Glovo is a multicategory delivery platform with presence in more than 1500 cities in 25 countries, and since mid-2022 is part of Delivery Hero. It is the fastest-growing multicategory player in Europe, Western Asia and Africa with technology at the core of their business. Glovo has created a four-sided marketplace formed by:
Customers, the people who use Glovo to get something delivered to them
Partners, local businesses whose goods Glovo delivers to customers
Advertisers, a special type of partners which pay to get promoted in the marketplace
- Couriers, the people who deliver to customers
Glovo aims to create shared value to all the agents in this marketplace, for which data is a critical asset which comes with its own challenges inherited from the very nature of Glovo’s business. But no matter how big Glovo could be, these challenges should not be an obstacle for Glovo’s needs of trustworthy data to achieve their business goals.
The history of data at Glovo
Glovo has always been a data-driven organization. From its beginnings, data roles have been across all business units and the data community is widespread in the company. The combination of the extensive use of data and the hypergrowth which Glovo has experienced for a long time, has had consequences to their data assets. Over time, data was becoming increasingly dispersed, eventually reaching the point where each business unit was doing their own analysis of the data they had available, normally in a very ad-hoc manner. In addition to this, there was no common quality bar for data. Even though each unit was doing their best efforts to keep their data in the best shape, it was easy to see the differences in the data quality each unit could provide.
To make things more interesting, Glovo is on its own journey to move from the monolith it started with, to a more distributed architecture. New coarse-grained and micro services coexist with the original monolith, which still sustains an important part of the day-to-day business. This transformational journey adds extra complexity when it comes to identifying data sources.
In this context, data engineers had been stuck between the operational and the analytical data planes for a long time. The main issues they regularly raised were:
No trustworthy data sources.
Lack of quality standards for data.
Questionable availability of data.
Misaligned or unknown ownership of data.
Difficulties to discover useful data.
- Requirements to involve multiple teams in collaboration to create new services which heavily relied on data.
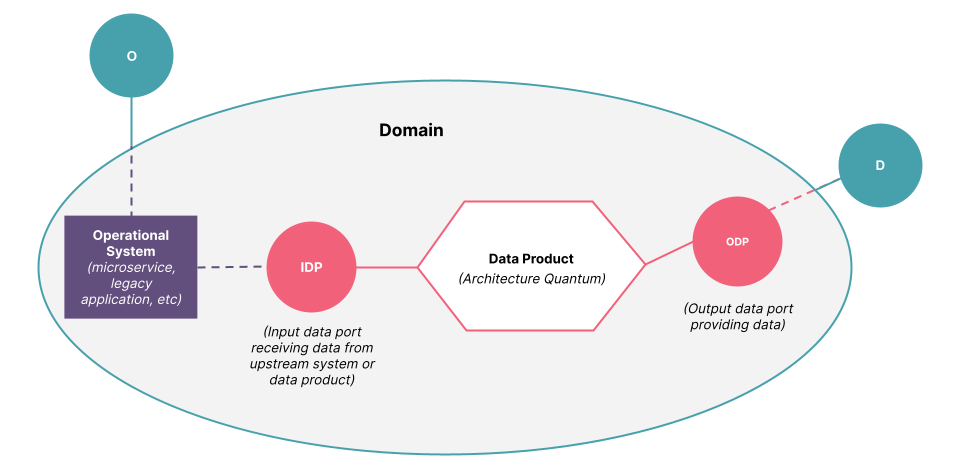
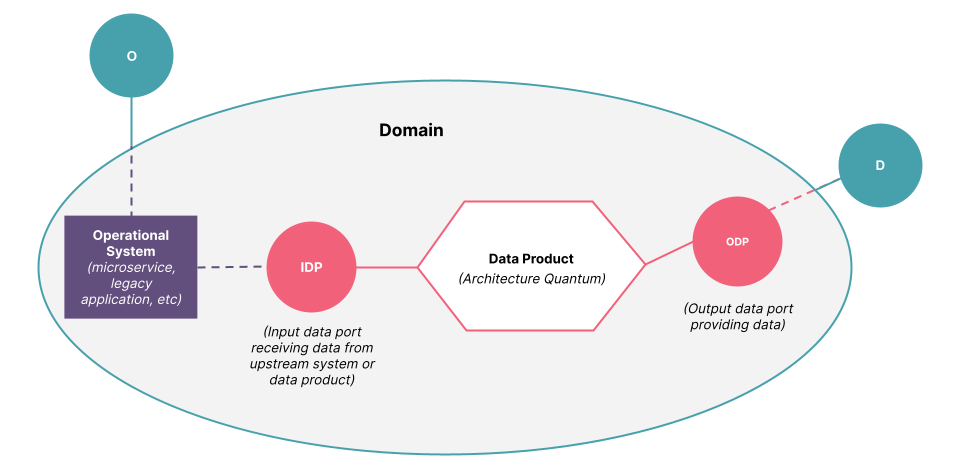
For a company like Glovo, these issues were well beyond technical problems and posed some important threats. On the one hand, the company was at risk of making decisions based on no data (flying blind) or, even worse, being confident about decisions which were made based on incorrect data, only to find out when it was too late. This was making stakeholders lose their trust in the data which was provided to them. On the other hand, the critical tech talent that Glovo had been growing over time was feeling more frustrated with the situation, and the risk of having a big churn on this talent was more present than ever.
A new data strategy is born
Glovo partnered with Thoughtworks to develop a comprehensive data strategy which could address the previous challenges. With the engagement of executives, data and software engineers and product managers, a special task force worked on analyzing the situation and making a concrete proposal to move forward. After four weeks of deep diving and exploring the problem space with multiple parties, the team considered that Data Mesh was a useful approach to tackle the challenges Glovo was facing. In this particular case, there were four specific reasons which led to making this decision. The fact that Glovo already had a very decentralized ownership model for its business was well aligned with the general philosophy of Data Mesh to decentralize data ownership, and data profiles being already present in each business unit could make the transition easier. Glovo was already a product-centric organization, which lowered the barrier to start thinking of data as a product, too. Lastly, Glovo needed to keep the freedom the business units had been successfully leveraging so far, while providing a common framework to ensure data quality.
Among the four principles of Data Mesh, the focus on product orientation and the federated governance model were the ones that resonated the most to consider it part of the new data strategy.
Bringing Data Mesh into reality
The first steps in the journey of implementing Data Mesh at Glovo had more to do with cultural change than technology. It involved shifting from thinking of data as tables to seeing it as the combination of multiple elements, like code, infrastructure, metadata, etc. that has to meet the expectations of usability, feasibility and value for its users.
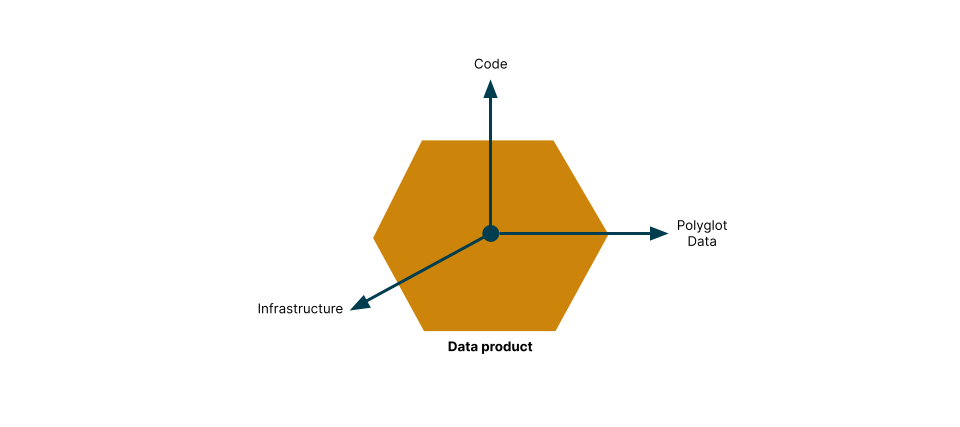
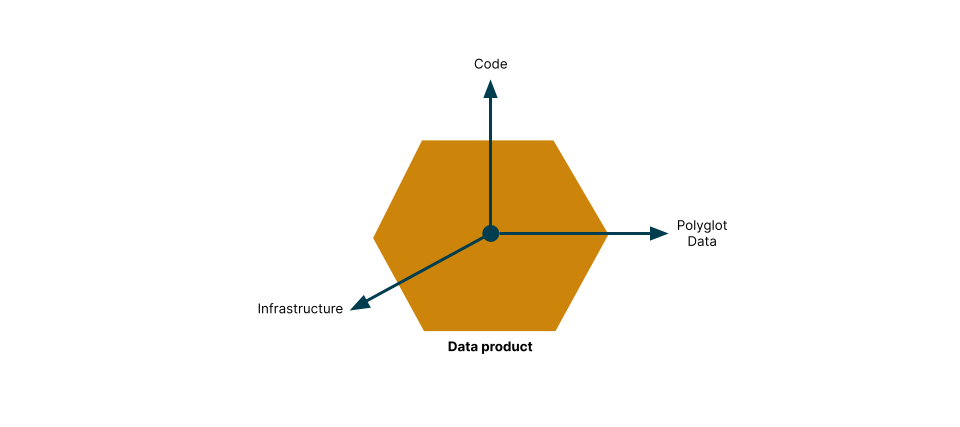
The team needed to see concrete results sooner and minimize risk while building the elements of Data Mesh, so they took a thin slice approach: from the initial list of potential data products which came up during a discovery phase, the team prioritized them based on business value and chose one. That one would be built alongside the minimum necessary elements of the data platform and the federated governance model to make it work. This data product, called Customer Behavior, provided a complete vision of the app conversion rate funnel (CVR) which would enable stakeholders to analyze and understand the customer journey –and it would also feed into other data products that would be built later. After the team completed the Customer Behavior data product, other data products would follow, and each slice would add new elements of the operating model, data platform, federated governance mechanisms, etc.
During this phase, the biggest effort went on building the data platform which would enable self-serve capabilities, as Data Mesh advocates for. The first slice produced, in addition to the first data product, an MVP of the necessary data platform, which included:
Self-service data pipelines, for data ingestion
Processing capabilities
Security policies
Query engine, for data consumption
The new strategy also brought changes to the organization with new types of teams which would support its implementation:
Embedded teams would include product analysts, data engineers and (potentially) data scientists. They would produce data, models or metrics to be consumed only by their own team. This type of team was more of an intermediate state, as they should eventually transition into another type of team –the data product team– in order to benefit from the new governance model and platform capabilities.
Data product teams would work on creating data products which could be consumed by multiple teams and provide high levels of data quality, robustness and performance.
Data platform teams would be responsible for building technical capabilities into the data platform as data platform products, which could be used by the data product teams. They would not own any domain specific data product.
Enablement teams to help other teams to adopt the new approach and use the tools provided by the new platform.
Finally, there could exist special purpose teams to be created on demand.
Lessons learned, challenges and what follows
Glovo is still in an early stage of adoption of Data Mesh, and the rest of the industry. Taking one step at a time and learning continuously from the experience has been a fundamental part of the process. One of the most complicated activities during this journey has been keeping a balance between opposing forces, for example, the time needed to fix immediate problems versus building the necessary platform. Or, at the organization level, explaining Data Mesh and creating awareness about it, while, at the same time, managing the high expectations that were being created around it.
After delivering the first data product, many of the fundamental building blocks which were needed to accelerate the development of new ones were in place. The next steps include the creation of a comprehensive catalog of data products, built on a prioritization based on business value and considering the analysis of the potential dependencies between them. This will be combined with a deeper exploration of the team structures that would best support the implementation of the strategy. Finally, the focus will now move to push for Data Mesh adoption outside of the boundaries of the tech organization. And this last point will be fundamental to realize Data Mesh’s full potential and get all the benefits it can bring.
In part two, we examine the challenges we faced as we continued the journey, as more domains created data products and the complexity began to increase.