Over the last decade AI and ML have moved from lab and academic scenarios to become commoditized and democratized to a huge extent — driven by the rise in data generation and computing power. The sheer speed of sophisticated innovations (No-code ML, AutoML, MLOps, GANs, Zero shot learning etc.) in this space has been making AI/ML far more accessible, affordable, and easy to use.
This evolution is impacting the way retail organizations plan product life cycles, manage pricing promotions and markdowns, forecast demand and plan inventories. It’s also creating a sea change in the provision of hyper-personalized experiences for customers. AI is being used to analyze behavioral data and leverage computer vision to customize promotions in real-time. Novel patterns and correlations from a diverse range of data are radically transforming decision-making to make it better, faster and economical.
However, while many might view AI as a cure-all, facts paint a slightly different picture. A well-known study from 2018 estimates 85 percent of AI projects will fail and deliver erroneous outcomes. Also, an MIT report states 70 percent of companies report minimal or no impact from AI, while 87 percent of data science projects will never make it to production.
This blog looks at five critical myths about AI’s potential in the retail industry — the idea being to help business leaders who want to further their retail businesses with huge investments in AI and ML tech.
Myth #1 we 'have' to do AI.
The old saying that “if the only tool you have is a hammer, you’ll treat everything as if it were a nail,” seems the perfect way to summarize the expectations of AI as a business tool. Retailers are rapidly, perhaps even blindly, investing in the tech with somewhat unrealistic expectations in place.
For example, let’s look at a retailer who wants to adopt facial recognition in their store. They want to use this tech to decode customer behavior — to know, for instance, which customer will walk into the store, so that they can then provide a more personalized experience. Such an implementation requires a lot of background assessment. Several questions like the ones shared below will need answers:
What problem are they trying to solve with such a feature?
What should be the measurable estimated return for predicting customer intention?
What value are their customers getting?
Do they have the data (facial data from all segments of customers) and technology landscape readiness for such an implementation?
Are the privacy-related nuances addressed?
What are the resource and timeline commitments to build such a sophisticated feature?
How does the overall implementation tie back to larger organization priorities?
What changes are required in their organization?
This is a lot of groundwork to cover for an initiative that will improve in-store customer assistance by a very low margin. In fact there might be many other low-cost technological interventions (like camera feeds to understand the physical attributes of the shopper rather than identifying the person itself) that could achieve similar business goals.
Hence, instead of tech leading their investments, retailers should decide on investments based on business priorities. We recommend having a quantitative baseline of current processes to see where the problem lies. We would suggest first identifying the problem and then deciding which solution to apply. A solution that may or may not have AI in it.
Myth #2 AI will replace humans.
Humans play a critical role in the use, innovation and the implementation of AI. With this in mind, one of the primary lenses in Thoughtworks Looking Glass 2022 is called Humanity Augmented. Partnering with AI — or if you will, the evolution of human-machine experiences is going to be one of the most significant tech-led business changes we should expect in the near future.
AI/ML is a catalyst for augmenting, and not replacing, human talent. AI’s focus should be on automating repetitive tasks to enable humans to evolve their experience, creativity and intuition — that cannot be replicated by machines.
Today, several fashion brands are using AI to predict market demand and respond quickly with the right product mix, inventory, pricing and category management of trendy and customizable styles. AI can help restructure the way brands work on the required product design and development while also predicting what customers want to wear next. But these AI designs take their lead from the ecosystem’s human designers, trends from fashion shows and merchandizers.
Interestingly, Google’s Project Muze (ML-based fashion designing in partnership with Zalando) developed many designs that were completely unsuitable. On the other hand, brands like New Balance are partnering with AI platforms like Heuritech to predict where to take the design of 80% of their collection. This approach has greatly increased the design team’s efficiency, allowing them to be more creative with the remaining 20%. And increasingly, designers are able to validate new design recommendations using AI.
Myth #3 all we need is a pool of data-scientists.
To thrive in a data-driven world, business leaders do not have to spin up a data science lab. It is not the main ingredient when developing ML models. Data scientists do play an instrumental role in AI initiatives — however, there has to be an ecosystem of resources in play to realize the true value of ML models. The lack of cohesiveness amongst data scientists and other players in an ecosystem can produce inferior outcomes, increased costs and deployment delays. Appreciating the role other stakeholders play in the ecosystem ensures productionizing ML models at scale alongside being resilient and scalable.
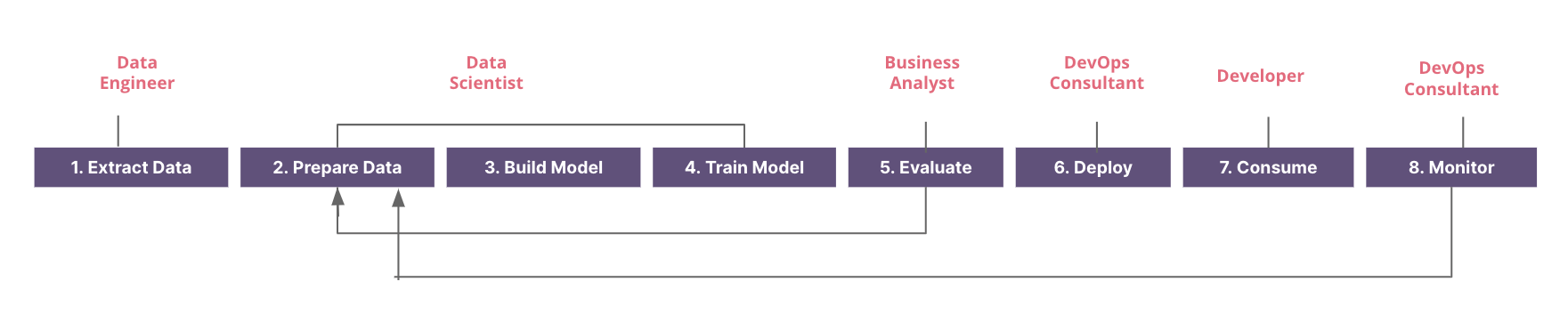
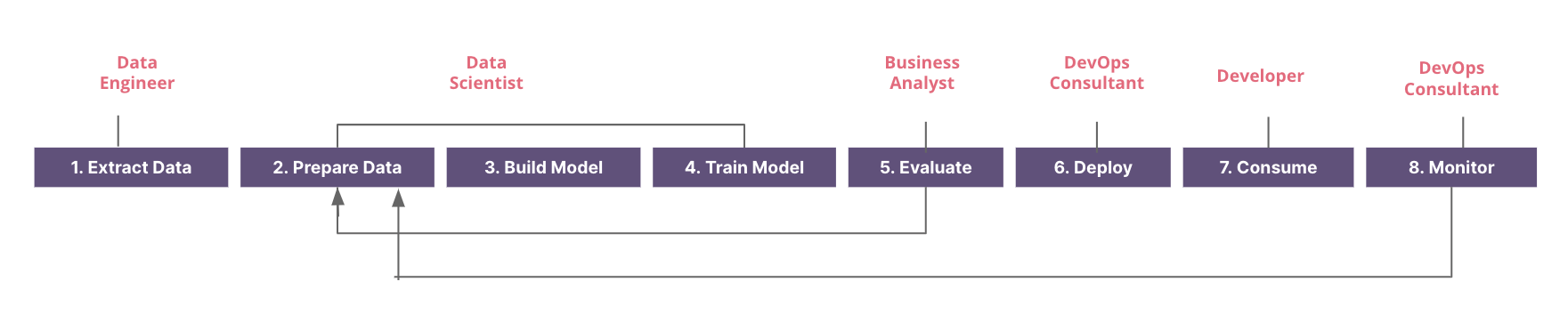
Stakeholders in the ecosystem, apart from the data scientists
As evident in the above diagram, out of the eight key stages in an ML project, data scientists are responsible for only three stages – less than 50% of the life cycle .
For instance, Kroger created a subsidiary unit called 84.51° (a retail data science, insights and media company creating relevant and valuable experiences) to churn out retail analytics use cases and transfer customer data into actionable knowledge. Rather than focusing on building only a data-science team, Kroger’s holistic approach helped it actionize AI.
Myth #4 AI is very easy and we’ve just finished a successful POC.
As per a NewVantage Partners survey of 70 Fortune 1000 companies, 91.5% of companies are reporting ongoing investment in AI. However only 14.6% have deployed AI into widespread production. This contrast is evidence of several organizations falling into ‘model-debt’, with undeployed models that are experimentally successful but lack infra-readiness to deploy at scale.
As discussed above, creating an enterprise level AI solution requires bringing the IT/devops and data science teams together. Production-ready AI pipelines should include versioning, model managing, scalability and traceability – a comprehensive ML ops strategy. However, unlike traditional software, AI model performance should be continuously monitored and measured, with incremental data, as variation in attributes can be pivotal to ensure model effectiveness in production
For instance, AI makes ‘out of stock prediction’ significantly easy for stores in an area. But making that model consumable and able to seamlessly trigger timely insights that can deploy field sales persons to the right place at the right time, at a regional (if not international) level — all that requires a lot more effort than a proof of concept might need.
Myth #5 model accuracy is the north star.
When businesses start considering high model accuracy as a success measure of AI, it’s indicative of a fundamental mistake in analyzing AI use cases. An AI use case goes beyond model accuracy, as once deployed, it starts generating automated decisions which if not captured with the right business and human lens can put the entire effort in vain.
Additionally, the growing need is to build responsible AI that goes beyond precision or accuracy metrics like the F1 score of an ML model. Inclusivity, privacy, explainability, trust and security of the model should be incorporated into the process right from data collection to deployment through to continuous learning from model performance.
Initiatives like personalization in digital commerce should be based on A/B testing so that customer feedback can be incorporated early on and AI models can be improved iteratively before they are launched in the market.
In conclusion, the potential of AI as a disruptive force is hard to dispute, however, organizations should focus on building adaptive AI – which can self-adjust (minimum manual overhaul) based on the inevitable changes in customer, competition and operating environments. This approach will bring increased differentiation for retailers across the board.
By bringing decision intelligence to augment human being at the heart of retail functions, retailers can leverage through a unified, connected, decision making framework that learns continuously from the environment and make predictions time-relevant and self intuitive.
Disclaimer: The statements and opinions expressed in this article are those of the author(s) and do not necessarily reflect the positions of Thoughtworks.