Business leaders are surrounded by cautionary tales of dominant incumbents being displaced due to not taking the threat of disruptive forces seriously. There are plenty of examples from the last few decades, typically featuring innovative newcomers undermining established players (think Netflix and Blockbuster or Facebook and MySpace) or rapid changes in technology and consumer preferences (consider Kodak’s failure to the dominance of digital cameras or Blackberry misjudging the popularity of the touchscreen).
Many traditional Financial Services (FS) firms find themselves at this familiar precipice. Today, they are confronted by the onslaught of costly-to-comply-with regulations, fintechs and non-banks pushing into the market, disruptive technologies upending traditional business models (e.g. blockchain), and, arguably the most crucial factor, rapidly changing customer needs and expectations. Although there are a number of strategic plays firms can pursue to address these looming threats, all too often they do little more than reduce complex challenges into cost reduction problems.
We see artificial intelligence (AI) as the core play FS firms need to embrace over the next five years. Doing so will not only help them respond to these challenges but also drive profitable growth. The opportunity space here is made particularly attractive by the amount of ‘fuel’ that is now available for AI algorithms: valuable customer data (something incumbents possess in abundance). The implications of AI should not be underestimated by the industry: there is a growing divide between firms willing to leverage the power of the data at their disposal and reimagine their business models and those that are falling behind. McKinsey estimates that AI technologies can potentially unlock $1 trillion in incremental value for global banks annually (McKinsey, 2021).
Within private banking and wealth management in particular (largely as a result of aggressive efforts to increase productivity and shrinking coverage models) relationship managers (RMs) are now expected to manage an ever-increasing number of clients. This presents a double-edged sword: while clients expect increasingly personalized advice, RMs must manage a hefty cognitive load, having to contend with increasing volumes of information. This is already having serious consequences, with the EY Global Wealth Research 2021 noting lack of personalization as one of the major complaints from wealth clients (EY, 2021).
Thoughtworks has a set of AI solutions that can be deployed into specific areas across the private banking value chain to help alleviate this double-edged sword:
Prospecting: As attrition and competition for a share of the wallet intensify, prospecting for potential clients becomes more difficult. This is, in part, due to the data on prospects being opaque, unstructured, and difficult to obtain. Here, algorithms could be invaluable; they could be used to aggregate and identify signals from the vast pool of data RMs have to struggle through — emails, video-call transcripts, Linkedin announcements, public reports, company data, etc. AI models would assist RMs by creating a prioritized probability-weighted list of potential clients to target
Cross-selling is a powerful strategy; however, identifying connections between bank targets and those clients already banked by other divisions (e.g. commercial banking) has always been a challenge. Advances in graph-based algorithms now make it possible to identify those connections without sharing sensitive data across divisions
- Personalized advice at the right time. RMs often get the first pick for lucrative investment opportunities that are not actively marketed or open to the wider public, e.g. new private equity fund subscriptions. Here, algorithms can match a client’s unique situation to investment opportunities on the market, so no client misses out on a perfect match. Below is an illustration of how this use case would work:
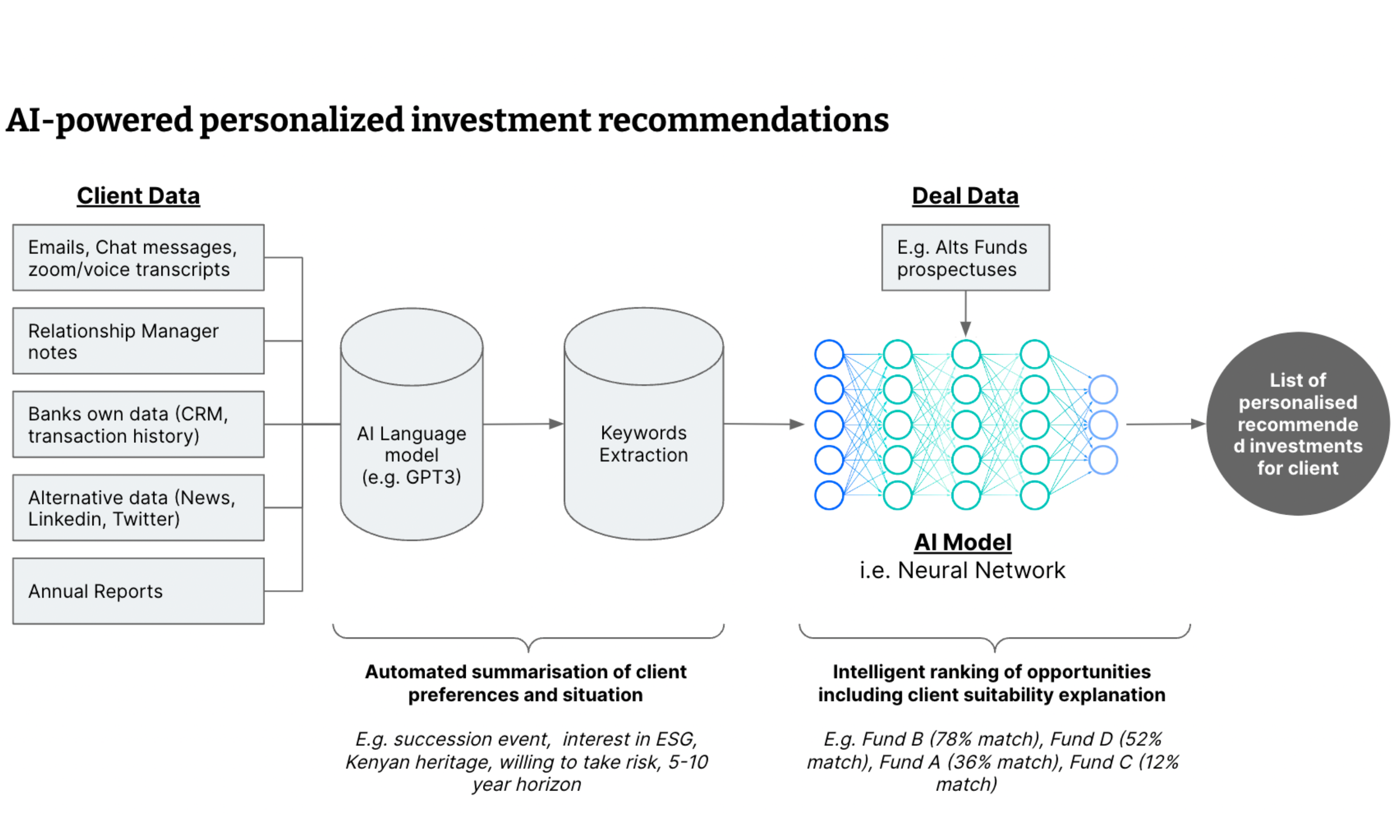
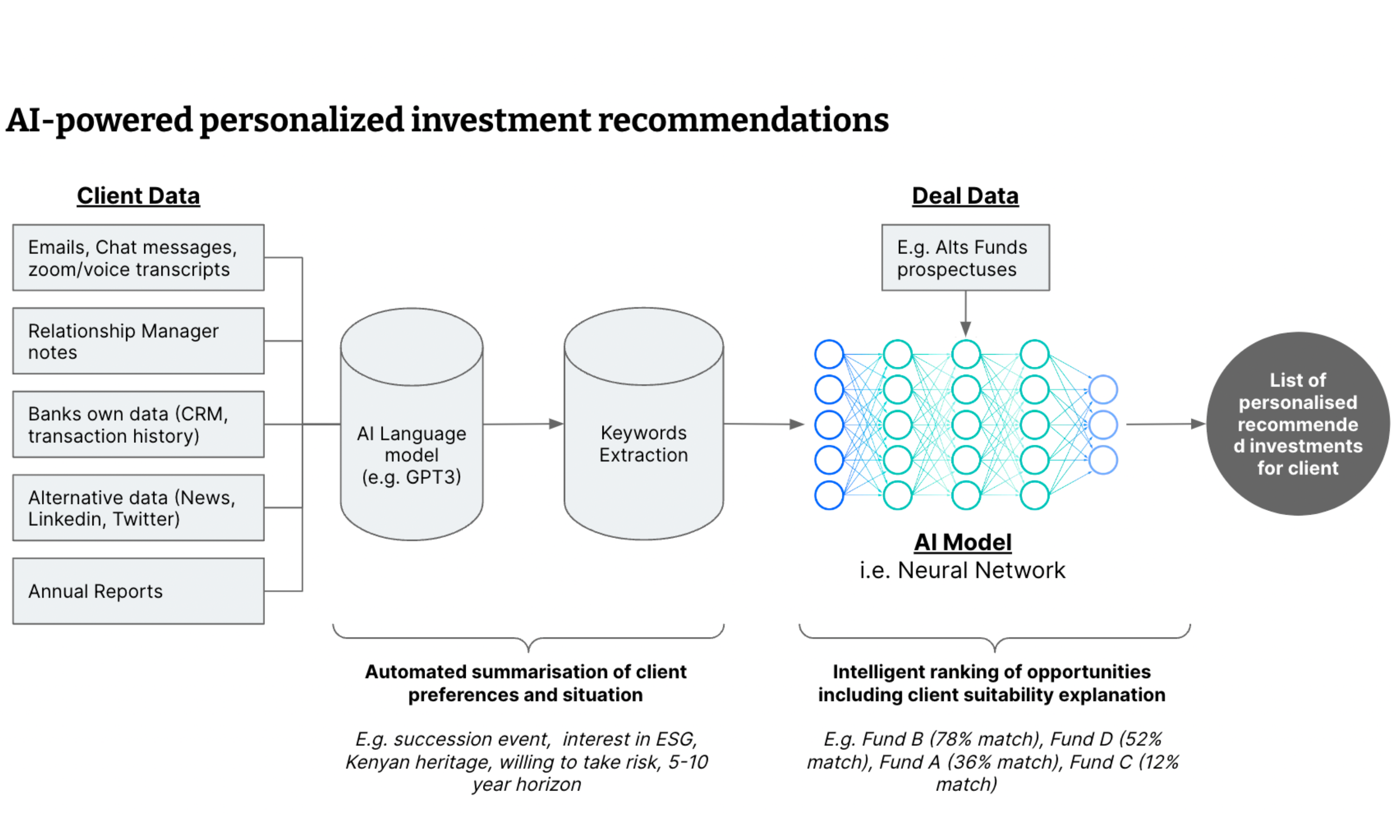
Customer service is one of the most common reasons clients leave. Customers now expect near real-time resolution and exceptional service from their bank, comparable to the experience offered by digital-first organizations. Algorithms can help here by triaging client requests based on their complexity, and routing them to the most appropriate middle and back office staff with the resources needed to resolve them
These use cases only focus on existing operations yet the true growth-generating power of AI is found when it is leveraged to answer strategic questions, such as:
How well aligned are our executives' revenue expectations with probable market realities?
What trends will we see as our client base shifts from older to younger generations?
Which new propositions and service offerings will generate the most interest from existing clients and help attract new ones?
Is our response to short-term economic fluctuations reflective of our client’s values?
In an AI-driven enterprise, these use cases wouldn’t be considered separate. Modeling macro trends builds on a deep understanding of the signals that lead to a new prospective client. Gauging interest in new propositions is impossible without intimate knowledge of which investment opportunities to offer to each of the bank’s clients and when. As shown in the figure below, when AI is used to drive the client experience it generates unique insights that allow wealth managers to better answer strategic questions in a way that competitors cannot.
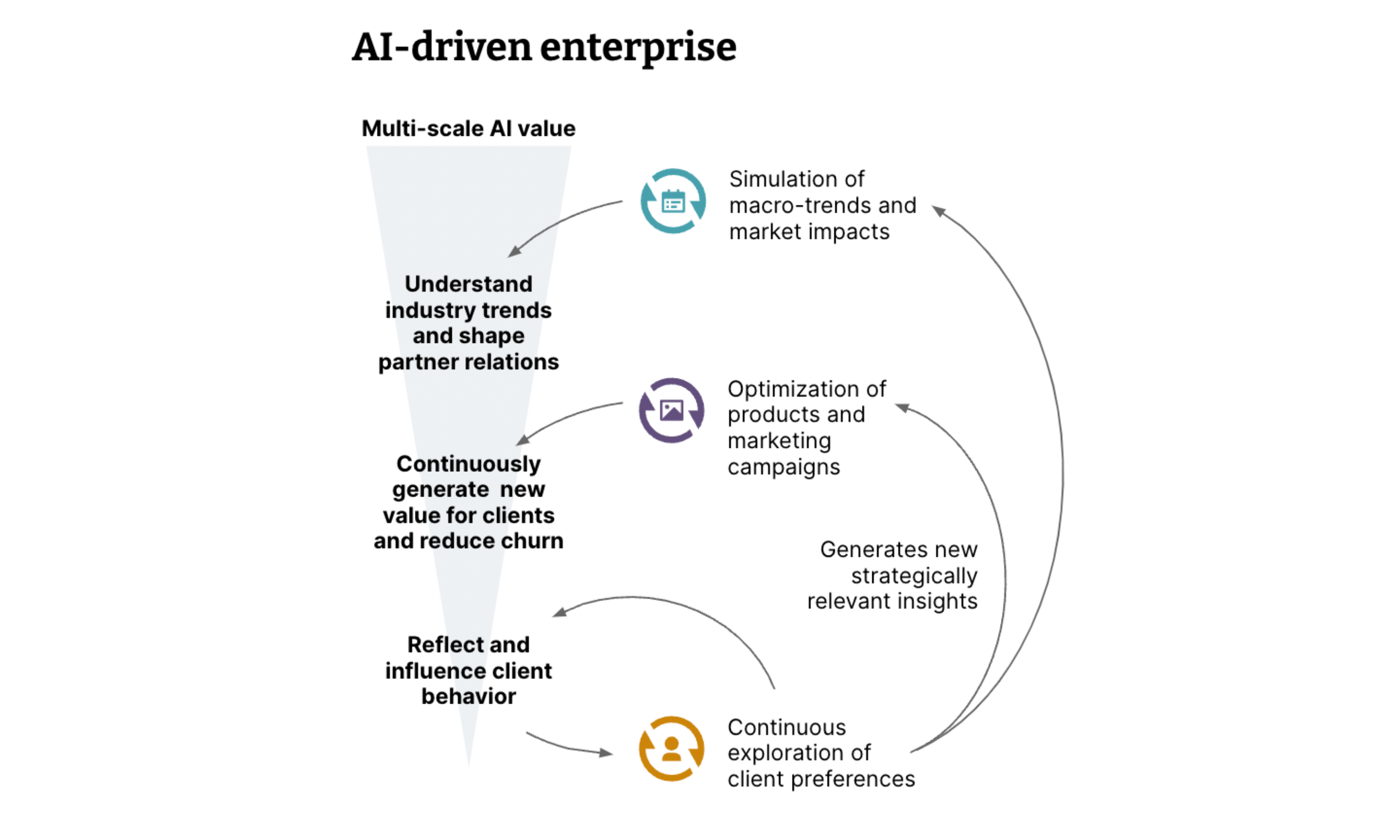
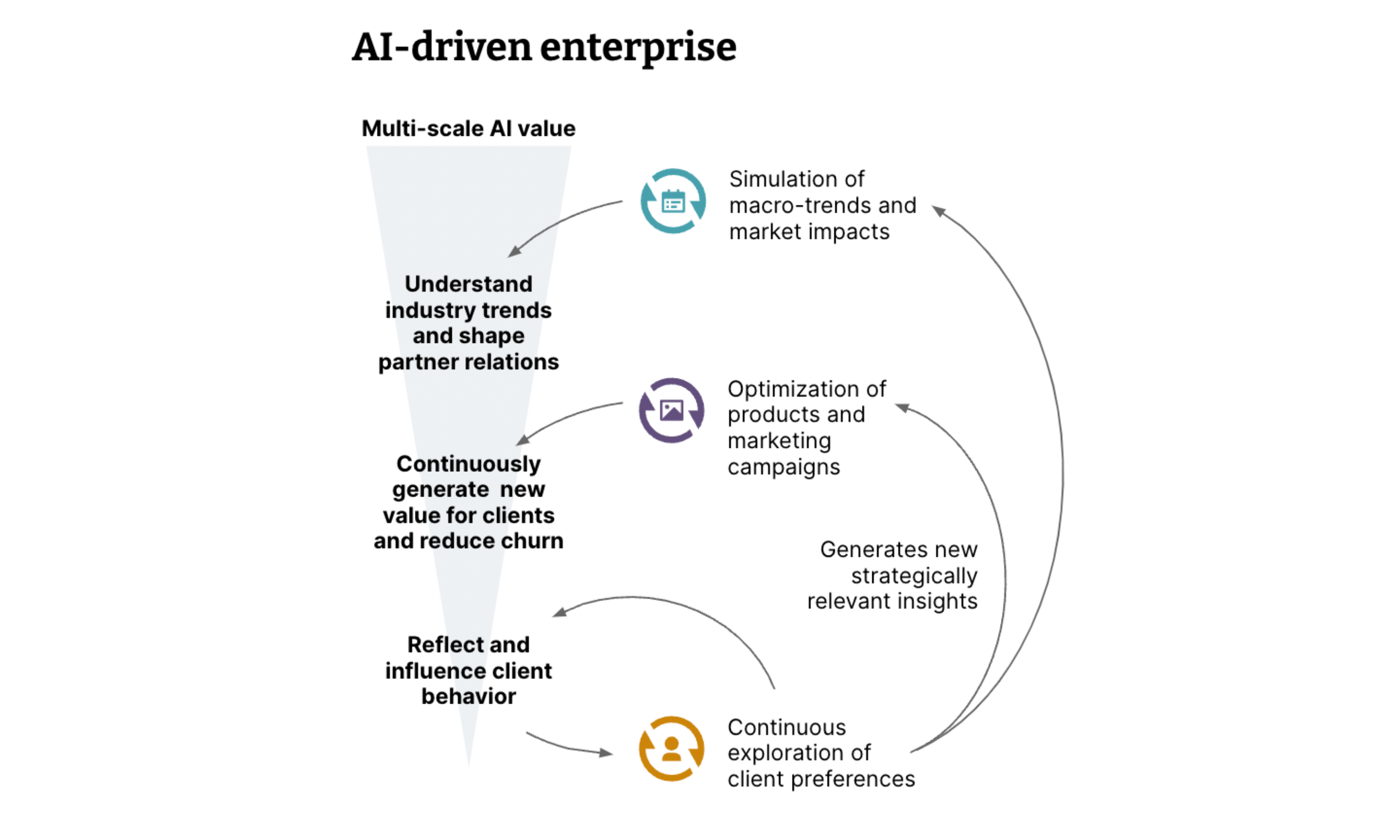
Realizing the value of AI as outlined above requires organizational capabilities that foster continuous experimentation and learning. The goal should be enabling human learning and supporting human decision-making, not trying to force value out of historical data. Solutions aren’t always going to be right the first time, and even if they are, customers and contexts will change over time.
Thoughtworks’ AI: Augmented approach is designed to ensure humans and AI are aligned and work together. By framing AI as a series of experiments, it helps firms adapt and grow in complex and changing business environments. Executives need to remember that AI is not just another piece of software that can be outsourced to SaaS vendors; it is a shift in mindset and an adaptive operating model that requires constant experimentation. In addition, there are practical considerations such as:
The need for ‘AI-savvy’ skill-sets in the organization that span the boundaries of business, tech and data
Managing regulatory constraints on data use and quality, such as GDPR — while still empowering innovation
Deployment mechanisms that support a scalable machine learning approach that takes models into production, and a way to access and manage data and analytics at scale
The secret to success does not lie in the AI itself: it can instead be found in the way AI-backed techniques are actually implemented and used to solve challenging problems. It is for this reason that having a trusted partner on the journey to becoming an AI-driven business is more pressing than ever. At Thoughtworks we apply a set of tools to support firms through AI value discovery and realization:
AI: Augmented — our approach to AI that reframes human decision-making by using computers to help stakeholders think more effectively, not simply predict from the past. This is especially valuable in problem spaces with greater uncertainty and little data available
EDGE — our approach to building the organizational capability to evolve and continuously adapt, that is critical to success as a modern digital business
Product Thinking — our approach to delivery, where we explore problems through design thinking, craft the right solutions with lean practices, and deliver results through agile methodologies
Thoughtworks brings additional technical leadership in enabling capabilities for data-driven enterprise:
- Data Mesh — our approach to enterprise data where data is treated as a product and owned by the teams that most intimately know and consume it. Applying principles from modern software engineering, it helps businesses unlock the true potential of enterprise data
- Continuous Delivery for Machine Learning (CD4ML) — our approach to the process of AI deployment, applying continuous delivery principles and practices to enable teams to deliver AI-driven products quickly, reliably and responsibly