Organizations today face significant challenges in harnessing the power of data and artificial intelligence (AI). To discuss how team structures can drive the success of these initiatives, I recently sat down with Becky Thorn Sietsma and Thiago de Faria from Conflux. Their insights reinforced a growing truth I’ve observed across industries: traditional organizational structures are no longer sufficient to meet the demands of this new era.
The challenges organizations face today — such as moving AI projects from pilot to production, ensuring access to high quality data, and modernizing data management processes and team structures — highlight the need for a more holistic approach to data and AI implementation, one that considers not just the technology but also the people and processes involved. The stakes are also high: for a typical mid-sized organization, inefficiencies could result in up to $30 million lost annually, underscoring the urgent need for transformation.
To address these challenges, forward-thinking organizations are turning to two complementary frameworks: Team Topologies and Data Mesh. These approaches offer a new way of thinking about organizational structure and data management that aligns with the complexities of modern businesses.
Matthew Skelton and Manuel Pais’ book introduced Team Topologies, providing a model for organizational design that focuses on team structures and interactions. It recognizes that teams have a limited cognitive load and offers a way to structure organizations that respects these limitations. Data Mesh, on the other hand, offers a decentralized approach to data management. It emphasizes treating data as a product and aligns well with the team-based approach of Team Topologies.


By combining Team Topologies and Data Mesh principles, organizations can:
Create cross-functional teams that own entire value streams.
Keep data and AI connected to domain expertise.
Reduce bottlenecks in centralized data teams.
Allow for flexible data exchange across the organization.
Adopting these frameworks requires a shift in mindset. Organizations should focus on avoiding the trap of treating transformation as a one-time tool acquisition and resist the urge to map business structures based solely on existing organizational charts. Breaking down silos and functional teams that hinder value flow is crucial, as is addressing the complexities of data handovers through clear documentation and data contracts.
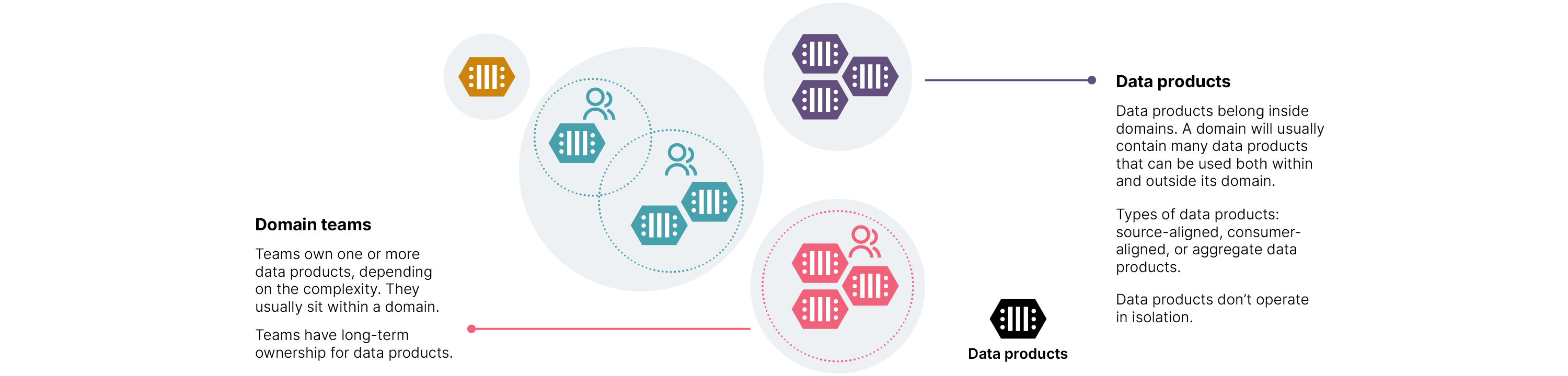
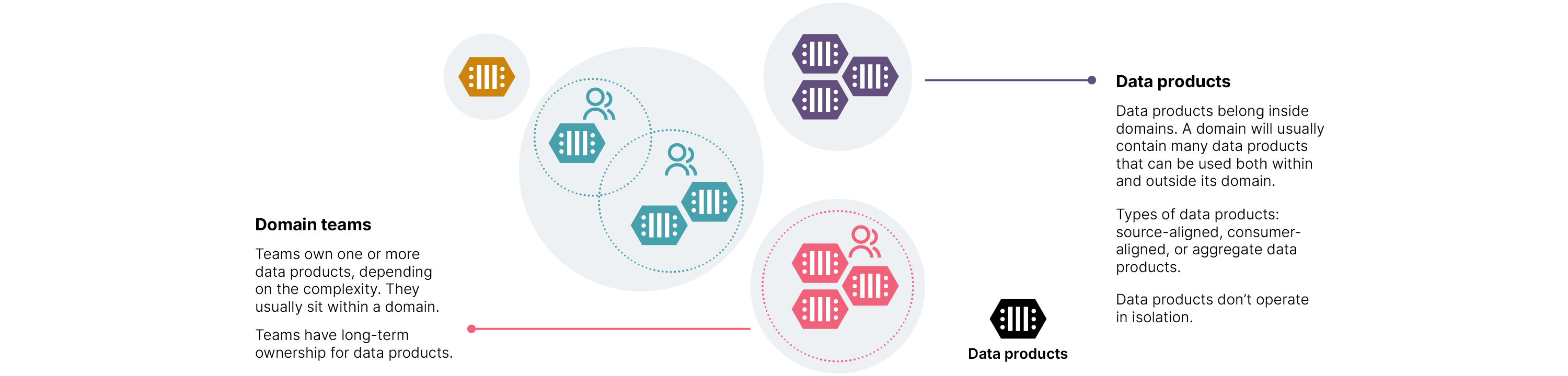
As organizations evolve, they must strike a balance between standardization and innovation:
Create platform teams that provide boilerplates and standardized tools to reduce cognitive load.
Allow teams the flexibility to explore new technologies while offering proven solutions.
Accelerate decision-making processes for platform choices to keep pace with technological advancements.
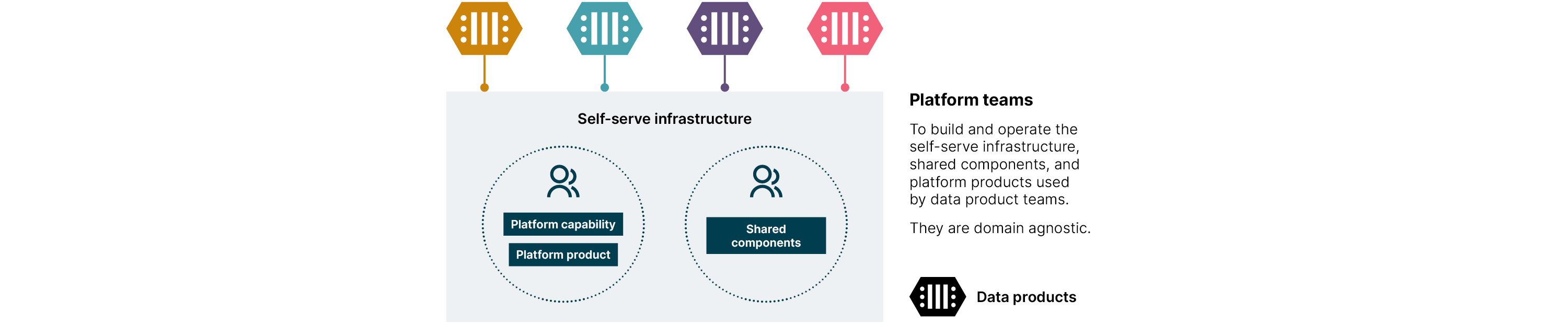
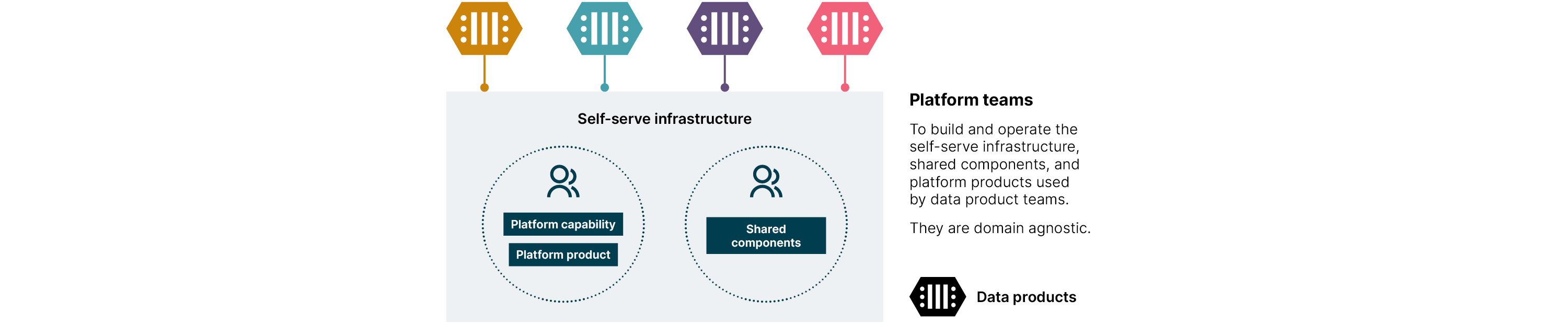
The journey to this new organizational paradigm is not without challenges. It requires embracing complexity rather than shying away from it. Organizations must be willing to evolve continuously, recognizing that there is no "perfect" domain model or data structure. By focusing on creating the right team structures, organizations can develop domains that teams can fully understand and maintain over time. This approach allows for natural evolution and adaptation as business needs change.
The time for incremental change has passed. Leaders must champion a fundamental reimagining of organizational structures to unlock the full potential of data and AI. By embracing Team Topologies and Data Mesh principles, organizations can create environments where innovation flourishes, teams are empowered, and data drives real business value. To uncover the full insights from my conversation with Becky and Thiago, watch the video.
Disclaimer: The statements and opinions expressed in this article are those of the author(s) and do not necessarily reflect the positions of Thoughtworks.