When it comes to data in business, the grandiose metaphors flow fast and furious. We’ve all heard data is the new oil. Perhaps even the new currency or competitive advantage. What about the new corporate superpower? Or the very lifeblood of capitalism?
The comparisons may vary, but the implications are the same. Data is valuable. Essential. A Very Good Thing. The enterprises that aren’t vacuuming it up and using it in spectacular ways are doomed to be left behind. Perhaps even to go the way of the dodo.
Cue a great deal of corporate panic. Companies are lying awake at night (or would be, if they needed sleep), tortured by difficult questions: Does my data classify as "big" data? Am I doing it right? How should I store it, who should get access, and whom should I put in charge? Just how many data scientists or artificial intelligence solutions am I going to need?
The natural response to the surge in interest in data (see chart) is to try to capture every last scrap of it. And so we’ve entered the era of data gluttony. More is more. The bigger the data, the better. The exponential rise (and eye-popping valuations) of companies with data-based business models—Amazon, Uber, Airbnb—adds fuel to the frenzy.
Search interest in data terms over time
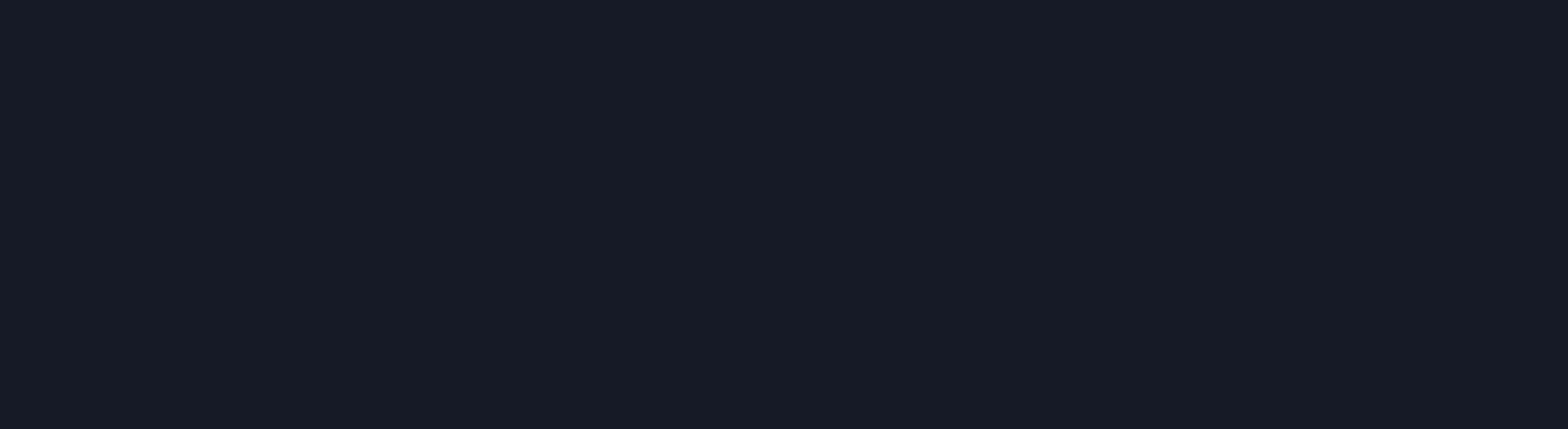
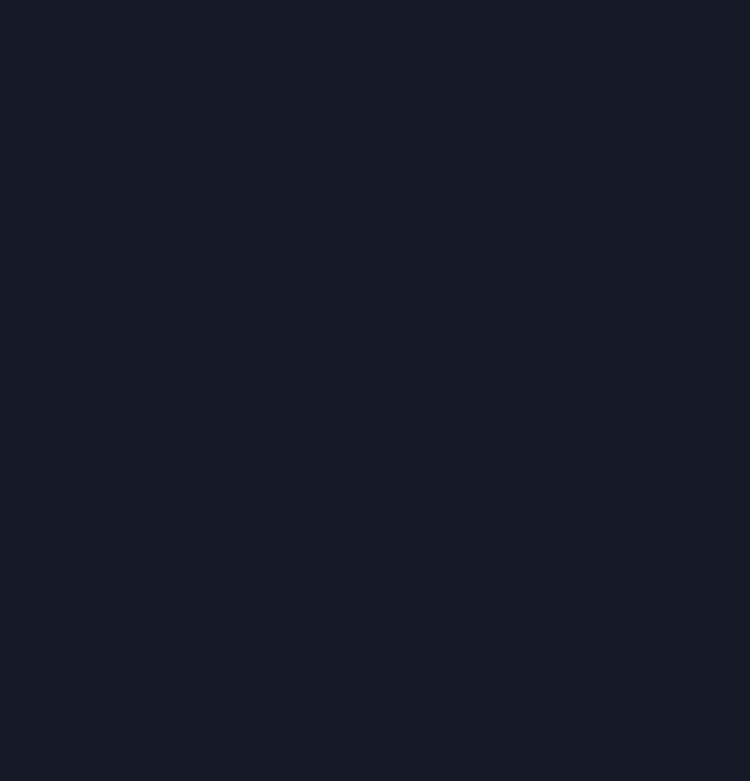
We’ve all heard the success stories. Netflix’s recommendation engine, powered by big data, has saved the company an estimated $1 billion in customer retention since it went live in 2009. Even lumbering UPS has adopted data analytics to enhance its logistics network, aiming to save up to $300 million annually. Research by PwC has found over half (54%) of business executives saw productivity improvements from adopting AI solutions.
Yet despite all the chatter to the contrary, data initiatives don’t always work out. More than a few enterprises devote massive resources to gathering and storing data, implementing analytics, and building vast data lakes, but not a lot changes. By some estimates, 85% of big data projects fail. Organizations find themselves data rich yet insight poor.
Why is this the case? Partly because the data craze has created myths that need to be toppled. Google search data shows interest in the term "big data" has eased slightly while interest in "data scientists" and "artificial intelligence" is on the rise. This suggests businesses are looking for ways to make sense of the data hype. There’s no doubt most organizations need a data strategy, but now more than ever that strategy should be based on principles grounded firmly in reality. We asked leading data experts, inside and outside of Thoughtworks, what those principles should be. We used their insights to develop questions to help organizations evaluate whether they’re on the right track or still in the land of data fantasy.
Principle 1: Data needs to connect directly to business purpose
"Never, ever do a pure infrastructure project—without immediate business value, it’s almost certain to fail," says Dr. Christoph Windheuser, Global Head of Artificial Intelligence at Thoughtworks. "The most important questions a business leader should ask themselves before kicking off a data project are 'What am I doing here?' and 'What is the value I’m creating for my company?'"
That companies should mesh data and business value seems obvious, yet organizations are so taken with "keeping up" they forget to ask the most important question of all—why bother? All that talk about data as the new gold or oil probably isn’t helping. It’s important to distinguish between data—a collection of values or parameters—and information—a value that resolves uncertainty or can otherwise be useful to the recipient—the "signal" to data's 'noise."
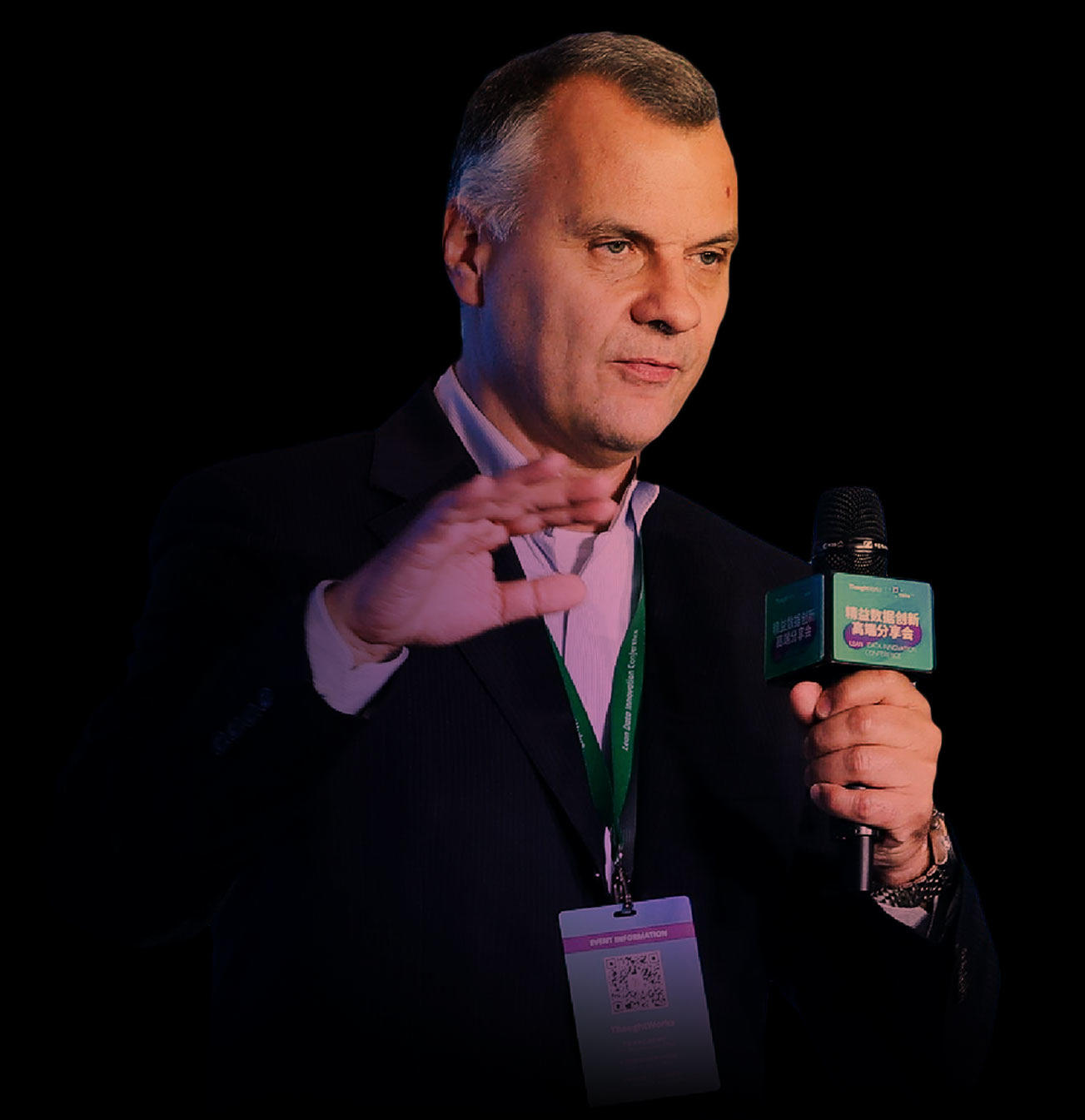
“Never, ever do a pure infrastructure project—without immediate business value, it’s almost certain to fail.”
Dr. Christoph Windheuser, Global Head of Artificial Intelligence, Thoughtworks
The best information results in insights, teaching the recipient new things and serving as a basis for action. But as businesses typically produce and store avalanches of data as a result of the most mundane day-to-day operations, finding/generating the information requires knowing where to look, and what data to prioritize, gather, and process. That can’t be done without a good grasp of the questions the business needs to answer, or the problem you’re trying to solve.
The need to link data projects to concrete business goals is a clear argument for the involvement of senior business leaders at the earliest stages—and means such projects should never be strictly "technological" ventures.
If we accept the premise that the initial driver has to be the business outcome, you have to have a business owner who’s willing to pull that team together, who can both provide insight on the problem you're solving and start to influence the rest of the decision-makers around actually making use of the data to drive decisions. That’s very much an organizational and a business issue rather than a technical issue.
Principle 2: More isn’t always better (or, startups could teach you a thing or two)
If bigger data really is better, many established companies are in pole position as they typically have more historical data at their disposal than a startup. It’s true that bigger firms excel in terms of volume but this often comes at the expense of clarity. As Rachael Hadaway, SVP of Product at Kroger-owned 84.51°, pointed out at Thoughtworks’s executive event ParadigmShift, "Having lots of data is not an asset; it’s a liability. It’s cumbersome and expensive. The asset is knowing what to do with the data."
Parsons said, "Most enterprises have got much more data at their disposal just because of how long they’ve been around. But that data is locked up in systems that are not necessarily well integrated, particularly given all of the M&A activity. You’ve got data coming from different organizational homes that has to be reconciled, and so the scope of the problems you have to deal with, from an implementation perspective, is completely different."
Similarly, established enterprises may have people with the potential to push new approaches to data but Mark Brand, Independent Consultant, believes they tend to be overlooked. "[They are] lost in a sea of other general roles and people who don’t draw a line around data-driven decision making," he said. "And therefore, they can never break out and do it."
A hungry young startup, by contrast, can embed data in the culture, and adopt a much nimbler architecture, straight out of the gates. At such companies, virtually everyone is "expressing things in terms of metrics and spreadsheets, writing their own algorithms or rules and asking the tech guys to deploy them," Brand said. "Even the people handling functions like branding are data-driven. They’re always collecting data, and they all talk in numbers towards enterprise outcomes. That’s what it looks like to be a data-driven business—not necessarily using AI or having a cutting-edge product."
Tech behind legacy enterprises vs. data-driven businesses
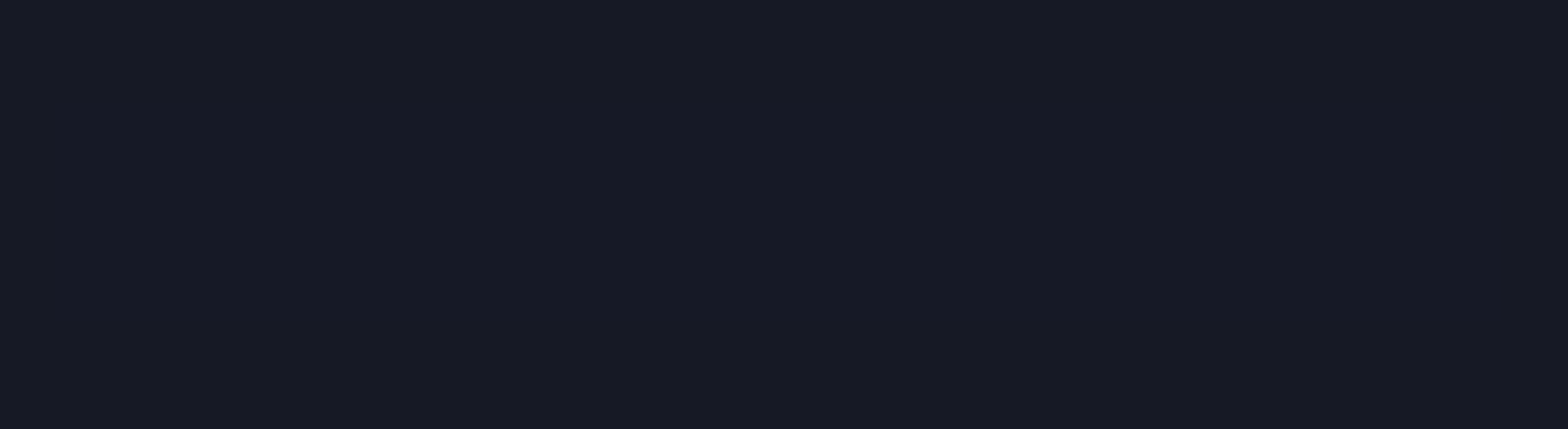
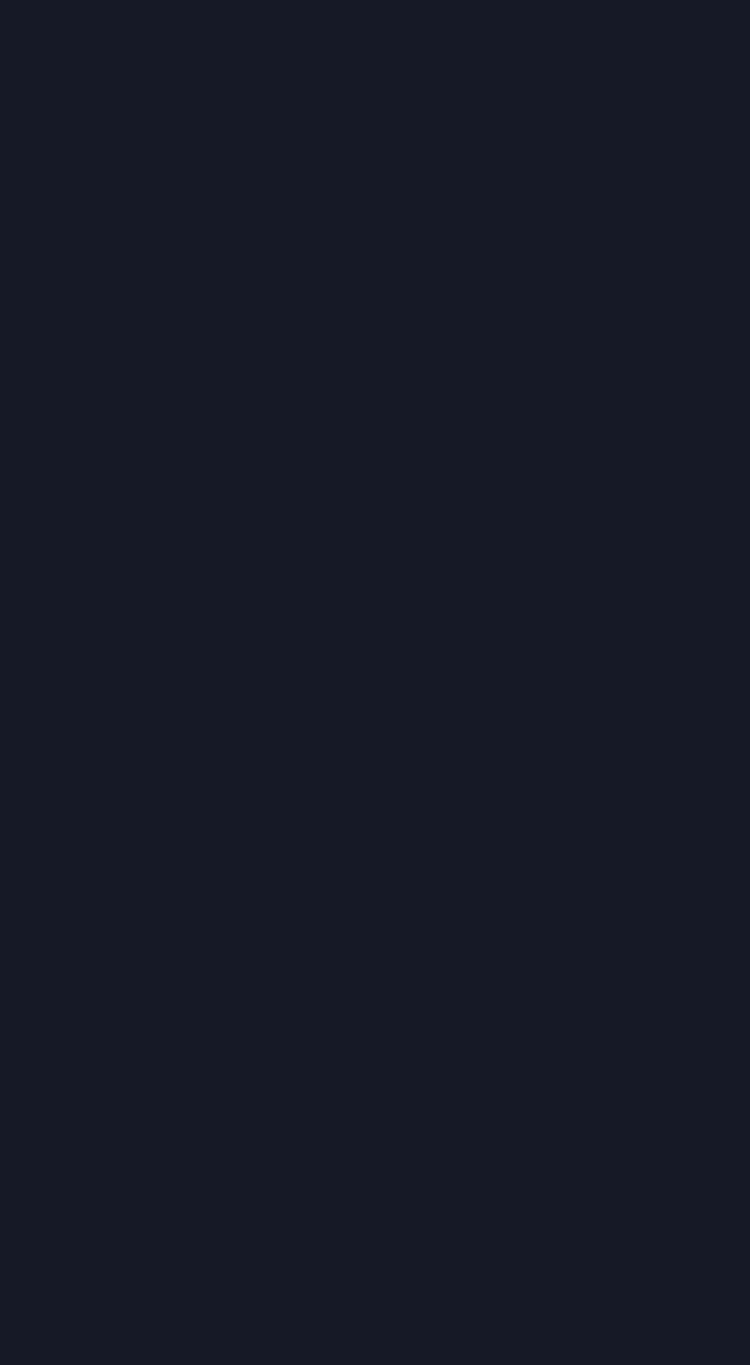
"What successful data-driven startups really have in common is a data-driven mindset throughout the whole organization," agrees Windheuser. "When they design a process, it’s data-driven by definition. Data governance is part of the culture and not something the company has to work on or define in a cumbersome process. All the IT systems and architecture are built with the free flow of data as a major requirement. In traditional big companies, that’s not the case. They have to change their culture as well as their IT systems."
Principle 3: Data lakes won’t save you (and neither will AI)
The hype that often accompanies new data-based approaches has fostered a "keeping up" mentality in some quarters. Solutions range from data "lakes" that pool data from across the enterprise to AI systems that promise to flag anomalies or predict customer trends. Organizations may leap to adopt them before understanding what they entail or considering where, or whether, these solutions fit the purpose.
There’s a tendency to see these innovations as "magic tricks" that solve all data-related problems in one fell swoop, which is misguided, Windheuser notes. Yet it’s also wrong to see them as all style and no substance. Data lakes, machine learning and AI are best viewed as no more and no less than what they are—"technical tools" to be applied tactically where needed to drive defined results, and in full recognition of their demands and limitations.
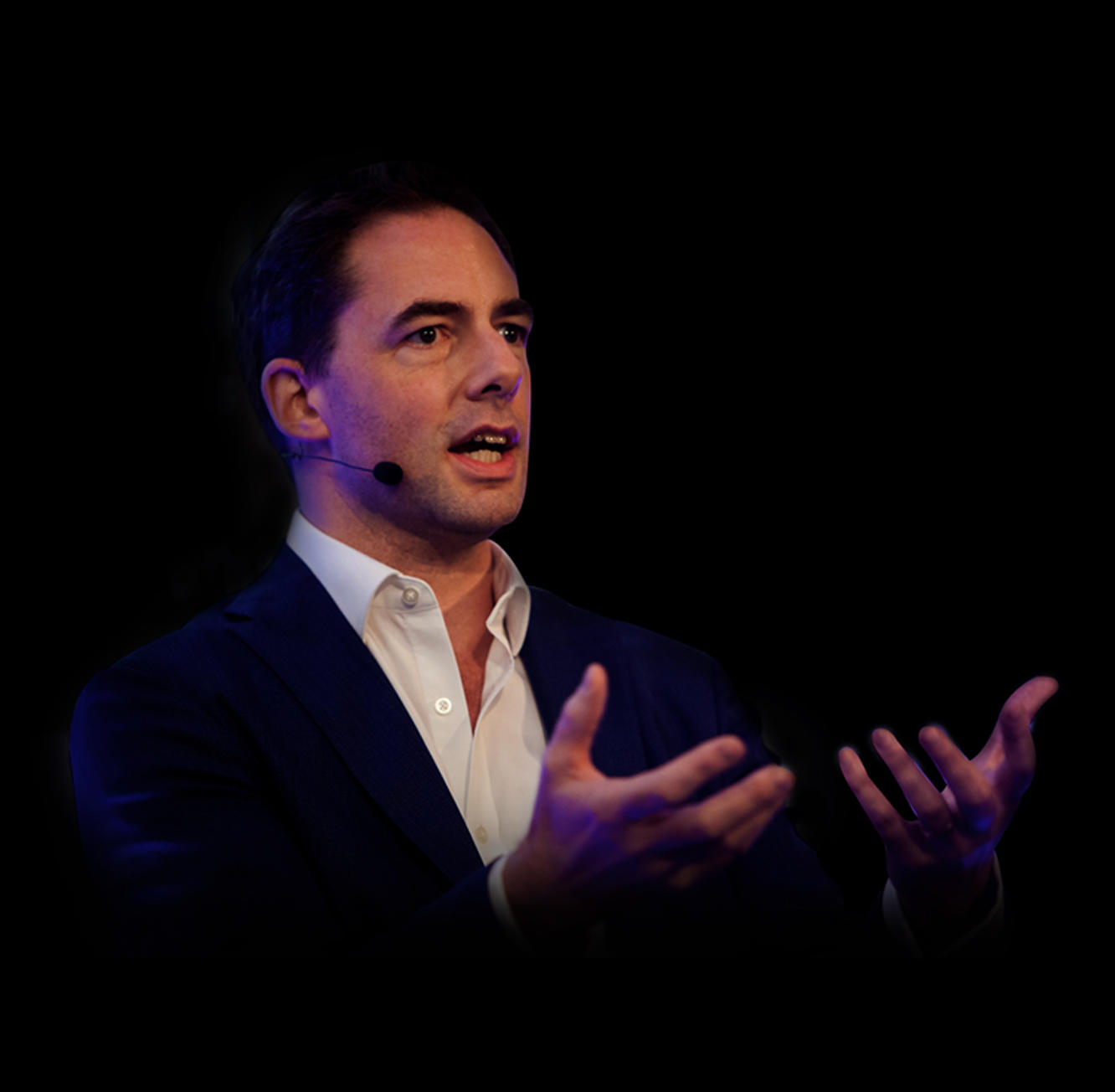
"It's common for people to say ‘I want a data lake’ or a ‘modern data architecture’ but have very little idea what for."
Mark Brand, Independent Consultant
“It's common for people to say 'I want a data lake,' or a 'modern data architecture,' but they have very little idea what for,” Brand said. “It’s common because those people often have two characteristics—they own data and data architecture, but they don't own any decisions or any outcomes. The most common result is people attempting to build data lakes like you build a data warehouse. At first they try to put everything in there and tag it, and then they give up because that's a bit tough. So they try to put everything in there from one of their monolithic critical systems and capture all states; so if the quality of a data point changes every 60 seconds, they try to somehow capture and tag that, before they've even worked out why they need to hold that information."
Similarly, while many enterprises are keen to adopt machine learning, Ken Collier, Author of Agile Analytics, notes "not every problem needs a machine learning solution. Some are best suited to just writing some standard software or some other code."
"AI by itself is a huge subfield in computer science that has a whole lot of different disciplines," Collier said. "I get bothered when I hear business leaders using machine learning as some sort of buzzword, when what they really mean is 'We want to do things in more leading-edge ways.'"
The immediate risks of businesses applying data-driven solutions for their own sake are clear enough, including non-performing projects and wasted resources, but Collier believes the impact could be even broader: "What I fear is that we're headed toward the next AI winter. After all of this hype wears off, and only some things have stuck or have proven value, all of a sudden AI is going to get a bad rap for not having lived up to some unrealistic expectations."
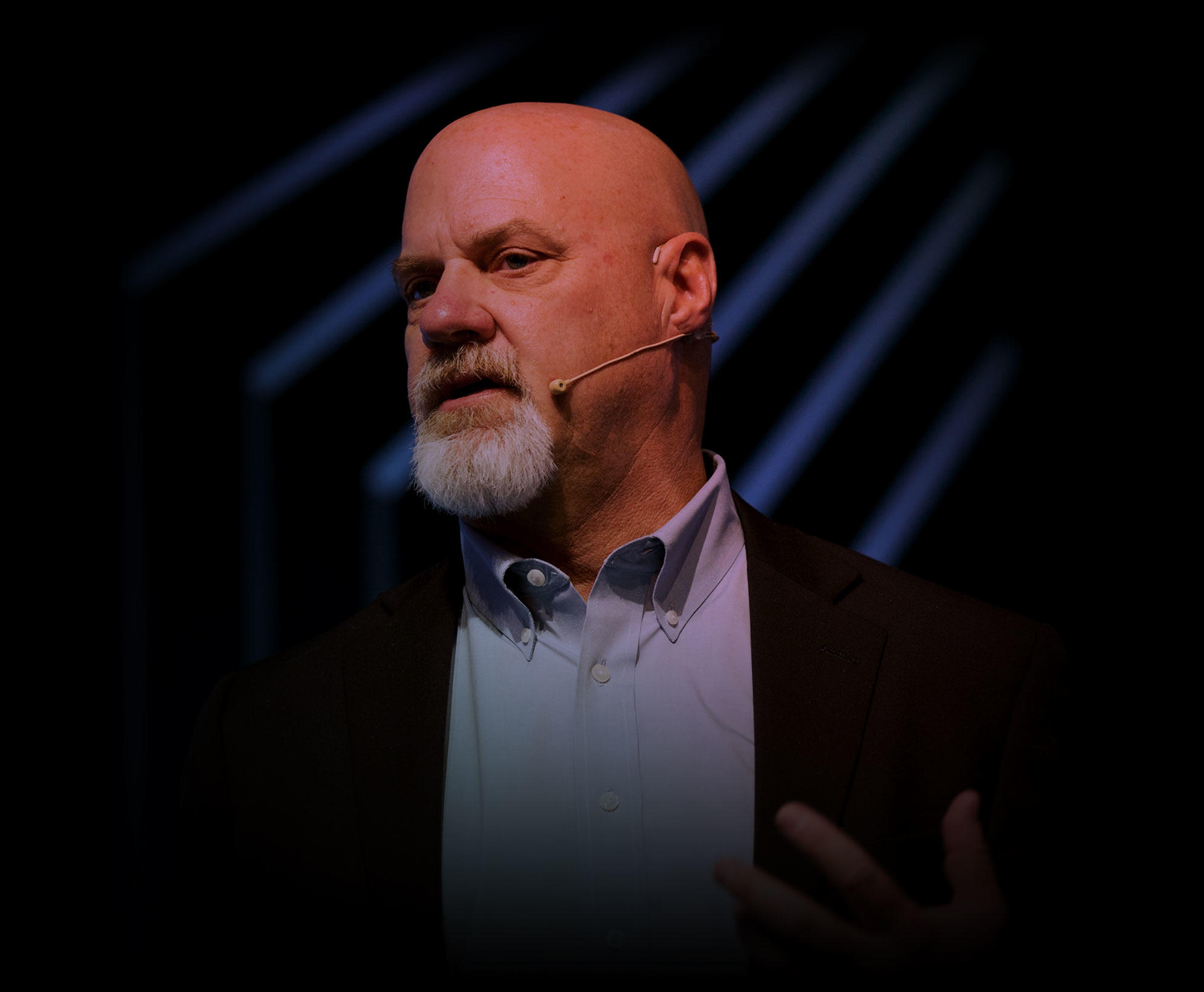
“What I fear is that we're headed toward the next AI winter. After all of this hype wears off, and only some things have stuck or have proven value, all of a sudden AI is going to get a bad rap for not having lived up to some unrealistic expectations.”
Ken Collier, Author of Agile Analytics
When faced with failure, the tendency is to blame the tool at hand. "Most data projects do not fail because of technology, but because of human factors," Windheuser said. "There may be a new [method of] organization necessary, or resistance to change, or no data processes defined." Which brings us to our next principle.
Principle 4: Culture and design are just as important as tech (if not more)
The data hype has many companies rushing to hire data scientists and set up bolt-on departments that focus on data and data alone. This has created an acute shortage of data scientists in key markets as cutting-edge AI and machine learning skills become increasingly sought after across all industries—LinkedIn estimates a shortfall of over 150,000 in the United States alone.
Data scientist shortages in the US by city
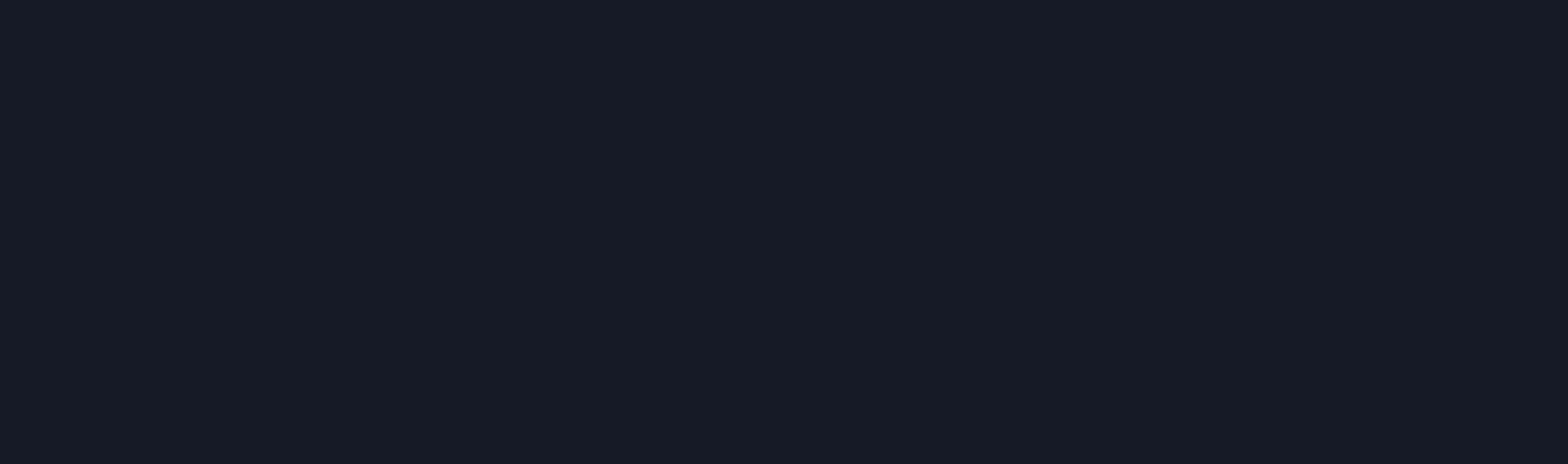
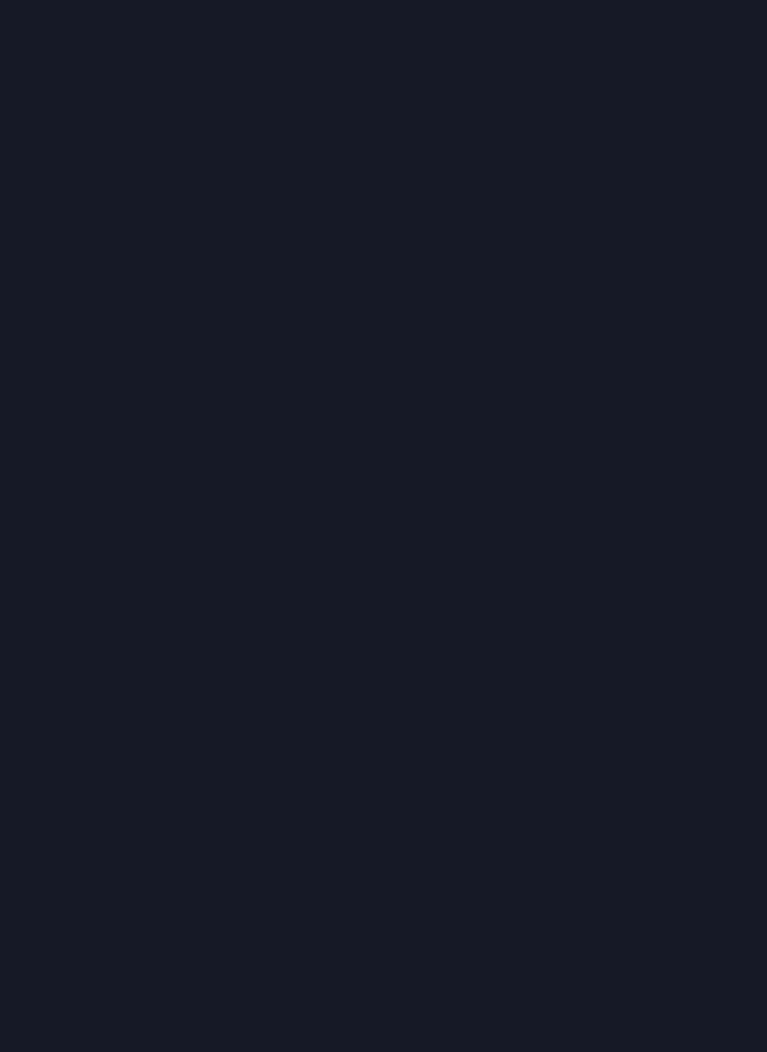
Given the need for data initiatives to arise from and maintain alignment with the business, a dedicated hive of data scientists may not be the best approach. As Hadaway points out, "Hiring data scientists will not make you successful. The rest of the organization also needs to know how to use data."
"People have been hiring up data scientists like crazy but don’t really know where they fit in the organization, so they park them in a shared services team, or maybe as part of the marketing or finance department," Collier said. "The data scientists sit in their silo, not really interacting well with the business to figure out what's important and what problems they’re challenged with."
Enabling these interactions and generating business-ready insights requires not only an open data architecture, but also cohesive teams. To work well with data, teams need to be driven by a shared charter and defined outcomes, and include both technologists and business leaders.
"You have to be willing to break down those walls between IT and business and structure cross-functional teams that are chartered to build products," Collier said. "Then you have to put the right people on those teams, not only to do advanced machine learning, but also business experts who can say ‘Yeah, this machine learning matters, and this machine learning model actually does something useful and meaningful.'"
This process works both ways. Just as data scientists need to grasp business goals, other parts of the business need to recognize that data is a shared resource and responsibility. Basic elements of data governance, such as defining who has ultimate ownership over and access to data, are typically established and managed from the top down. But people in every business line should be examining data generated in the course of daily operations to determine the role it might play in decision-making and ensure consistent standards are maintained.
"This is what we call data democratization," Windheuser said."Everybody is able to utilize the data, but everybody is also responsible for cleaning it, for taking care of it, so the quality is there and it’s up to date. It’s like a family living in a house."
This philosophy should extend to the wrangling and organizing of data so it’s fit for business purposes. This is a decidedly unsexy task, often referred to as "munging," and typically reserved for technologists.
"Business users have to be involved in the process because they’re the ones who understand why something is dirty," Parsons said. "You also need technologists, hopefully with a long history in the organization, because particularly for data that’s been around multiple years, there are all kinds of traps. So you need someone who understands the history of the systems, but also somebody who understands what the data actually means in the business context to resolve the problems that come up." As outlined in the latest edition of Thoughtworks Technology Radar, new data tools and innovations are just more buzzwords in isolation. What spurs progress is figuring out how to meld these data innovations with enduring engineering practices in well-defined teams.
Principle 5: Start small, learn, repeat . . .
Big data may be fueling the appetite for large projects and solutions, but investing everything in a single massive implementation or sweeping transformation initiative carries a correspondingly large risk of failure—not least because when it comes to disciplines like AI, organizations have little in the way of prior experience or benchmarks to guide them.
It’s important to see being data-driven as a process or journey that involves incremental steps, rather than a destination or something that is "done." "Think big," as Windheuser puts it, "but start small and with something that is feasible."
Of course, the steps must be the right ones. For many companies who are tackling machine learning or data science, the temptation may be to begin with a proof of concept (POC), but these aren’t designed for production, so rarely advance past the POC stage. “In the end it will never be proven that a POC has an advantage for the company, and it will never really get acceptance,” Windheuser said.
Better to start with a minimum viable product. Create a "little use case" that has immediate utility to a client or end-user and implement it with a larger application or project in mind.
Data initiatives have the best chance to flourish when mixed with agile development practices like continuous delivery and integration. Something that can be rapidly deployed in a productive way "will generate value and be really convincing for the whole organization" Windheuser said. "All you have to do is continue the journey."
Choosing the right steps requires knowing where you’ve been, building on your past successes (and failures) with data, and knowing where you’re going.
In a data-driven enterprise "the way of working becomes critically important to achieving outcomes," Brand notes. "We want things to be always versioned and saved so we can review the decisions we’ve made. Even if it’s on a piece of paper and decisions are made once a month, we want to know what the data captured was, how it’s been transformed, where it’s going, what action has been taken, and what the impact is to be understood."
In this way, the process of becoming more data-driven is itself driven by data that informed decisions from past iterations.
… and don’t ever stop
Rather than algorithms or cutting-edge analytics platforms, it is this discipline of constantly building and testing, as well as collecting, analyzing and acting on results that characterizes the data-driven enterprise.
By incorporating insights from one iteration to the next, products or solutions are in a state of perpetual improvement. In other words, continuous intelligence includes continuous delivery principles to minimize the data-information-action cycle. Agile has taken on all kinds of meanings in recent years but is best defined as the ability to complete this cycle quickly and seamlessly. This is an area where many enterprises still struggle.
Cycle of Intelligence
Combining agile and continuous delivery with data science
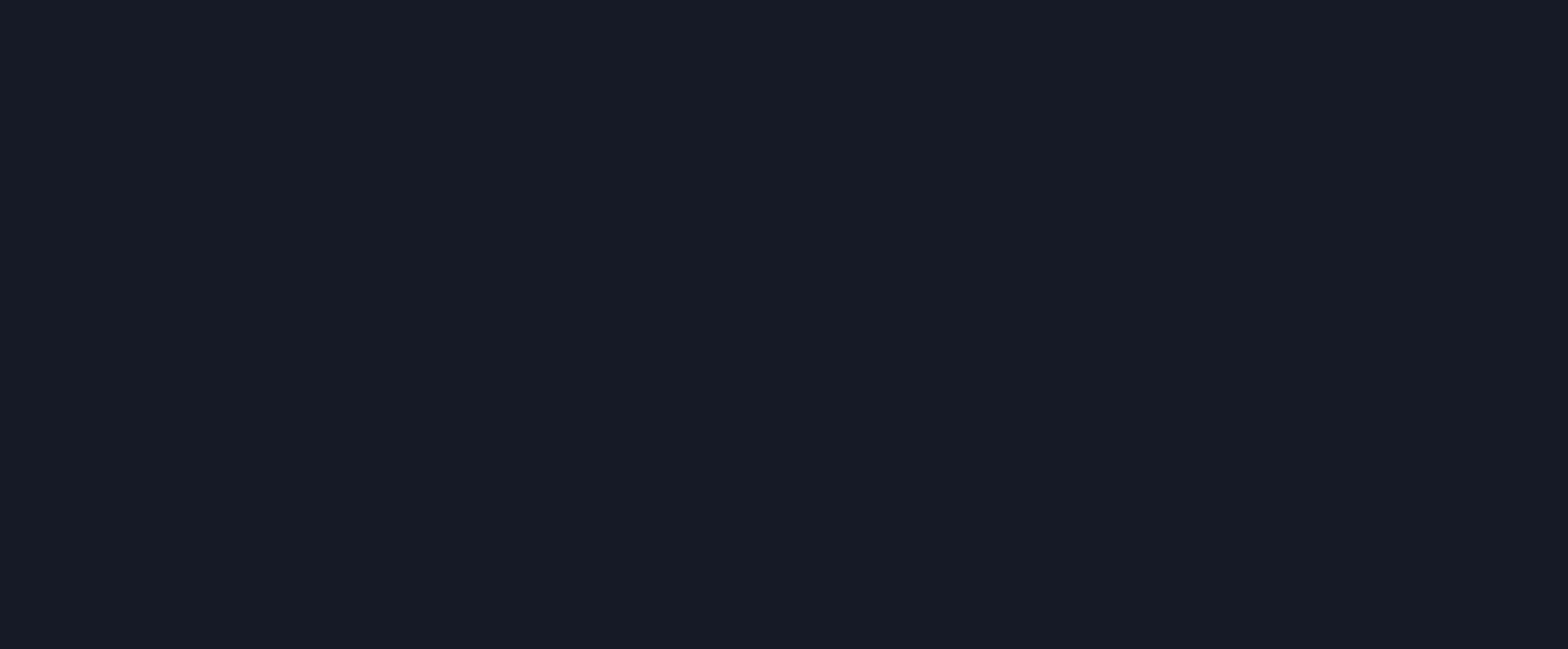
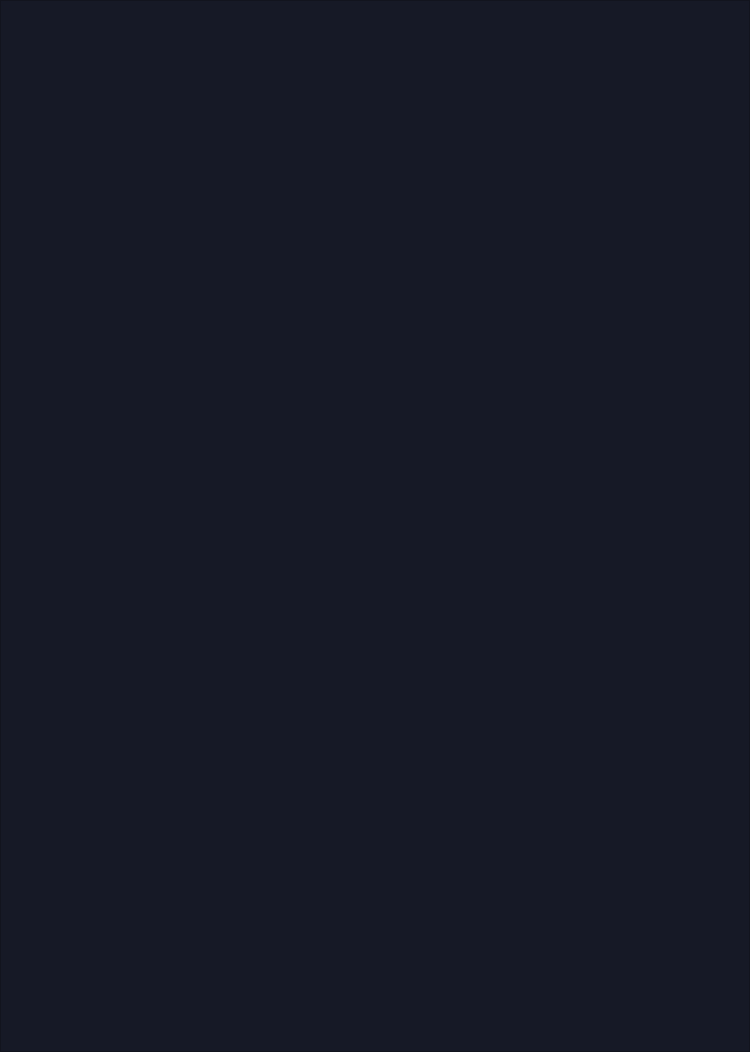
"This circle of getting data, extracting information, getting insight and knowledge, then transforming that into decisions and actions in a lot of organizations takes months or years," Brand said. "That’s not agile. In many cases this is down to an isolated data science team working in an isolated environment on the data and the data models. They’re starting from scratch to develop a scalable and productive user application which is given to operations to integrate and run in the IT environment. Continuous delivery allows you to reduce the whole cycle to days, so it’s more or less automated."
Data’s role in that process (and in agility) should be recognized and celebrated. Data and the fast-developing technologies that harness it are closer to building blocks than a commodity worth hoarding.
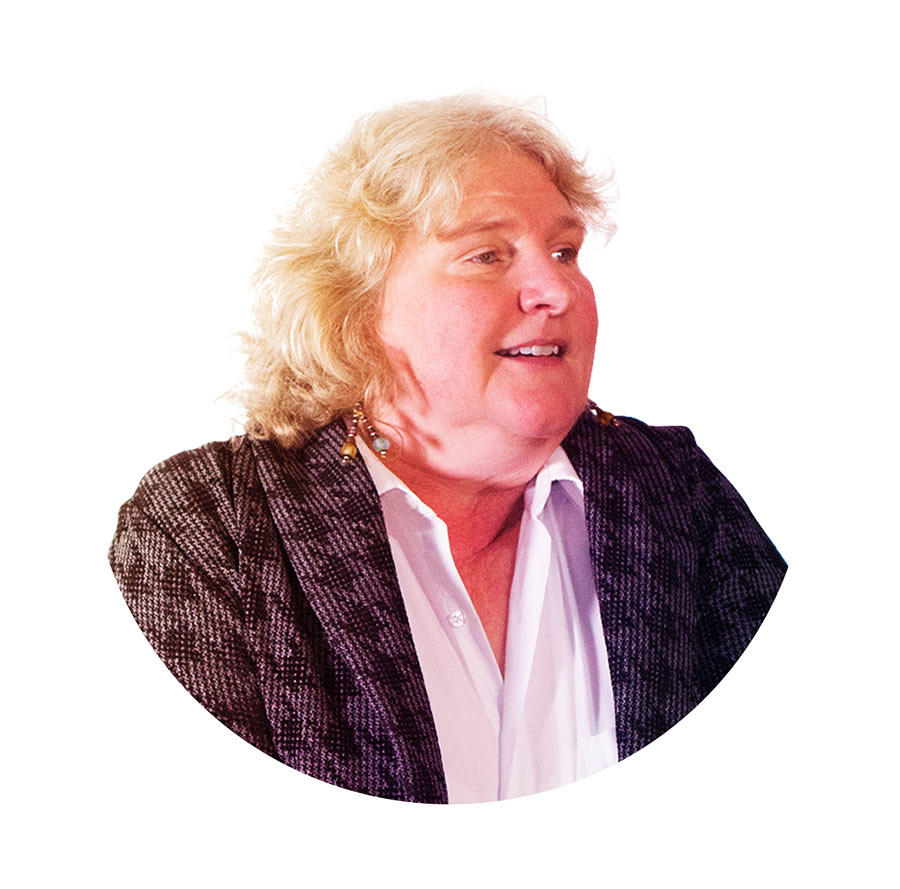
"With any new thing, business leaders feel that they need to go now and invest. But if you invest in isolation, you tend to miss the whole point. Data is just another component, rather than the only thing that you need to do to win in business."
Dr. Rebecca Parsons,
Chief Technology Officer, Thoughtworks
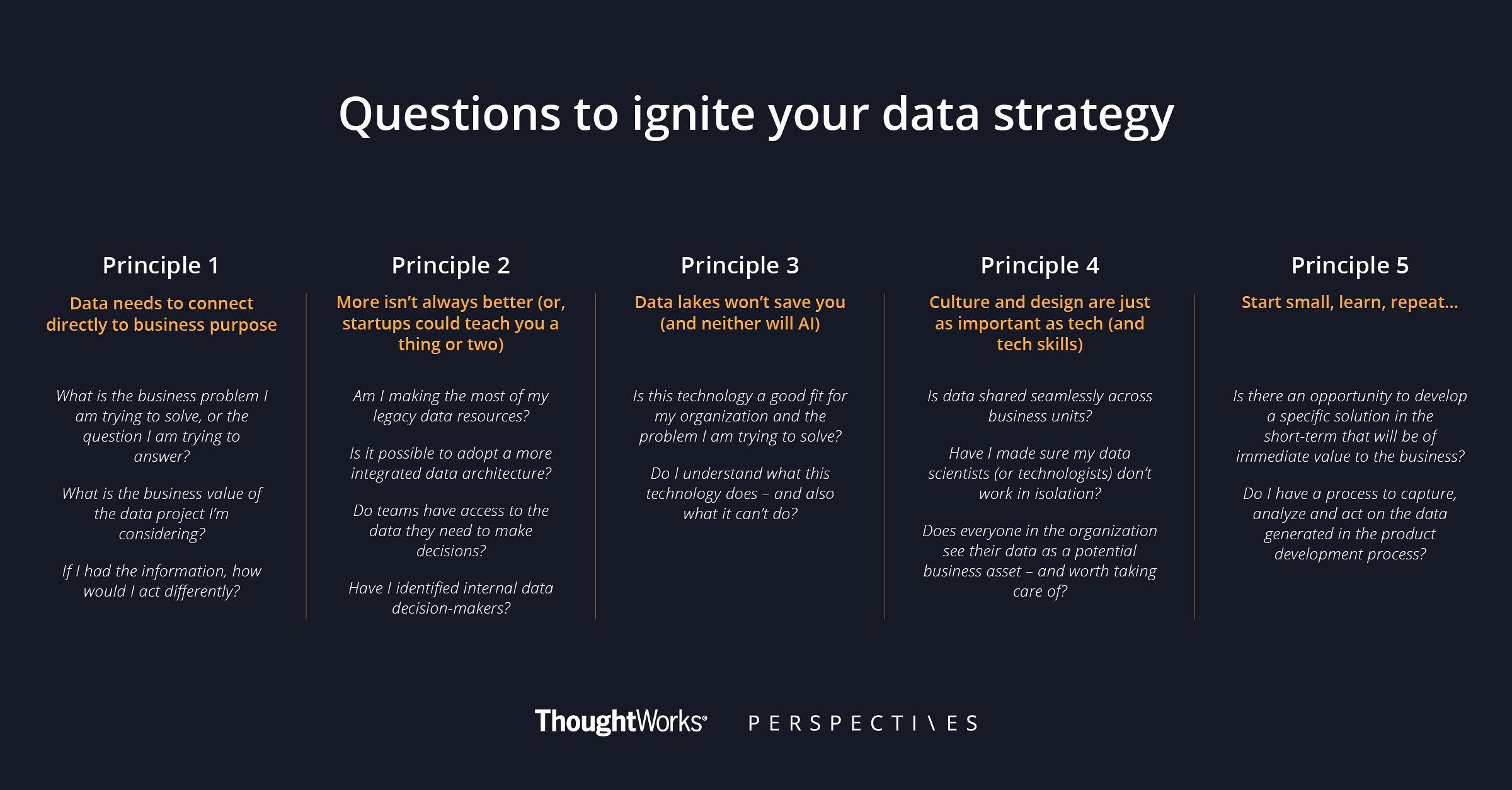

Perspectives delivered to your inbox
Timely business and industry insights for digital leaders.
The Perspectives subscription brings you our experts’ best podcasts, articles, videos and events to expand upon our popular Perspectives publication.