Collaborators: Dr Yogesh Wadadekar, NCRA-TIFR
Prediction of astrophysical properties of galaxies uses flux measurements from ultraviolet bands to far-infrared bands supplied by the GAMA survey. The current approach is about comparing millions of templates from population synthesis models to find the best-fit model for each galaxy. The state-of-the-art MAGPHYS technique takes about 10 minutes for each galaxy. As evident, this is too slow a process even when automated. To overcome this issue, we used deep learning, a machine learning based approach that learns from the survey data and the properties predicted by the MAGPHYS model, and then predicts the parameters such as the star formation rate, the stellar mass, and the dust luminosity of each galaxy. The deep learning technique predicts much faster than the MAGPHYS approach. For example, MAGPHYS would take about 2.5 months to estimate parameters about a million galaxies, while our model can do it in a few seconds. In addition, being a data-driven technique, it can learn from new survey data, and predict results with relatively less involvement from the scientists. Given the advent of new state of the art telescopes under way, which can look deeper into the Universe, this saving in time becomes all the more important as these parameters can be quickly estimated for the enormous data about galaxies that will be collected by these telescopes. This work has been published at the Monthly Notices of the Royal Astronomy Society, and can be found here.
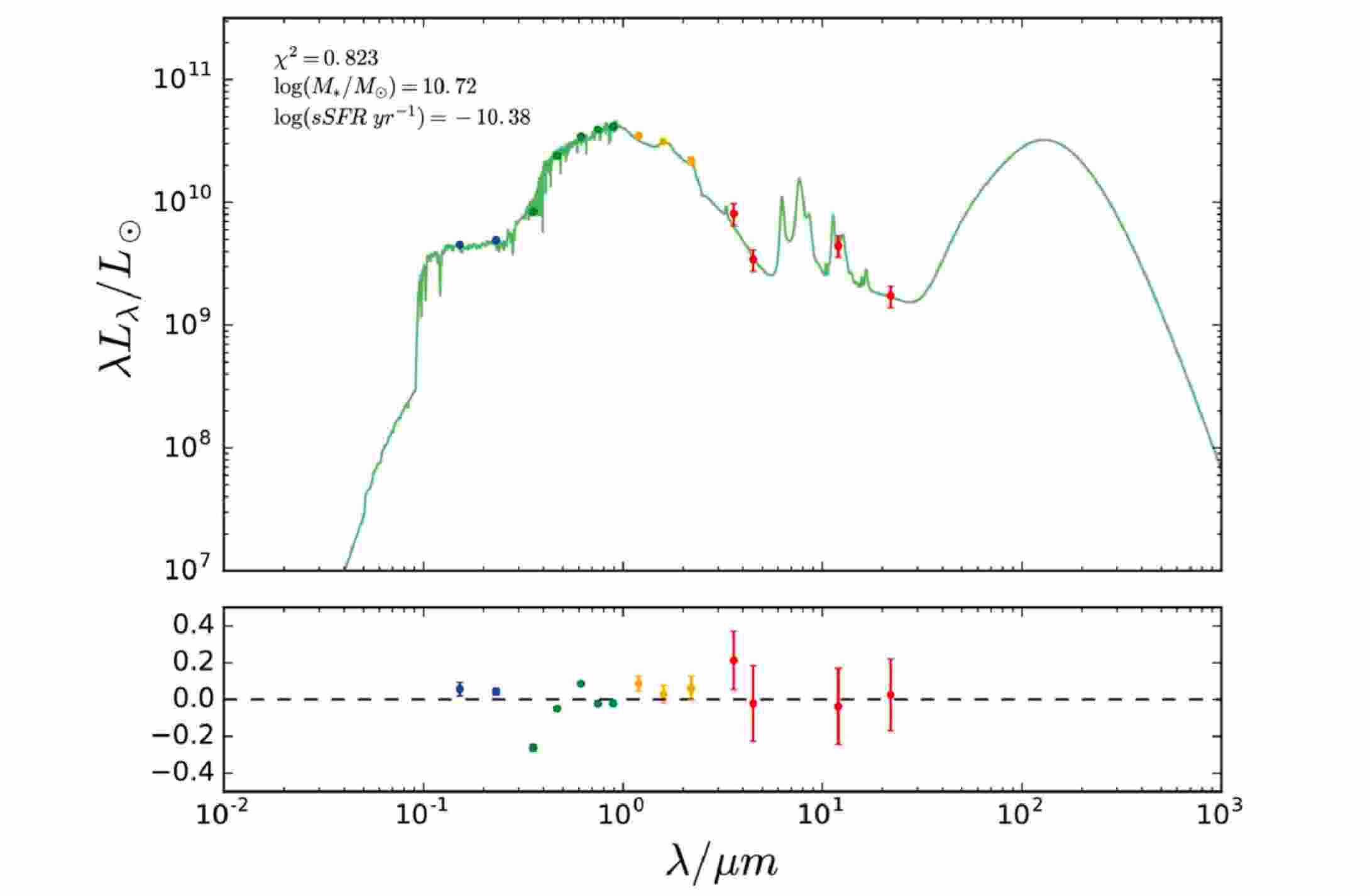
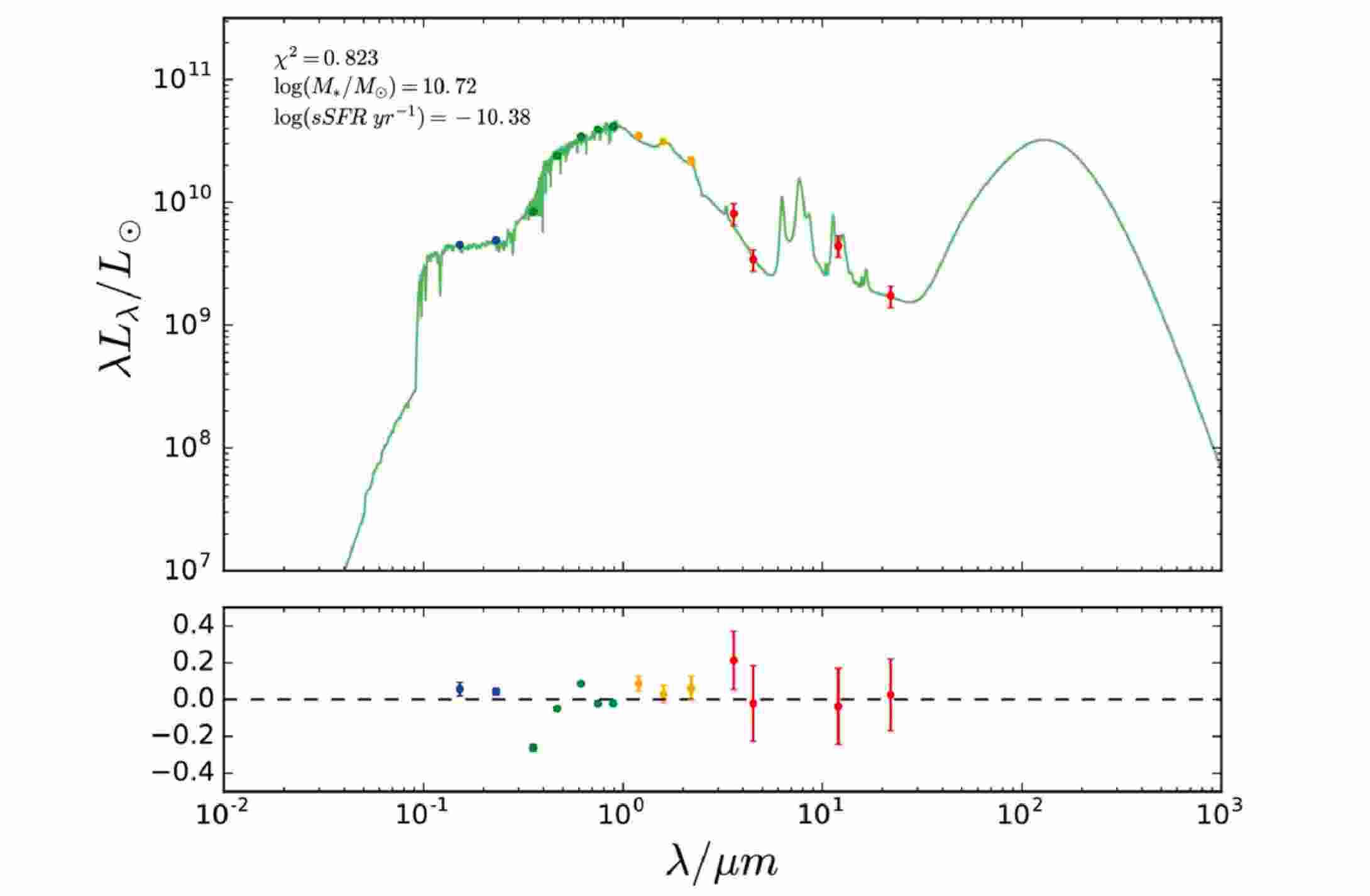
The figure above shows the flux of one galaxy as a function of wavelength in microns. The flux measurements are shown by 14 data points plotted in various colours and the green curve is the best fit stellar population synthesis model. The main free parameters in the fit are related to the star formation history of this galaxy - how many stars did the galaxy form at various points in the past.