Looking Glass
Lens one: Partnering with AI
Machine learning (ML) and artificial intelligence (AI) continue to gain traction across industries. We are seeing rapid adoption in use cases that range from automating everyday operational processes, to augmenting strategic decision-making. By better understanding the respective strengths and limitations of humans and machines, businesses can develop balanced and productive partnerships between their people and AI to extract maximum value from emerging technologies, while remaining mindful of the potential ethical implications.
Through the Looking Glass
To truly partner with AI, we can’t view it as a tool to solve every business problem. Instead, we must understand where it excels, where it requires human agency to fulfil its potential and where it might prove ineffective.
Some business problems – for example those that necessitate leveraging historical data or fast digital feedback loops – can be addressed by applying AI to completely automate processes or decision-making. However for others – especially those that require creativity, intuition and slower strategic feedback loops – AI’s role is best structured as augmenting human talent.
As AI-supported techniques become more mainstream, they can touch on more areas of our lives and their impacts need to be considered from an ethical perspective. This is driving research and tooling to support explainable AI (XAI) and more robust governance processes, including automated compliance.
Signals include:
A continued surge of investment in AI research and applications. Research firm IDC estimates the worldwide AI market will exceed US$500 billion by 2024
Continued high demand for ML, AI and data specialists in the job market. According to LinkedIn, AI specialist was the fastest-growing job category in 2020, while Glassdoor ranked data scientist as the number two best job in the United States in 2021
Increased growth in ML/AI start-ups, specialized products, IPOs, and acquisitions. In October 2021, Exscientia – a UK company that put the world's first three AI-designed drugs into phase one human testing – went public on the NASDAQ with a valuation of US$3 billion
Changes in existing jobs and roles. The World Economic Forum estimates that by 2025, 85 million jobs may be displaced by a shift in the division of labor between humans and machines, while 97 million new roles may emerge
- Increased public awareness of situations where AI had unintended consequences. For example, facial recognition has repeatedly been in the news due to privacy concerns, and racial bias has been found in Twitter's image cropping AI. Despite that, there are concerns that ethical AI design will not be the norm within the next decade
The opportunity
Most enterprises understand automation can improve workforce productivity, by automating repetitive, mundane and data-crunching tasks. This can fundamentally change or even remove the need for some jobs, but new roles will also be created where people are shifted to higher value activities that require judgement and creativity. Typical areas that can benefit from automation include dynamic pricing, recommendation systems, anomaly detection and supply chain optimization.
In other cases, the work of humans will benefit from AI/ML augmentation, with human and machine performing combined or complementary roles. These tend to address problems that require creativity, intuition, experience and holistic thinking. They involve important strategic decisions that don't get made very frequently — e.g. "What product should we sell next?" or "How can we achieve net-zero emissions?" — but can benefit from machines helping simulate and explore different outcomes or even to generate ideas that humans can then evaluate. Examples include AI-first drug or product development and dynamic simulations for planning complex scenarios like climate change.
All these applications demonstrate how AI can deliver value across the entire organization: by improving internal operations and informing better business decisions, all the way to product innovation and improving customer experience.
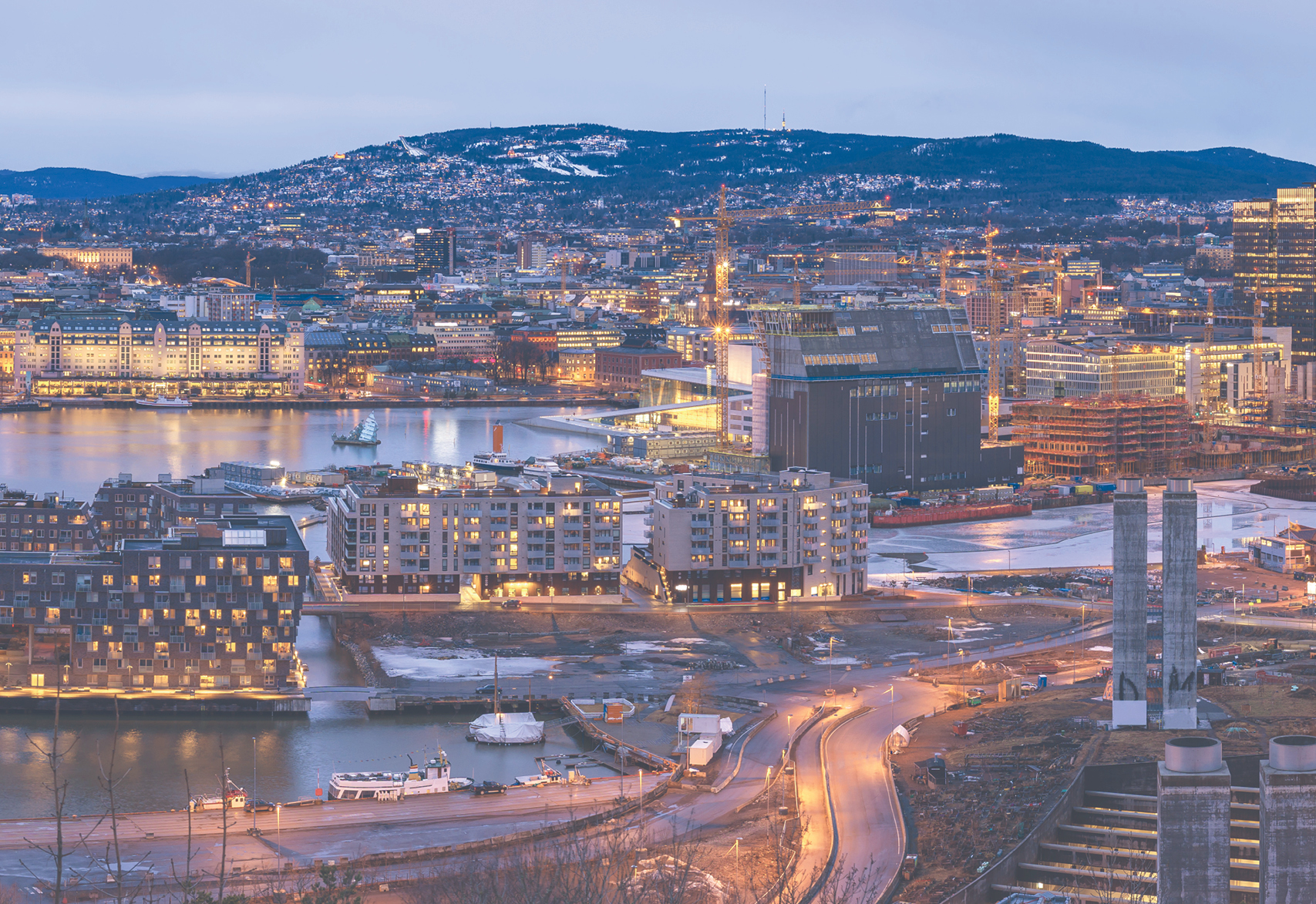
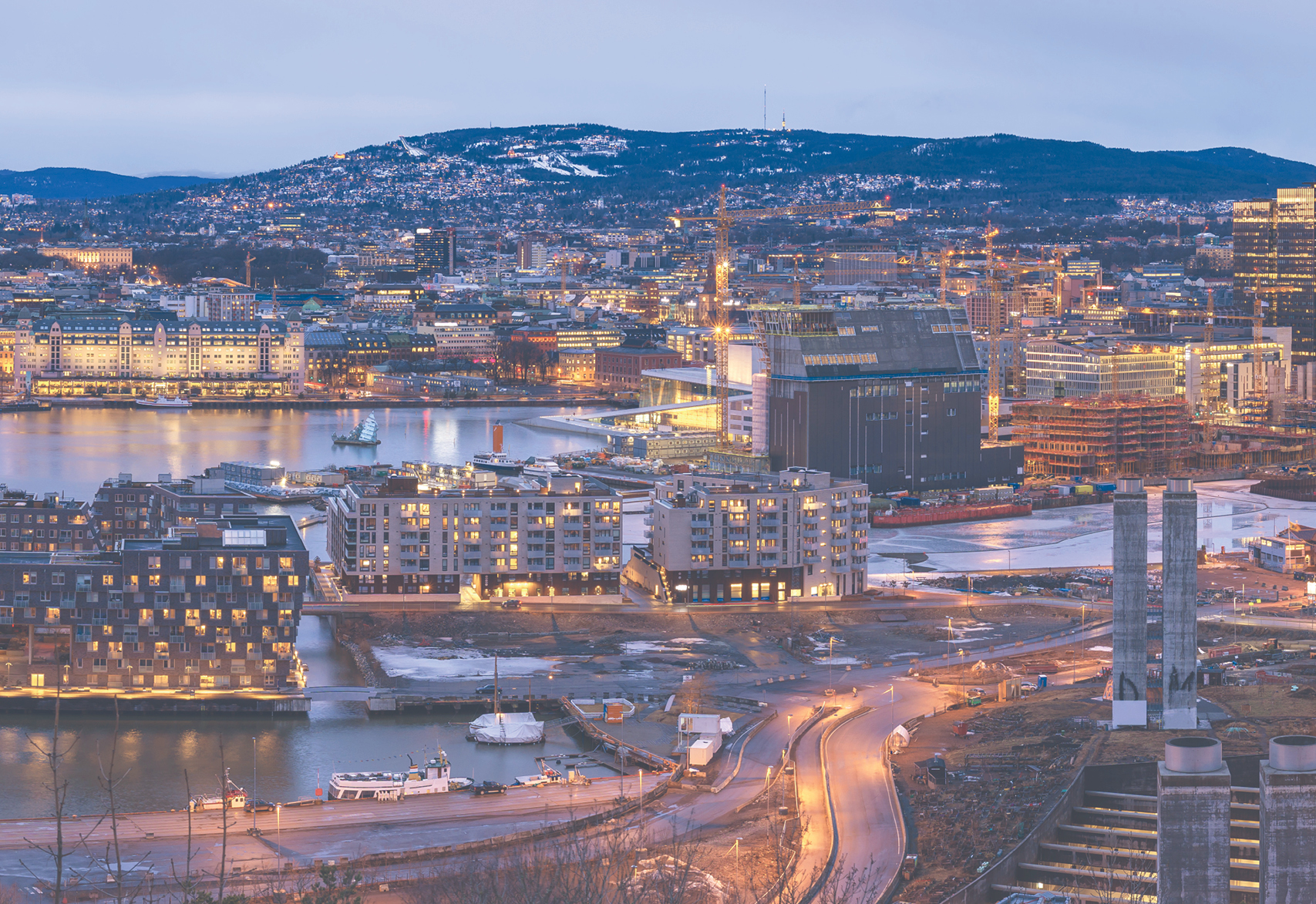
What we’ve seen
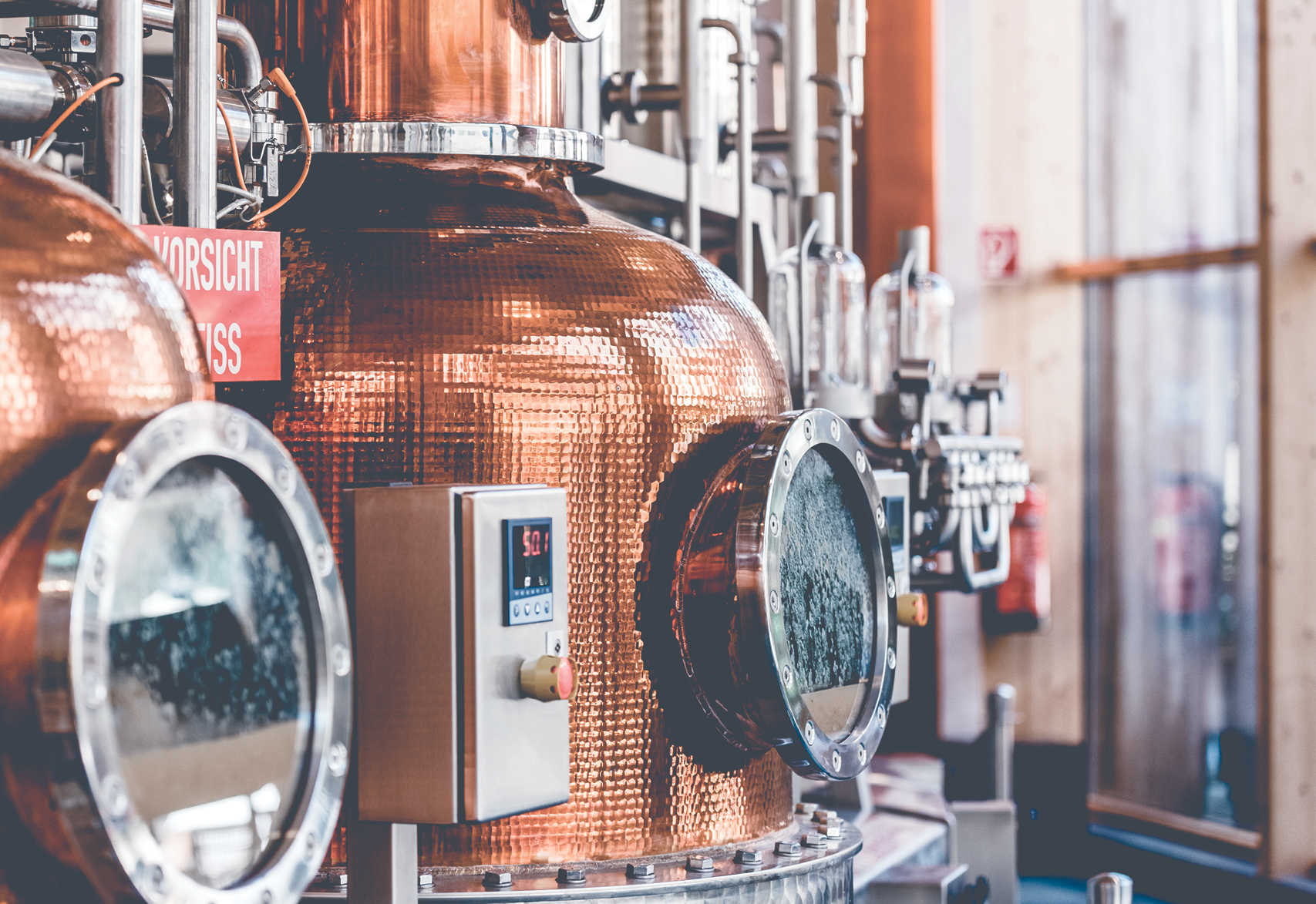
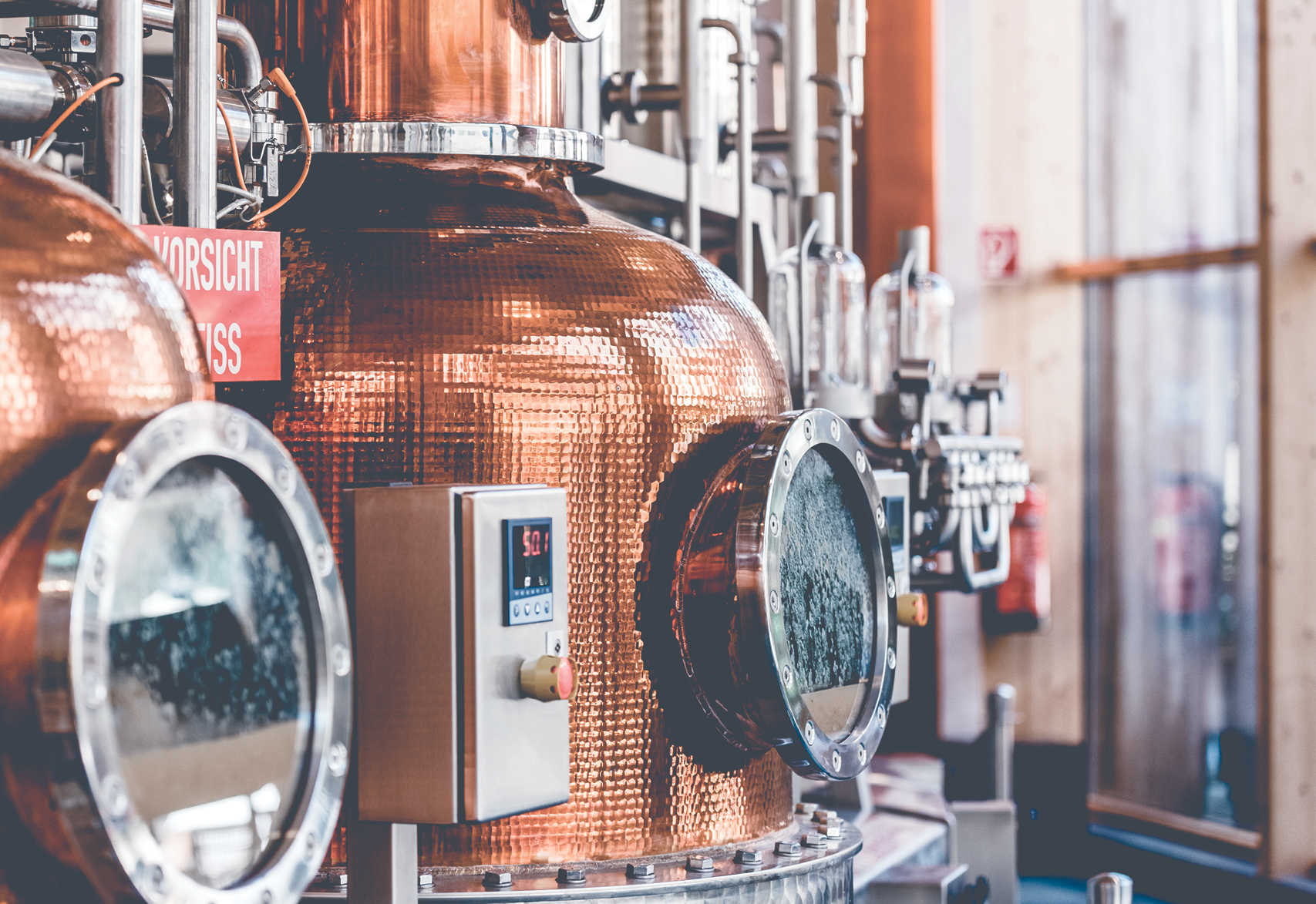
What we’ve seen
Trends to watch: Top three
Adopt
Operationalize AI. At many enterprises, AI and ML adoption is shifting from experimentation to production, growing closer to the customer. On top of developing the technical capabilities to train, deploy, and monitor ML systems (such as CD4ML), there are also process and operational issues that need to be considered. First of all, the governance process to deploy AI and ML needs to take into account audit, explainability, ethics, bias and fairness concerns. Secondly, the business needs to be willing and ready to adopt AI and ML solutions once they are released, which might require change management — especially when roles are impacted.
Analyze
Online machine learning. Machine learning models are usually trained as an offline batch activity, performed over a static data set that doesn’t evolve over time. The need to re-train models more frequently is exacerbated when the data itself changes at a fast pace. Online machine learning techniques enable solutions to continuously learn based on the sequential arrival of data. Reinforcement learning is an example of such techniques, which are less dependent on historical data and being applied in areas like content placement, personalization and dynamic pricing.
Anticipate
Causal inference for ML. Many machine learning techniques use statistical methods to learn, by finding correlations between variables. This works well when the data used for inference has not changed much from the training data. However, correlation doesn't imply causation. Causal inference studies techniques to draw cause and effect relationships between input data and the outcomes. If machine learning models can learn causal relationships, they become more generally applicable and require less training data to perform well. We are beginning to see research trying to apply causal inference-informed ML to real world problems, especially in medical diagnosis scenarios.
Trends to watch: The complete matrix
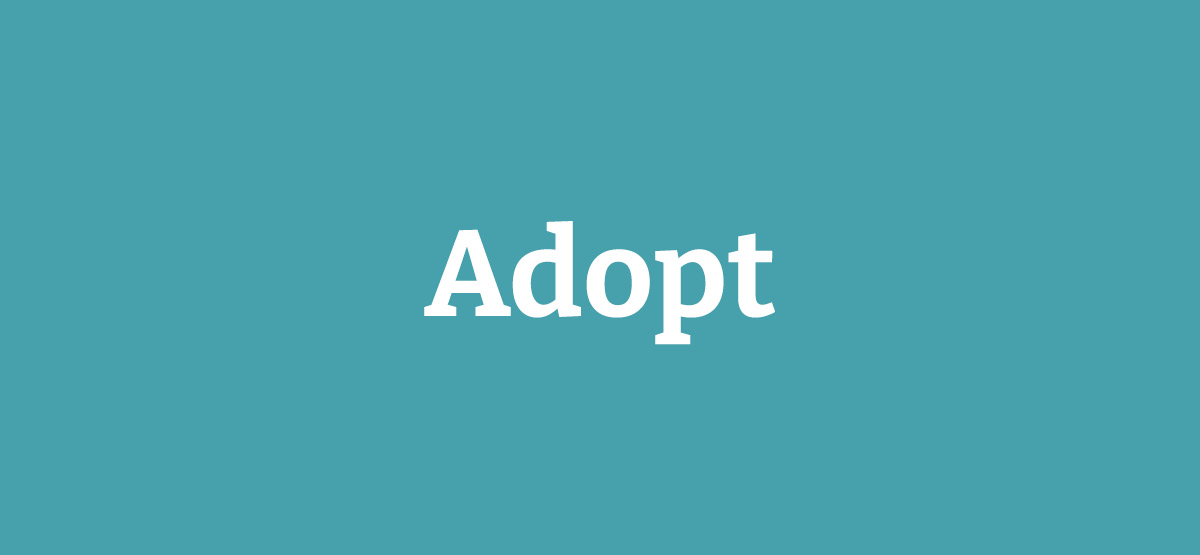
- Natural language processing
- AI as a service
- CD4ML
- Testing ML algorithms and applications
- Operationalize AI
- ML platforms
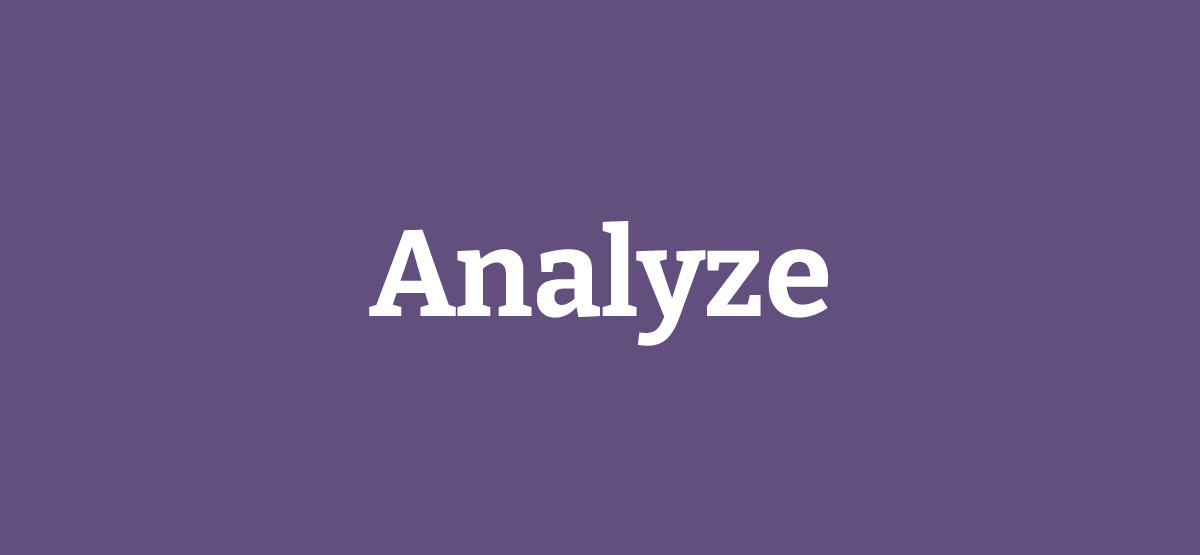
- Computer vision
- Ethical frameworks
- ML/AI on edge
- Explainable AI
- Platform business
- Decentralized data platforms
- Online machine learning
- Synthetic media in a corporate context
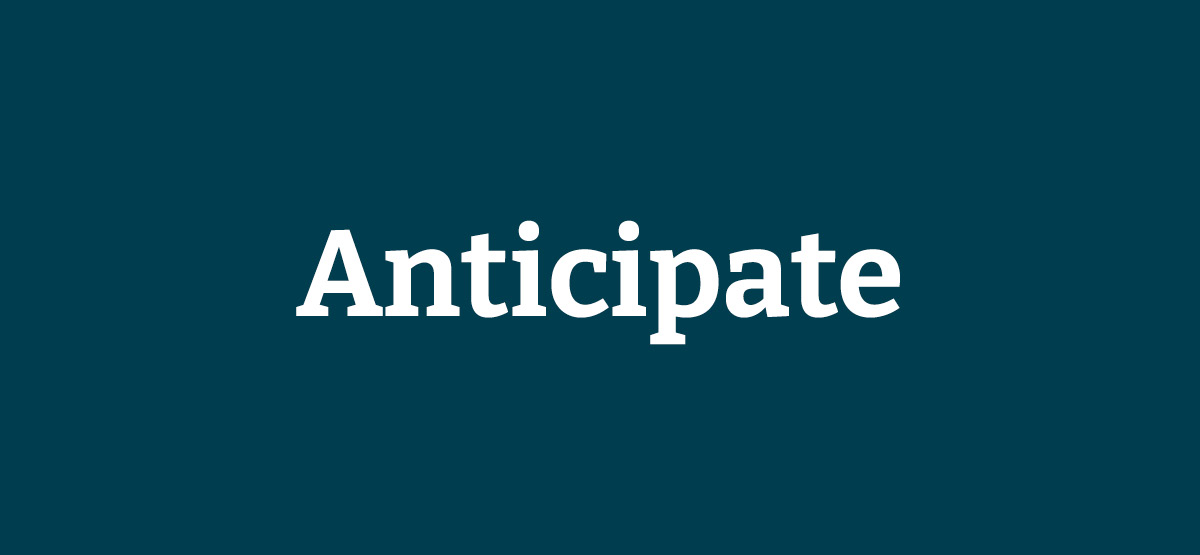
- Code of ethics for software
- Smart cities
- Surveillance tech
- AI marketplaces
- Autonomous robots
- Autonomous vehicles
Advice for adopters
Understand when to use automation vs. augmentation. There are processes or roles that AI and ML can automate completely, and others where they can assist humans to make better decisions, and it’s critical to understand the difference.
Operationalize AI experiments, taking a holistic approach. Consider the business readiness for adoption and the change management required, and build a robust technical and governance process to deploy your AI/ML solutions.
Consider the ethical implications of AI/ML and take the responsibility seriously throughout. AI and ML are extending into complex and sensitive areas such as financing, sentencing and medical diagnostics, where the impact of decisions can have unintended consequences. Bring any potential implications into consideration early on to assess and catch any issues before it's too late.
Prepare your staff for changes to their roles. Don't underestimate the effort required to support and empower employees that can be affected by AI impacting their jobs. Automation can free them up to perform higher-level tasks, while augmentation can help them be more productive. Develop clear transition and career progression plans for them to grow and thrive.
Choose your KPIs carefully. Understand the metrics you are trying to move with AI and ML; measure the current process to capture your starting baseline; and use KPIs to evaluate if AI and ML are delivering real business benefits. With the appropriate benchmarks in place, even if a particular AI/ML approach does not work, you can experiment with different techniques to achieve the desired outcome.
Invest in robust data governance and data management practices. AI/ML techniques still require access to data, and if your data is siloed and hard to access, progress will be painstaking. Consider where data ownership sits within your organization as data quality problems tend to emerge from organizational structures and architectures that don't incentivize teams to produce and share the data resources they have.
By 2023, businesses will…
… understand that AI is not the art of trying to force value out of historical data, but actually the art of creating new data and insight by interacting with the world.