You might start this article with the question “What is the most difficult problem facing organizations right now?” Given that organizations come in various shapes and sizes, across a wide range industries, it makes sense that they will face very different challenges. But there is one key challenge that they all have in common: the ability to make and execute decisions.
Organizations are, at their core, decision engines. The average Fortune 500 company makes 400 million decisions a day. Granted, some of those decisions will be “What should we order for lunch?”, but the majority are critical business decisions. These will range from day-to-day operational decisions that keep the company running, right up to the long-term strategic decisions made by the board to determine the future of the business. It’s great when all these decisions turn out to be right, but can be costly when they don’t. Poor decision-making costs the average Fortune 500 company around $250 million every year, just in wasted time for managers. That doesn’t include any of the other losses such as opportunity cost, reputational damage, regulatory fines, or wasted development and R&D investments.
In fact, the ability to effectively make and execute decisions has been shown to be the strongest indicator for company success. If companies get it right, they produce on average 6% higher returns for their shareholders. The same research also revealed that 4 out of 5 organizations surveyed globally were making and executing decisions ineffectually, highlighting the extent of this problem. There is tremendous value to be gained in getting it right, with top performers clearly separating from the pack, but why is it so hard?
In this article, I will explore some issues blocking companies from making better decisions, how we can use technology to solve them and how such an approach can deliver successful business outcomes.
The issue is complexity
It was recently found that 65% of executives feel the decisions they make today are more complex than two years ago. What's more, this increase in complexity is set to continue, if not accelerate. As humans, we don’t have the capacity to keep track of all the interacting elements involved in these decisions, let alone make sense of them. In recent years we have enlisted a plethora of rules, algorithms and AI to help us identify patterns from previous scenarios, providing predictions about what might happen next. This approach, however, has now also been outstripped by the rapid growth in complexity, and here’s why.
Described scientifically as a ‘complex system’, today’s business environment has three key characteristics; it’s non-linear, emergent and intractable. Crucially, this means that while it might look predictable with hindsight, these characteristics make it impossible to predict outcomes in any meaningful way based on just what you’ve seen in the past. As a result, we can’t use AI in its traditional form, harvesting all the possible data about a problem before training a model to act based on hindsight.
Which poses an interesting question for an article about AI. How can we use AI to make decisions more effectively then? Well at Thoughtworks, we believe it starts with how you frame the problem, and we aren’t the only ones who think that.
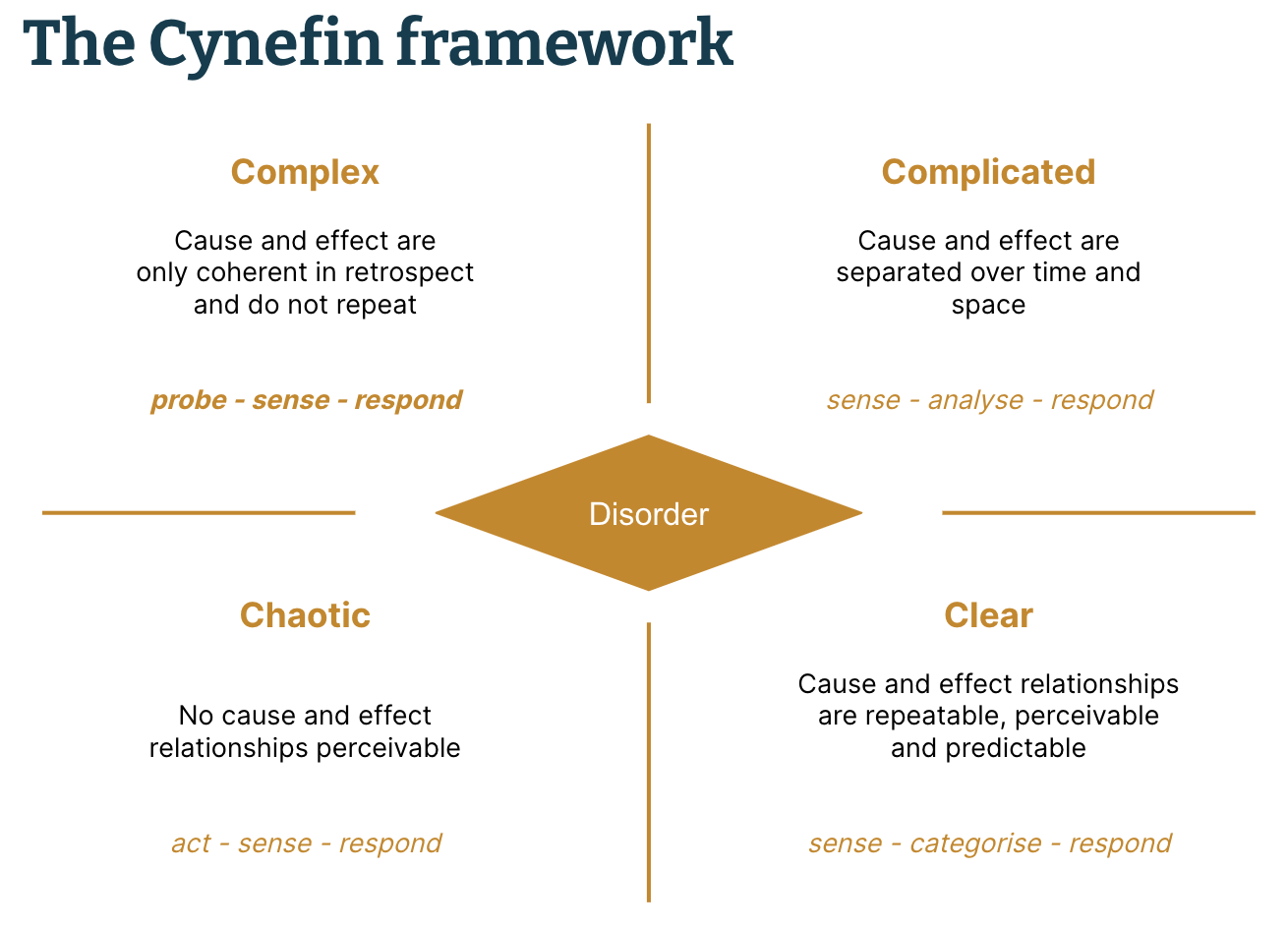
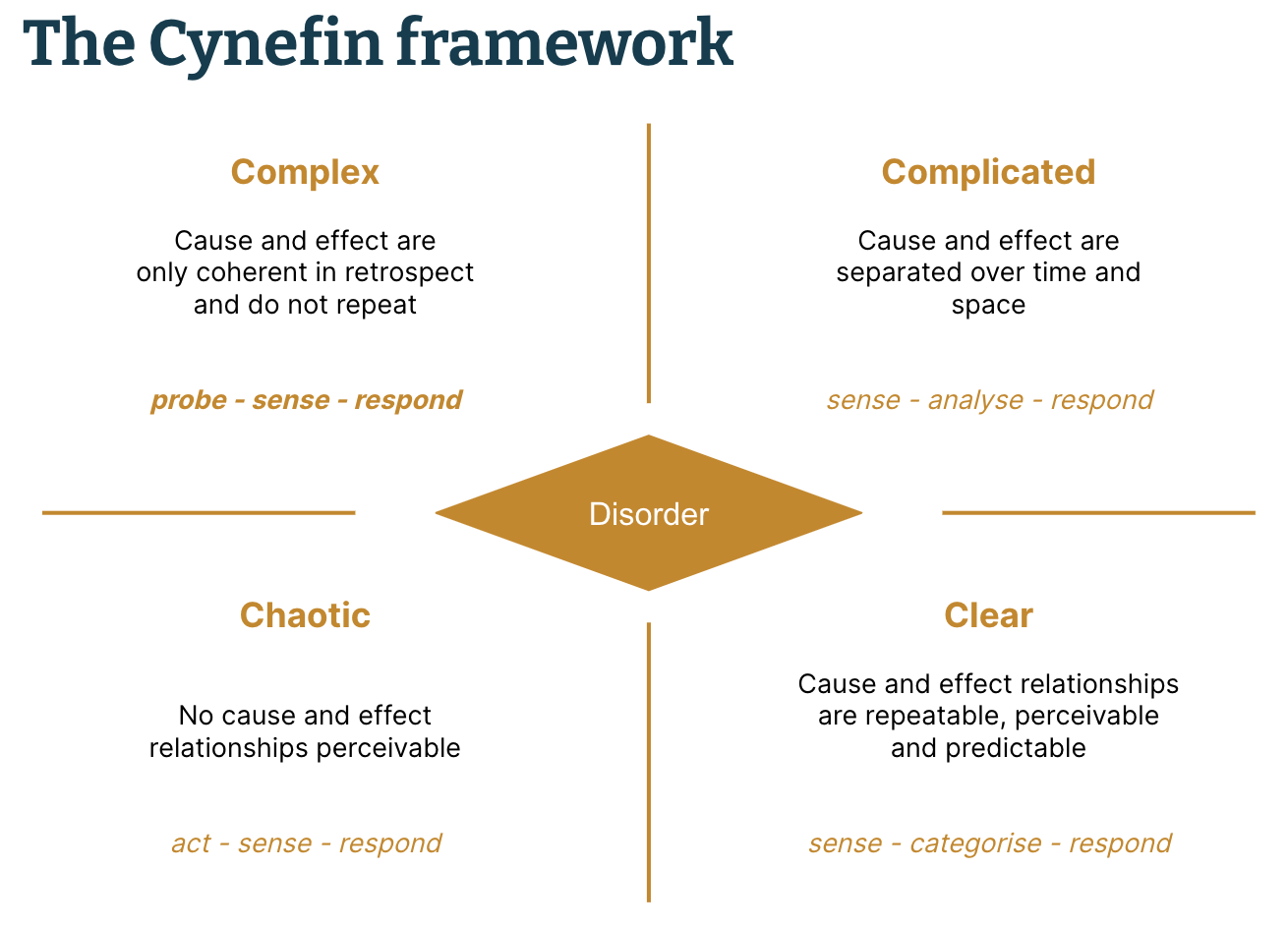
The Cynefin framework is a decision-making framework for leaders operating under uncertainty. Originally proposed in this Harvard Business Review article, it places decisions in one of five domains: clear, complicated, complex, chaotic and disordered. Crucially it tells us how to approach decisions in each domain. Our focus is the complex domain, and there is a quote from the article that sums up the approach for complex problems:
“Instructive patterns, however, can emerge if the leader conducts experiments that are safe to fail. That is why, instead of attempting to impose a course of action, leaders must patiently allow the path forward to reveal itself.“
So while complex systems aren’t predictable, we can run experiments to discover valuable cause and effect relationships that will guide our decision-making. This is where AI comes back in. Not only can we use it to help us find these relationships, we can use AI to identify which experiments will yield the most instructive ones.
Analytical systems, therefore, need to be built in a way that facilitates this process. The problem is this is not always the case. Most analytical systems are built with the sole intention of optimizing for a business metric, not conducting experiments. For example, in the retail space we often focus on increasing sales, but not on understanding the customer. Asking “What product will the customer buy next?” is very different from “What are the drivers behind customer wants & needs?”, “How long will those drivers last?” and “Why might those drivers change or disappear?” These are not mutually exclusive pursuits – our approach can both optimize for a metric and learn about customers - the key is in uncovering those cause and effect relationships. So let's delve into what such an approach might look like.
Revealing cause & effect relationships
A key sticking point to the complexity is the intractability of the problems faced. Situations have incredibly large numbers of elements, as well as confounding effects due to interactions. In order to build a system that enables us to explore cause and effect relationships between elements, we need to be able to constrain some of the factors by breaking down the relationships into more manageable components.
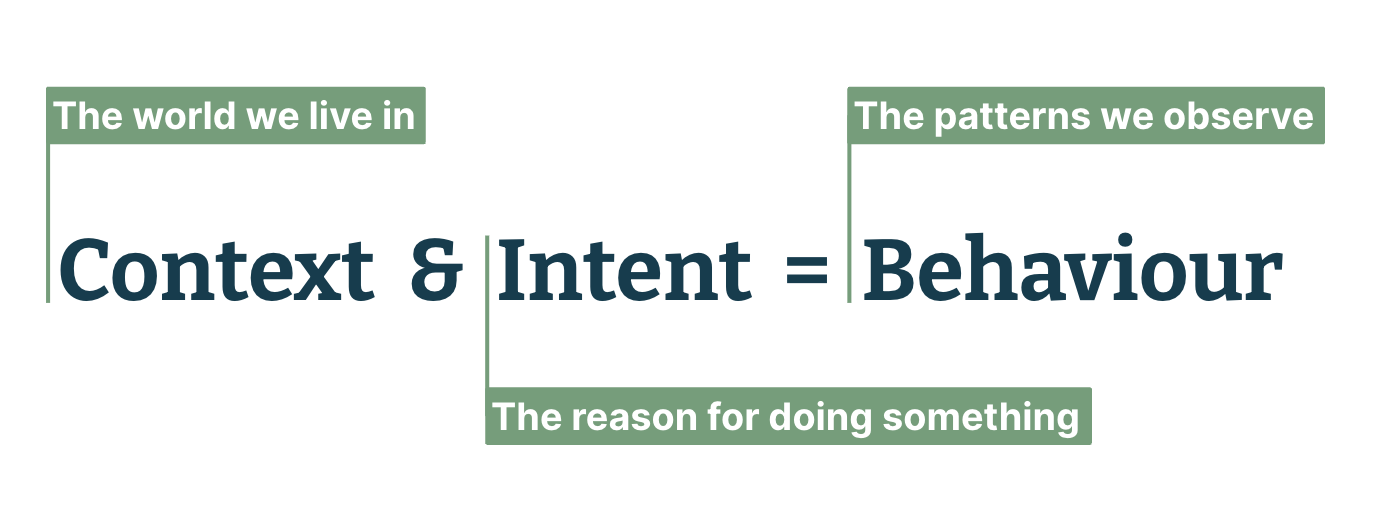
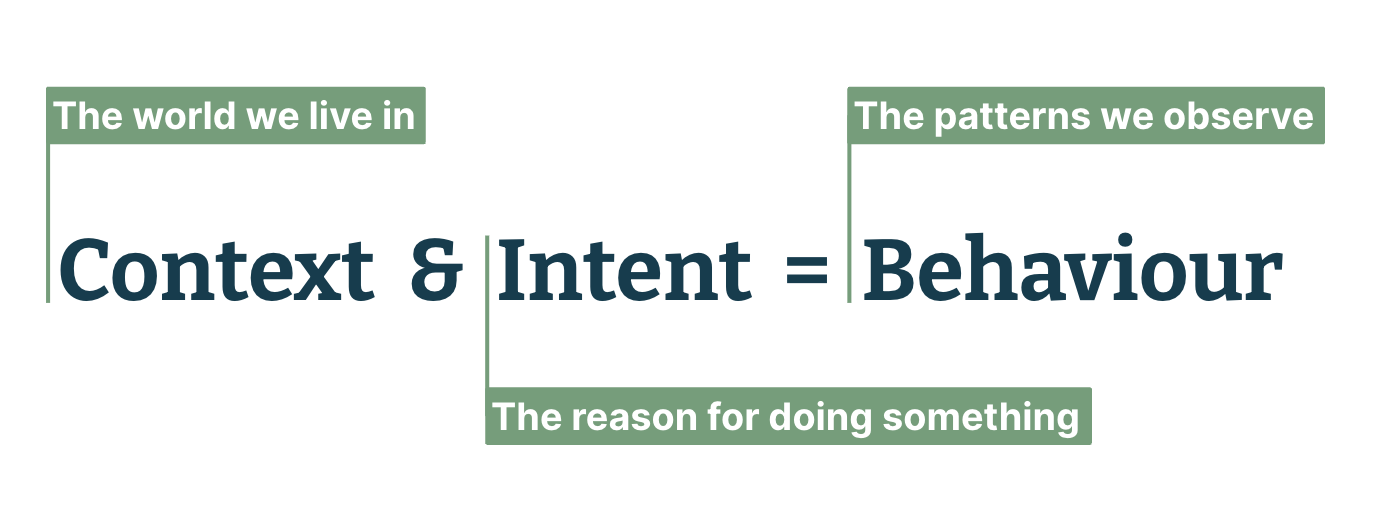
The diagram above is a very simple representation of cause and effect. Cause on the left, and effect on the right. Cause is made up of two parts: the context in which something happens, and the intent behind it. The organization often has control or influence over one of the two, meaning we can get a better handle on the complexity. Going back to the retail example, while we can’t possibly know everything that affects customer behavior, i.e. their intent, we can control the environment within which we interact with customers, such as a website.
So this means we need two approaches for better decision making, one for when the complexity is context-based and one when it is intent-based. But this isn’t the only way we can break down these complex relationships.
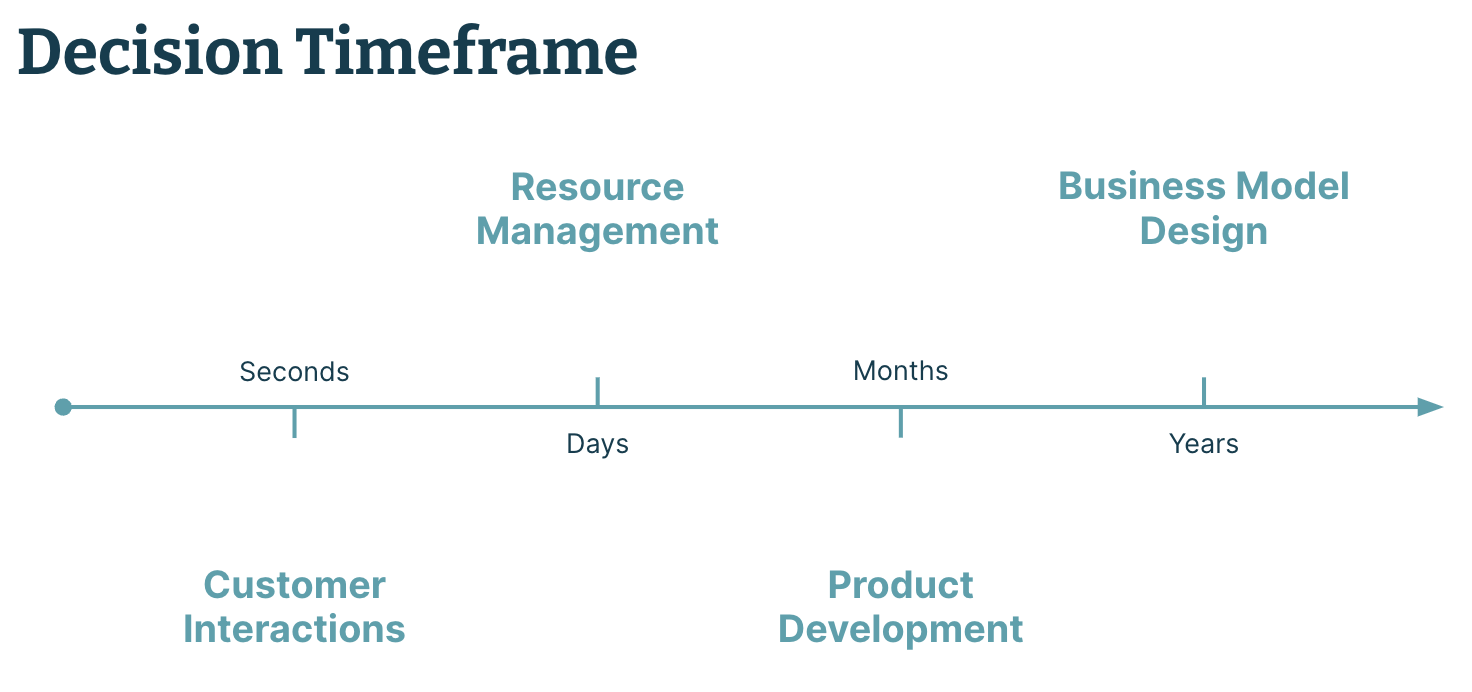
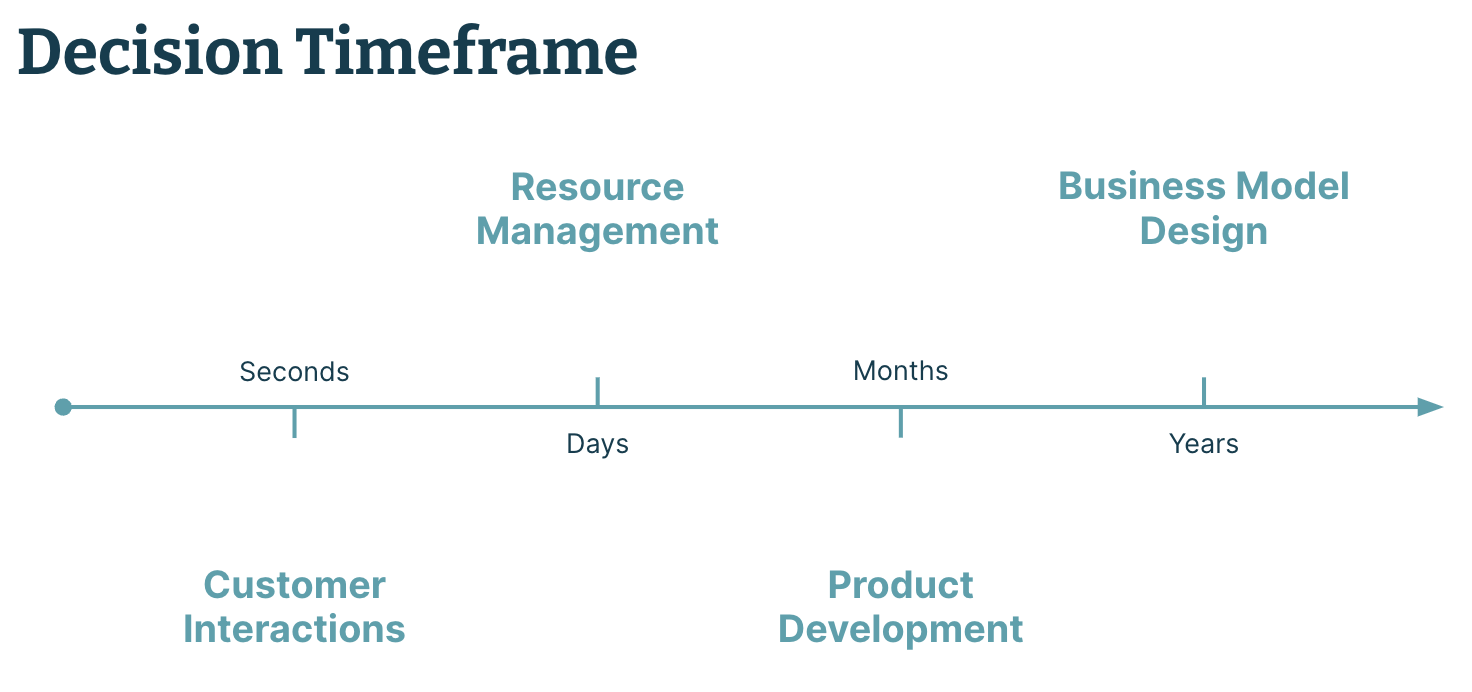
Different types of decisions have feedback loops, varying from fast customer interactions happening in a matter of seconds, to strategic business model decisions that might last years
Different decisions have different time frames over which they act which impact the feedback loops for us to gather enough data to learn from. This therefore constrains how we run our experiments as not all situations allow for the standard iterative approach. For example the outcomes of a strategic decision could take months or years to become apparent. Equally the cost of running multiple experiments could be incredibly high, such as in the case of product development. What this means is we again need a different approach depending on the timeframe over which the decision will play out. One for short-term, high-frequency decisions and another for long-term, low frequency decisions.
The analysis above gives us two dimensions across which cause and effect relationships vary greatly. Short, intent-based relationships are a very different problem to long context-based ones. To implement this in practice, we’d therefore need a technique that can handle all four types. We believe we have created such an approach.
Enter AI Augmented
Thoughtworks has created a family of four approaches called AI Augmented. Each approach is specifically designed for one of the four types of complex problem it aims to solve, while ultimately optimizing for human learning. What's more, the approaches span the full range of complex decision-making that takes place in an organization:
Automation Augmented interacts with its environment in real-time to personalize virtually any short-lived, high-frequency decision, by iteratively exploring and testing.
Operations Augmented optimizes day-to-day operational decisions in resource constrained situations, while remaining resilient to change.
Creativity Augmented creates an interactive R&D process between AI and experts to enable speed and quality in product development.
Strategy Augmented focuses on codifying & simulating scenarios, enabling robust and transparent strategies to be built and tested.
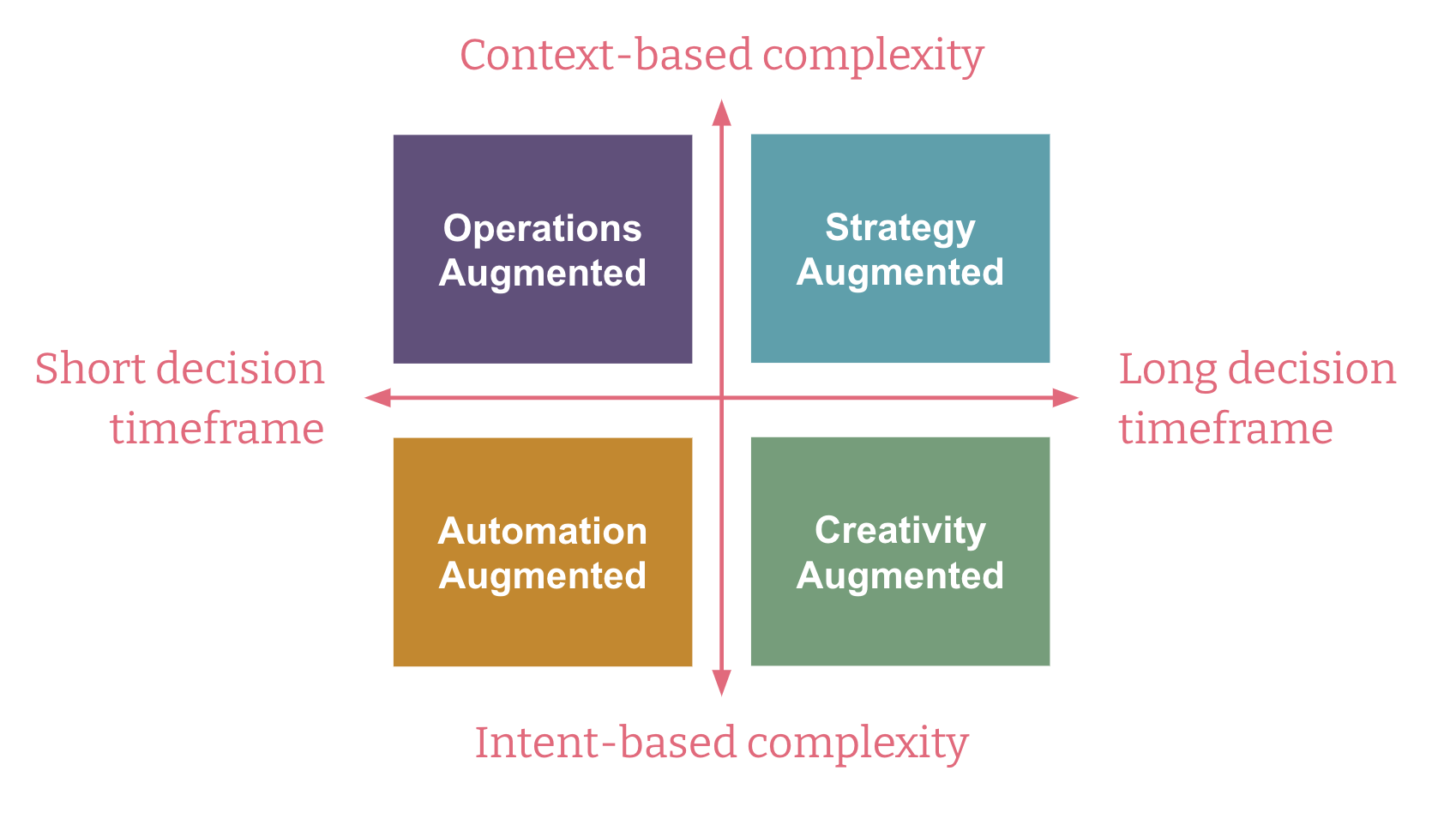
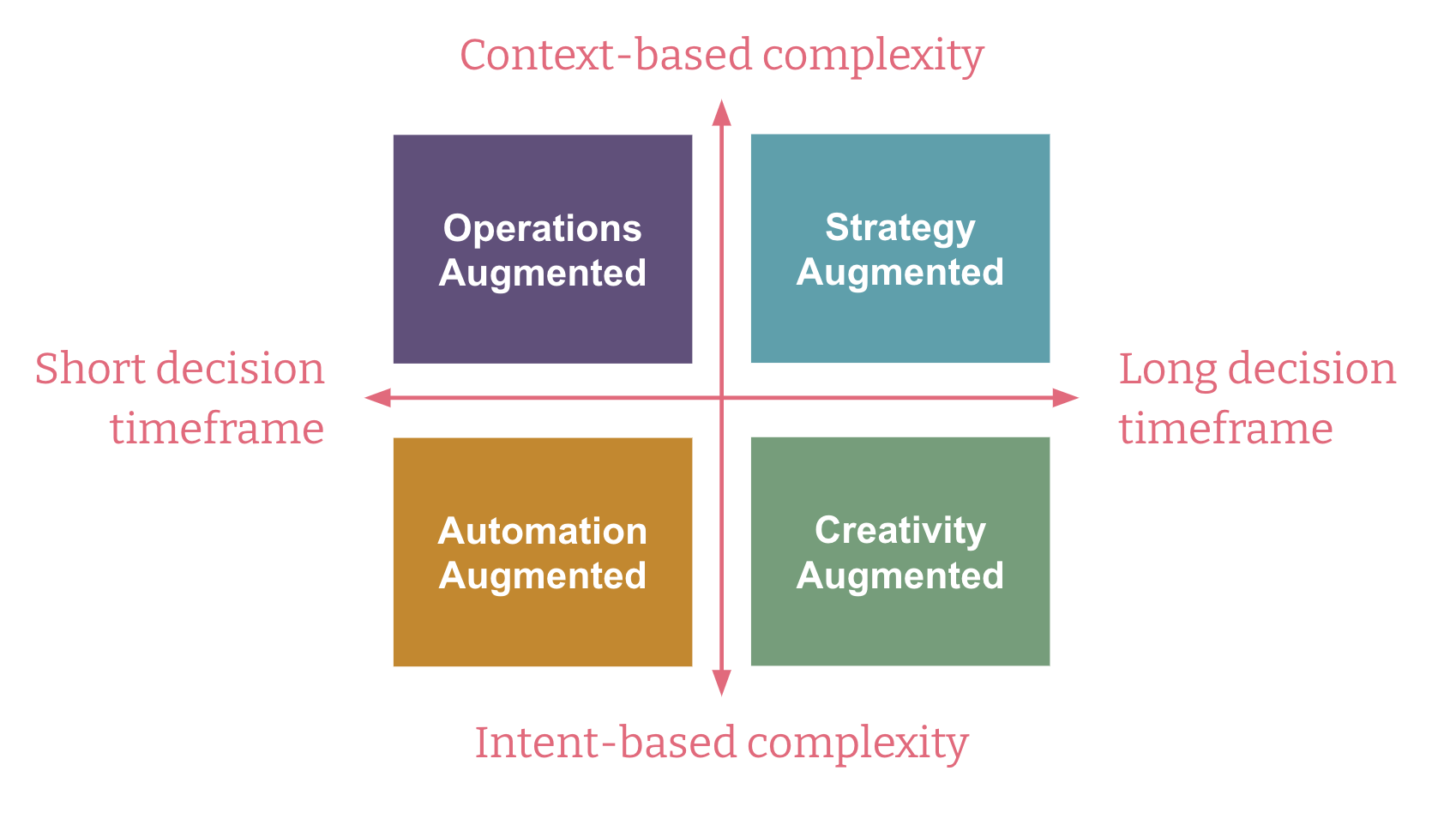
These approaches aren’t just theory though, we have already implemented them with our clients across a variety of industries, and the results speak for themselves. Our recent whitepaper on AI Augmented highlights some of the most exciting applications we’ve delivered to date and gives more insight into how each approach works.
While each individual application of the approaches can deliver tremendous value, it’s when multiple are deployed at scale across an organization that we see the sorts of game-changing results and cost savings discussed at the beginning of the article. Decision effectiveness at this scale requires an enterprise data approach to match - Data Mesh.
Making better decisions, faster and at scale
We believe the four foundational principles of Data Mesh create the perfect environment for AI Augmented.
Domain ownership ensures that the AI Augmented systems are as close as possible to the people and environments they are interacting with, enabling real-time learning through experimentation and the capture of domain expertise.
The concept of data as a product, and product thinking, ensures AI is designed for the people who use it - an absolute essential for facilitating human learning and effective decision making.
To reach a scale of 400 million decisions a day, self-service infrastructure is needed to remove the barriers to entry for setting-up AI Augmented systems, democratizing access to these capabilities.
Federated governance is the best way to ensure compliance at scale while transparency, explainability and ethical considerations all within the context of use.
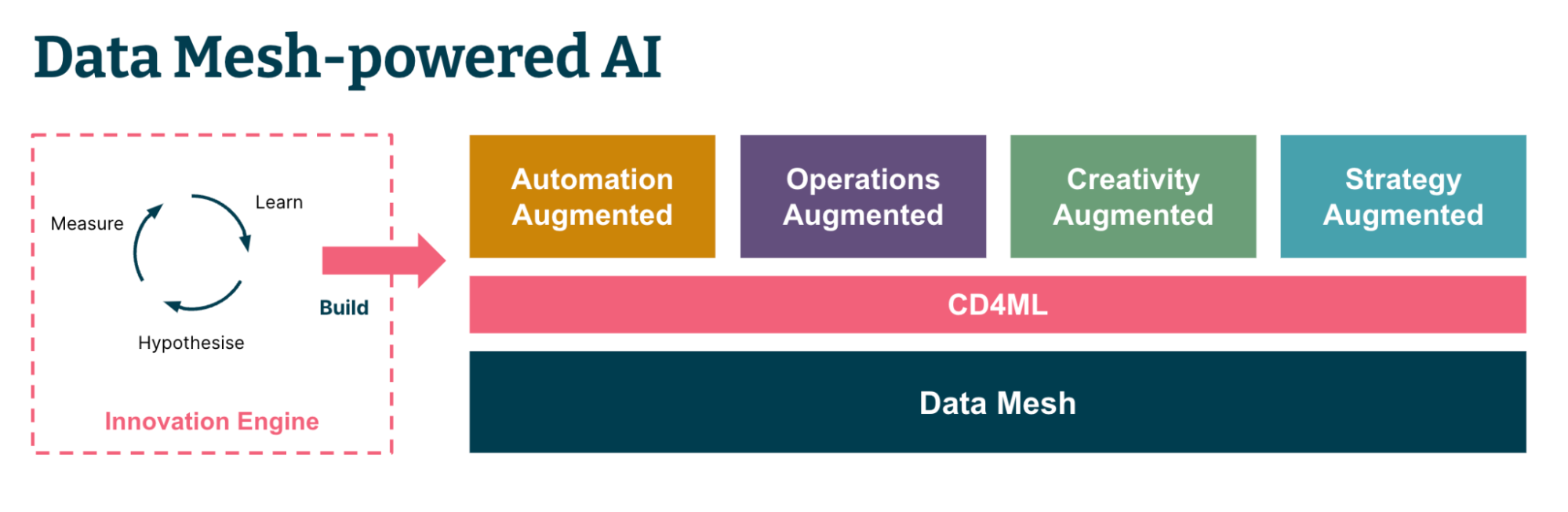
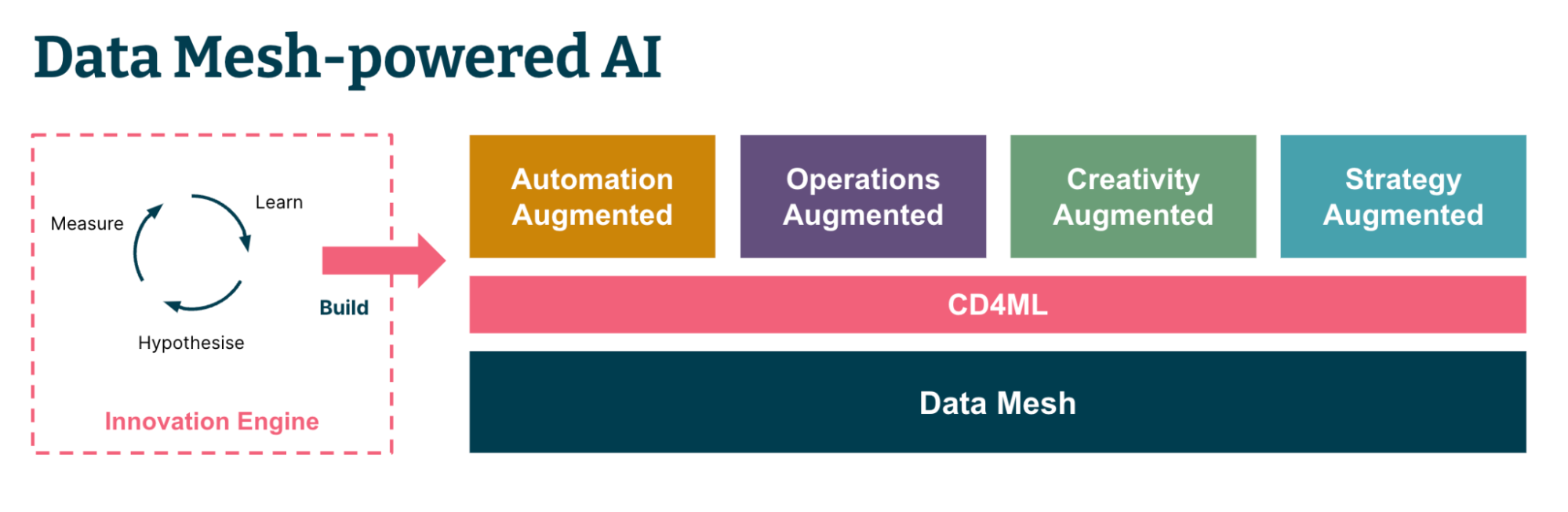
In the above diagram, we see each of the four approaches forming templates for data products that will sit on top of the mesh supported by a CD4ML / MLOps platform offered as part of the self-service infrastructure.
When an approach is employed within the data mesh, we expect it to start its life within an innovation function, where data scientists and engineers can work with subject matter experts to ensure the approach is properly suited to its needs, before it is handed over to a data product team to mature and manage. The data product will then work like any other product, driving value for the domain and business as a whole. It will create insights and data for other data products to consume - most likely other AI Augmented data products creating a ripple effect that amplifies the effectiveness of decision over time, generating value across multiple scales of the business.
We envision a situation where insights flow from Automation Augmented products, through Operations, Creativity and Strategy Augmented ones, with new insights being drawn at each stage, enriching the wealth of cause and effect relationships available to the decision makers. This is how organizations can solve their most difficult problem and reap the rewards of making better decisions, faster and at scale.