Data mesh as an architectural pattern has strong connections to lean theory and practice for managing complexity, responding to change and better serving customer needs. We shared this perspective on data mesh at a recent LAST Conference and provide a brief introduction here.
Data production and its wastes
Modern digital businesses demand analytical data products for intelligence-driven decision making. We consider analytical data products manufactured in an organizational factory. We consider running the factory - delivering timely and well-governed data from producers to consumers on an ongoing basis - and also building (and re-building) the factory - making new connections from data producers to consumers, driven by evolving demand.
The more we can eliminate waste from this factory, the better we can deliver Data and AI initiatives, and the more we can improve business performance and value. Contrarily, waste is often hidden and therefore hard to address in centralized data architectures, though the resultant impacts of cost, lead time, quality and poor outcomes are felt by practitioners and organizations alike. Our lean perspective, however, makes this waste highly visible, and shows clearly how we might remove waste, motivating a decentralized architecture and a lean approach to delivering value through analytical data production.
What is data mesh?
Data mesh is a decentralized approach to data architecture, originally defined by Thoughtworker Zhamak Dehghani. Data mesh is based on four interrelated principles:
Domain-oriented decentralization: data production and data consumption is owned by the teams closest to the data and associated processes, who can connect peer-to-peer.
Data as a product: the data served is deliberately designed to deliver value to the consumer.
Self-service data infrastructure: tools and services to support producers and consumers to build, connect and run data products with affordances for automation and accessibility to enhance the effectiveness of domain teams.
Federated governance: core governance concerns are built into the platform while individual data products support additional governance concerns in their context.
For more resources on data mesh, see the original article, principles & logical architecture, and our previous mini-blog on guiding the evolution of data mesh with fitness functions.
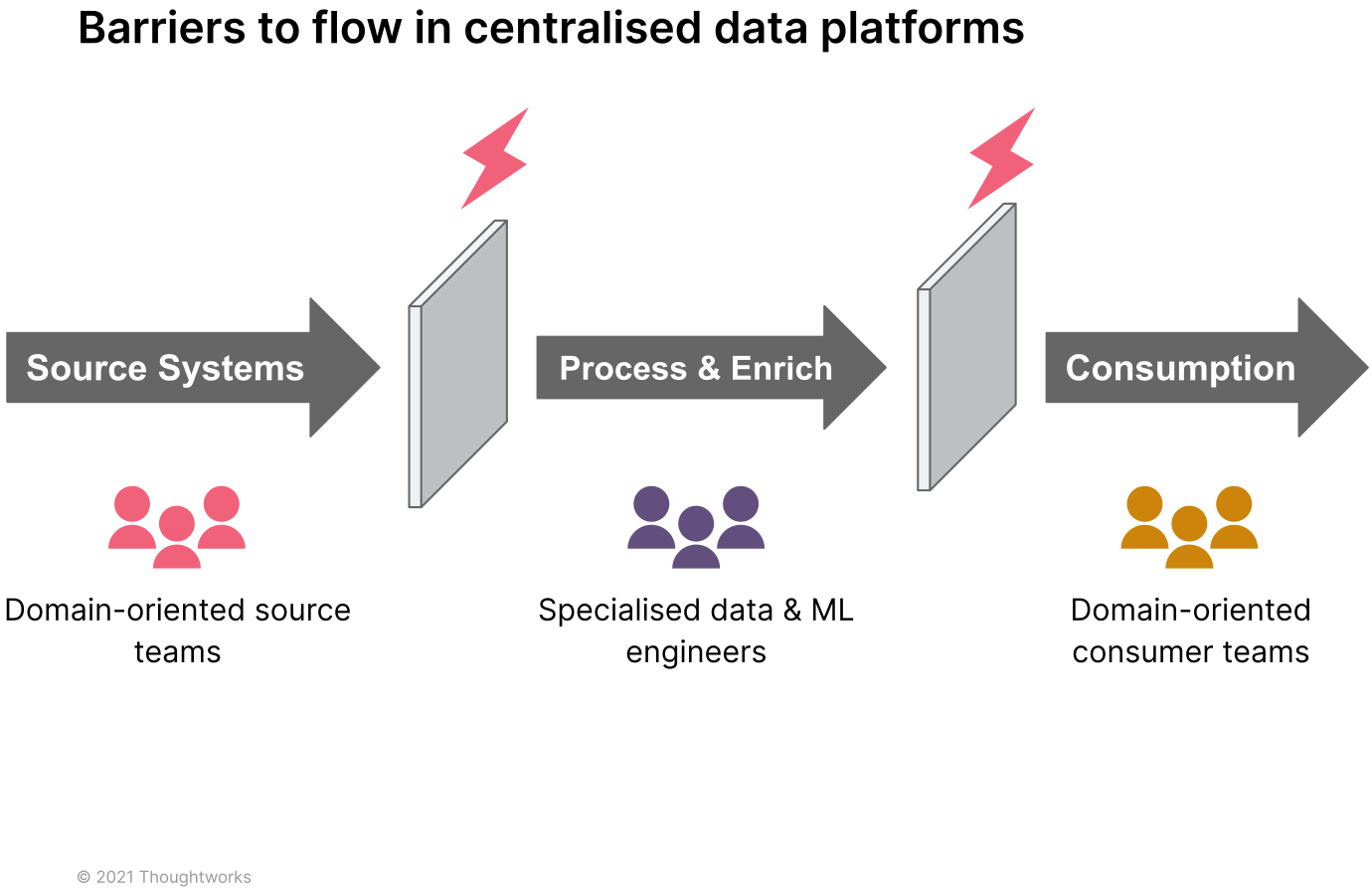
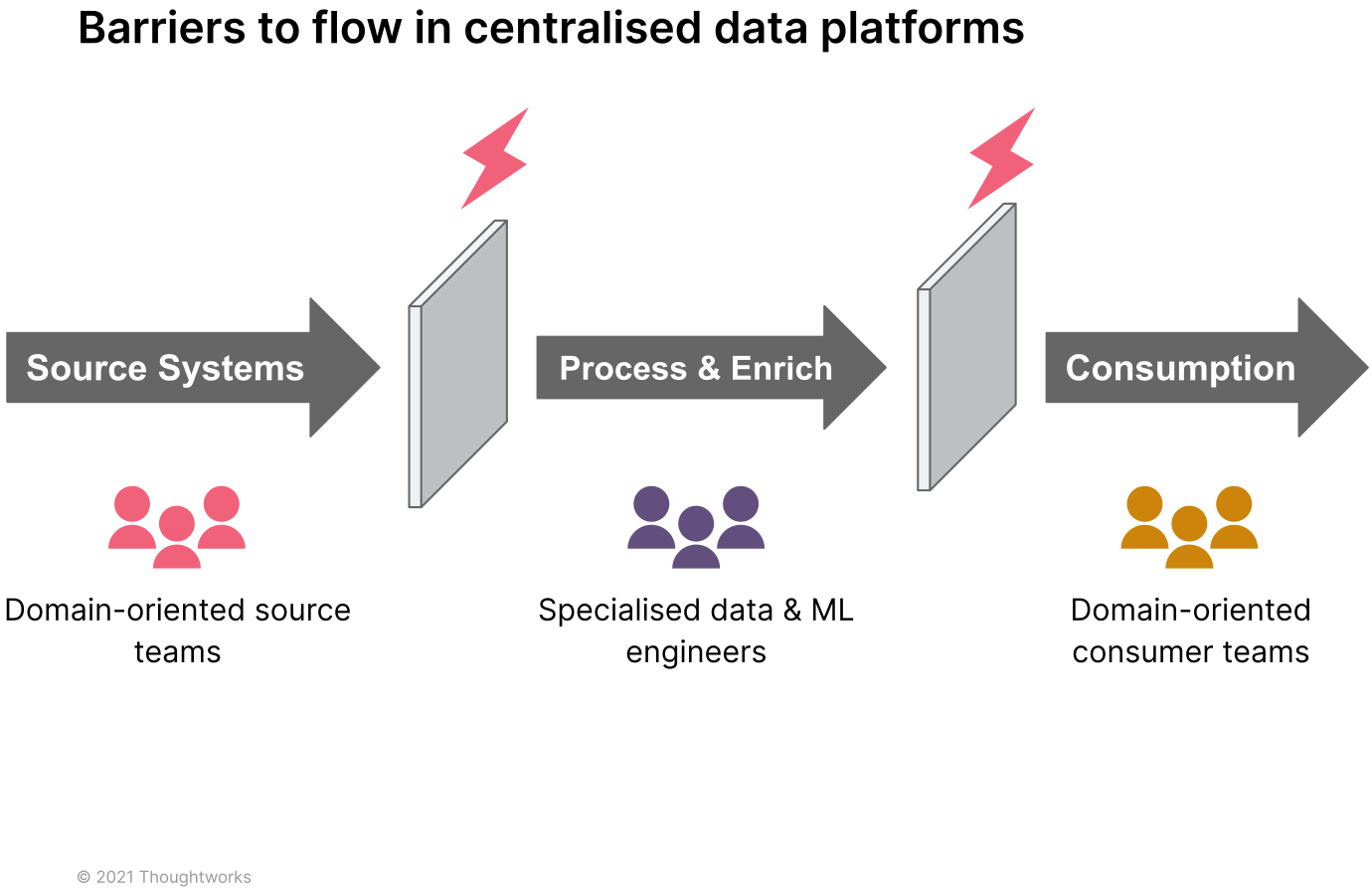
Centralization and technological decomposition leads to batch-and-queue inefficiencies.
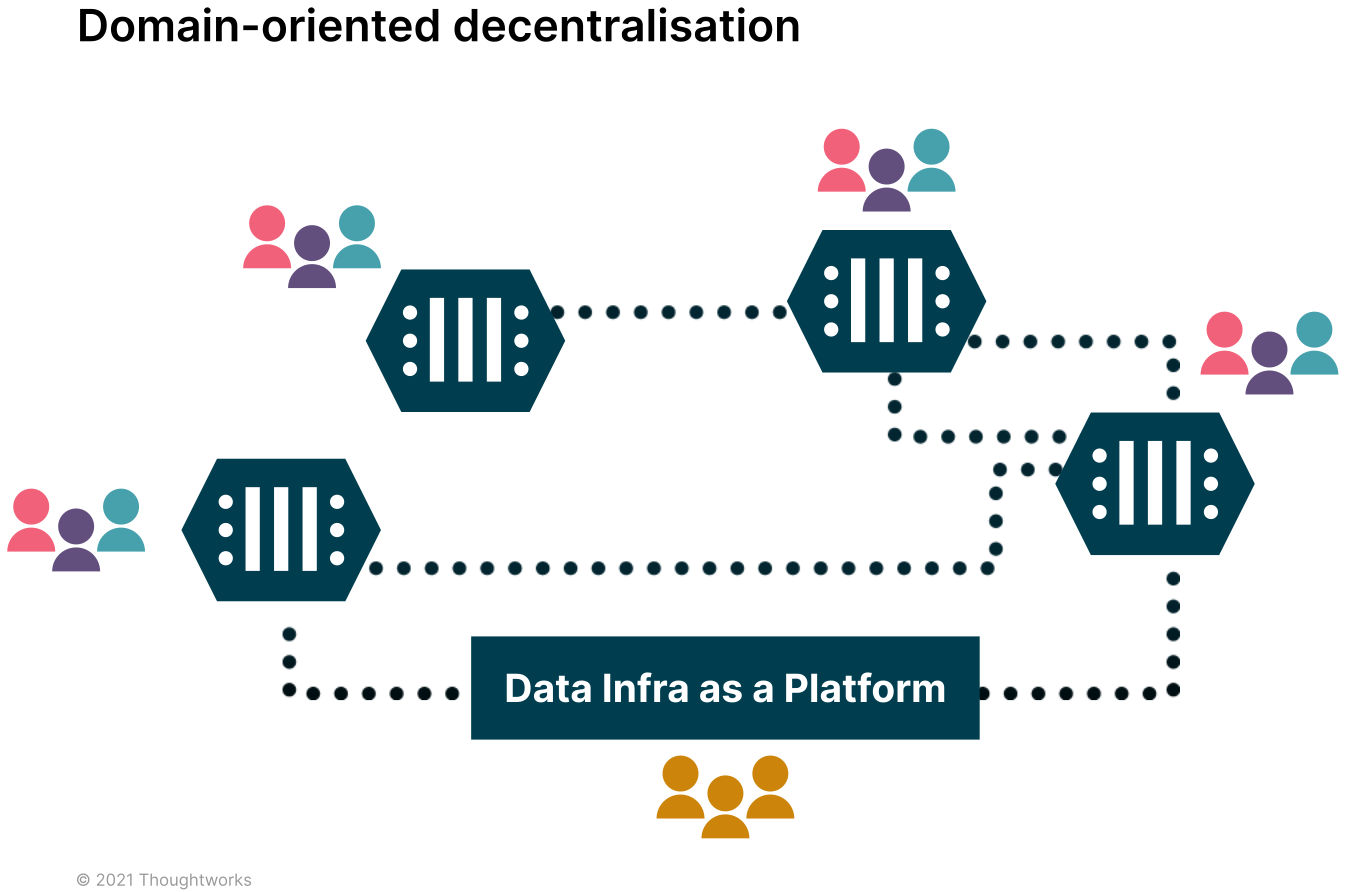
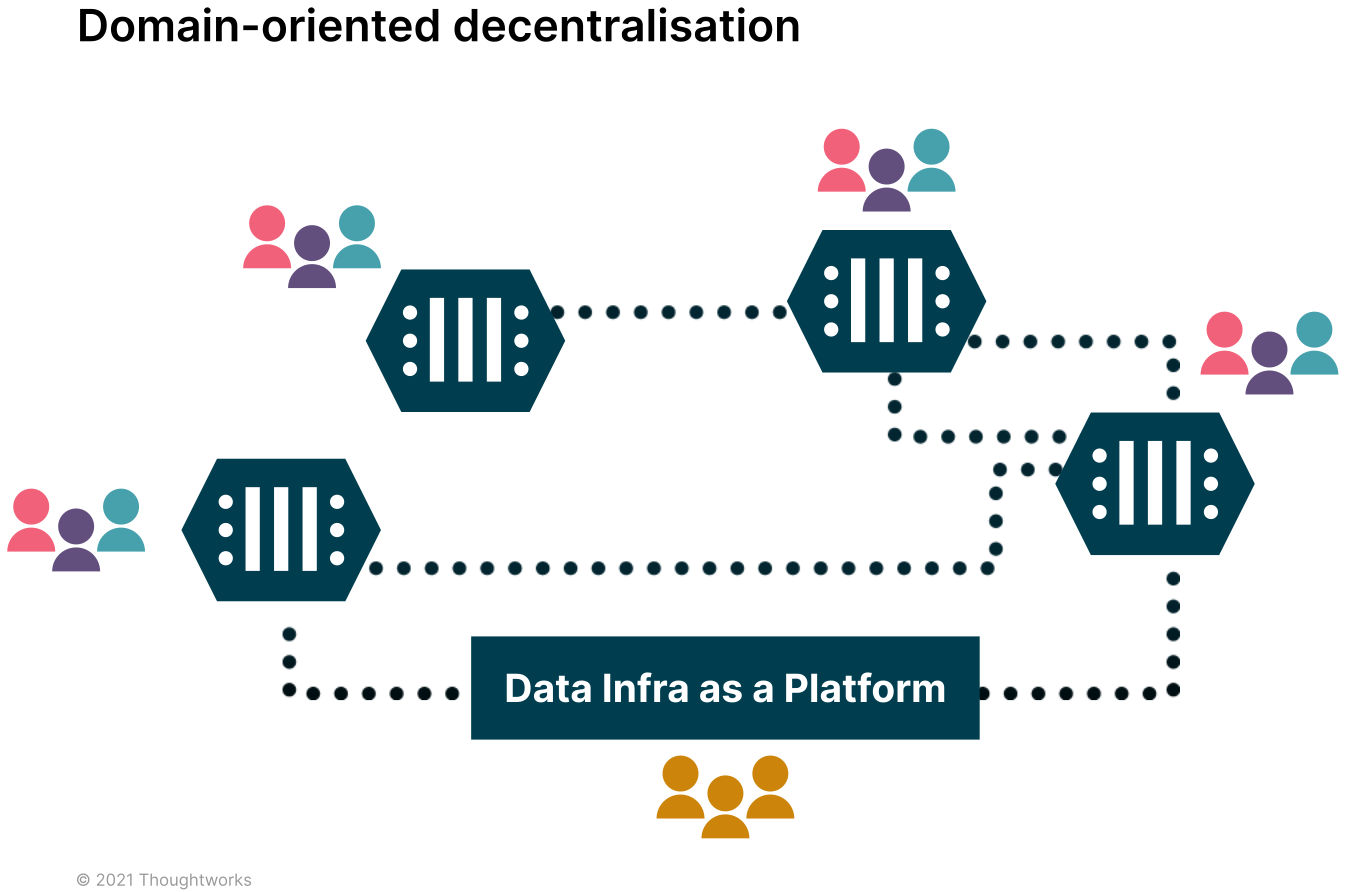
“Things work better when you focus on the product and its needs, rather than the organization of its equipment.” — James P Womack, Daniel T Jones, Lean Thinking.
Lean thinking and data mesh
As a response to the all too common dysfunctions seen in centralized data platforms, data mesh draws on a number of lean principles. Starting with the observation that analytics can be viewed as manufacturing, we can see that the core principles of data mesh involves the application of lean thinking to data management, resulting in value-driven processes and the elimination of waste.
Technological decomposition of data pipelines, with highly specialized technical teams handing work off to each other, is a hallmark of centralized data platforms that results in batch-and-queue inefficiencies and increased cycle times to deliver work. The domain oriented decentralization of data mesh reduces these wastes.
Value is defined by the customer of data products (both internal and external customers). In data mesh, this can be seen via the shift from thinking about data as an asset to data as a product and the resultant application of product management techniques.
The investment in shared self-service data enables data teams to focus on building out data products on the mesh, with flow being promoted through orientation around producers and consumers, with consumers always pulling from producers.
Continuous process improvement is enabled through federated governance, in the form of standardized APIs and processes for measuring data product quality and performance. This in turn enables the application of proven lean techniques such as value stream mapping and management.
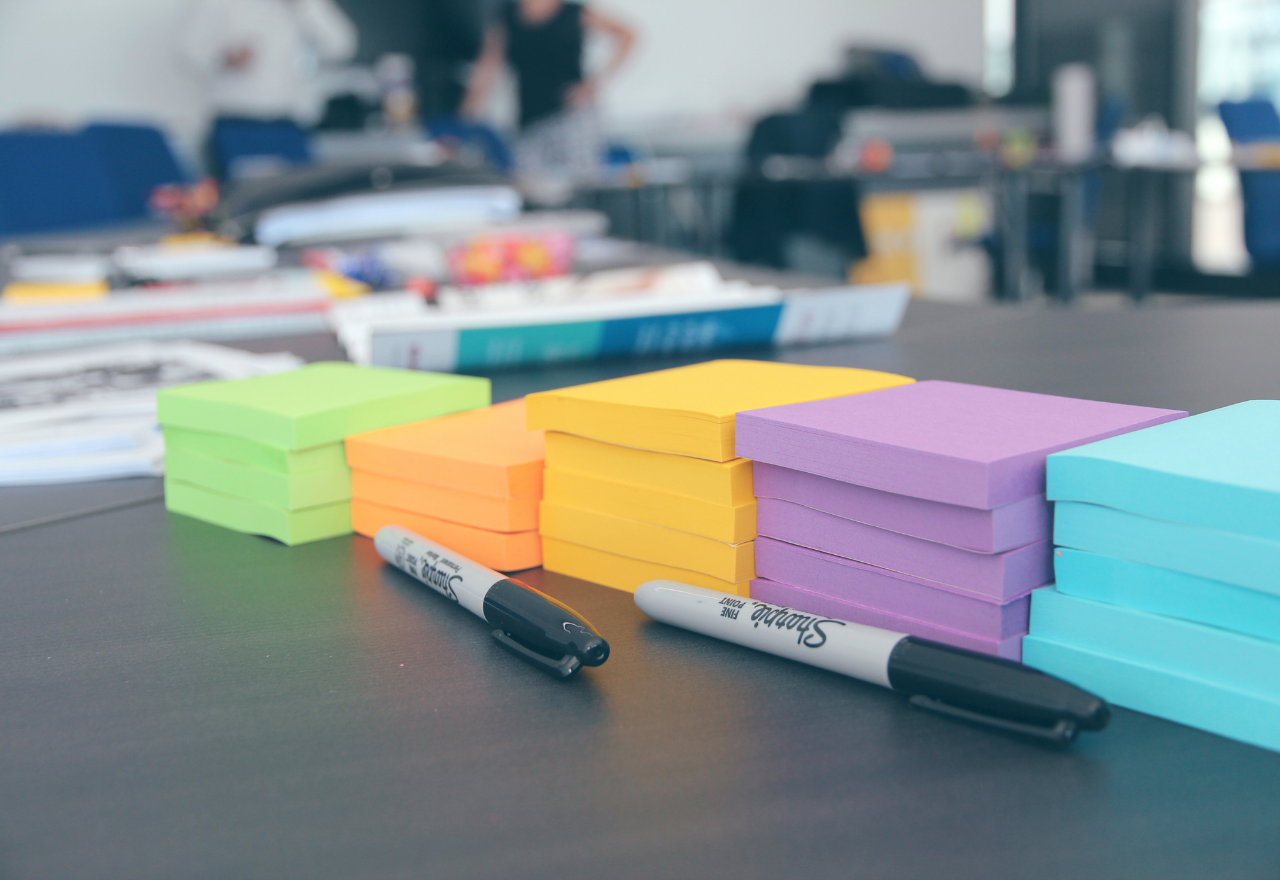
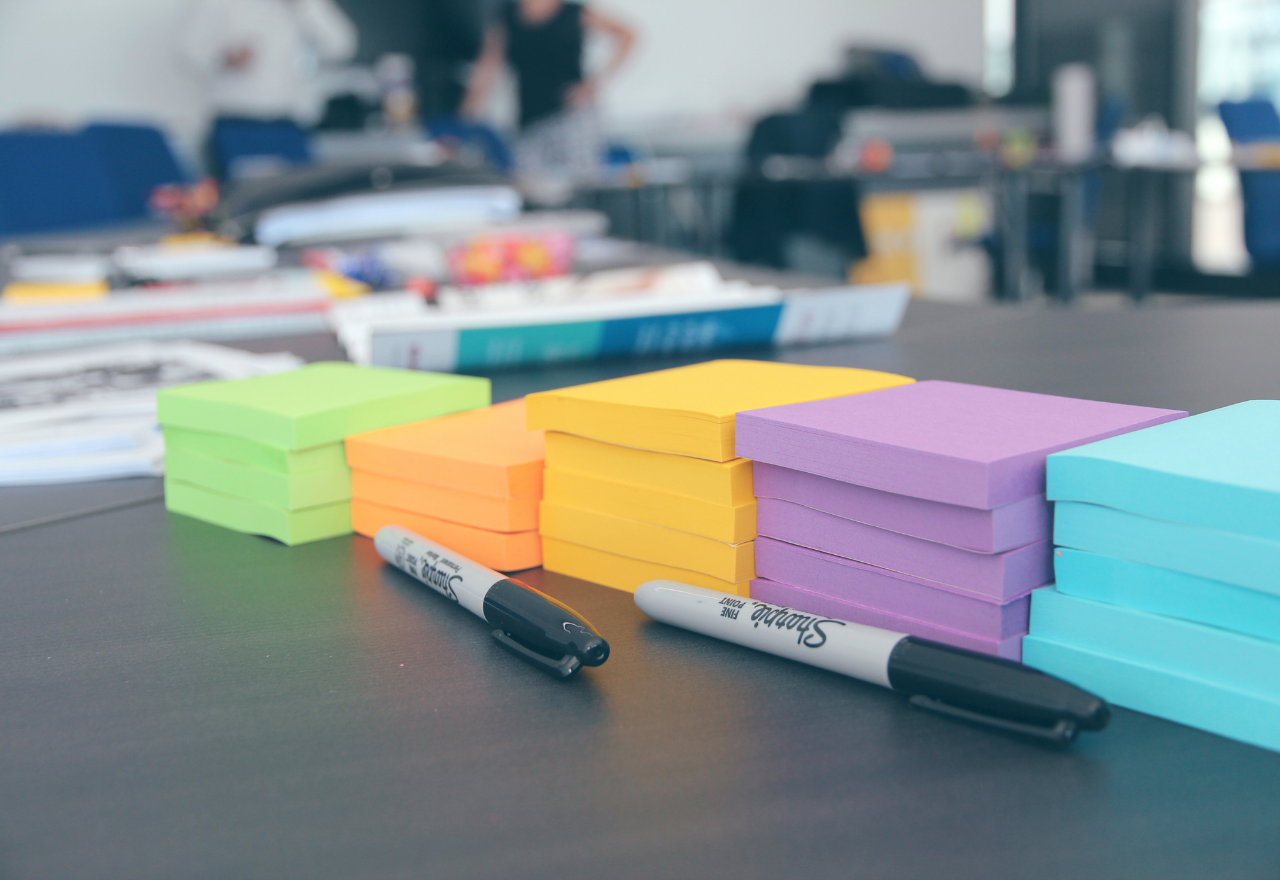
Disclaimer: The statements and opinions expressed in this article are those of the author(s) and do not necessarily reflect the positions of Thoughtworks.