outcomes.
When data ownership is clear, accountability increases, leading to a higher quality of data. As one frequently updates the Business Dictionary / Glossary, it standardises the nomenclature across the organisation.
Data product oriented domain teams will drive data usage across the enterprise for campaigns cutting across company product lines. They already have an in-depth understanding of their domain’s data fields and associated relationships with third party data fields. This will encourage and inspire confidence in the rest of the organisation to use third party data authenticated by these data product oriented domain teams.
Now, when a bank executive wants to know how many unique customers there are across products, the response that they will receive will be accurate, reliable, and faster; and most importantly the number will be the same across the organisation.
So, the vital output of a Data Mesh approach is the data product. It serves a business community for one or more business use cases. It is built very quickly — in just days to weeks. The data product is then typically accessed by the consumers that need it — analysts, business intelligence, machine learning applications — and connections can be automatically generated by the Data Mesh tooling. An important metric for Data Mesh time to value improvement is:
The number of data products that produce analytical ROI or business impact, deployed live to customer group(s) per time period. For example, the time to market for creating a business-relevant Data Mesh data set.
Escaping from years of legacy data warehousing architecture is not easy. After you have "changed your current mental model from a single, centralized data lake," how do you proceed? A few good practices for Data Mesh can guide your journey to a better data solution. They are made up of three phases:
Build a platform strategy to identify end-to-end use cases in one or more domains
Perform a data discovery to determine and validate the data sources, based on a glossary, to be read
Iteratively construct the pipeline, operate it, and share data products for your users to consume
While iteratively constructing and delivering, keep these principles of Data Mesh architecture in mind to help ensure your data products are complete:
Distributed domain-driven architecture. Ensure the data products are bounded by a glossary in a domain
Self-serve platform design. The software to create a data product is public — within your security perimeter. Ensure that others can "run and extend" the pipeline to create new data products
Product thinking with data. Ownership is established for the data products
The prevailing wisdom of more data and centralization is better, a.k.a. "a single version of the truth," will continue to be a challenge to building scalable and relevant analytical systems. But this is a wrong-headed approach. Financial data can lead to high ROI and customer 360 analytics, but the assets should be defined and distributed relevant to current use cases, the customers who need the data, and most importantly, in a timely manner, to capture market opportunities as they arise.