When it comes to data, many businesses still suffer a disconnect. Consciousness of the potential value of data has never been higher, yet enterprises still struggle to make the most of it. Companies have more tools and capabilities at their disposal than ever, but can’t necessarily translate information into commercial intelligence. Data is seen as a strategic resource, but many organizations have yet to define a data strategy.
One recent Harvard Business Review study found barely a quarter of companies feel they’re able to effectively measure and report on the business value of their data and analytics investments - despite 80% agreeing it’s important to do so. Research into the issues facing CEOs by PwC also points to a significant gap between the data business leaders know they need to make critical decisions, and the adequacy of the data they actually get.
The data disconnect
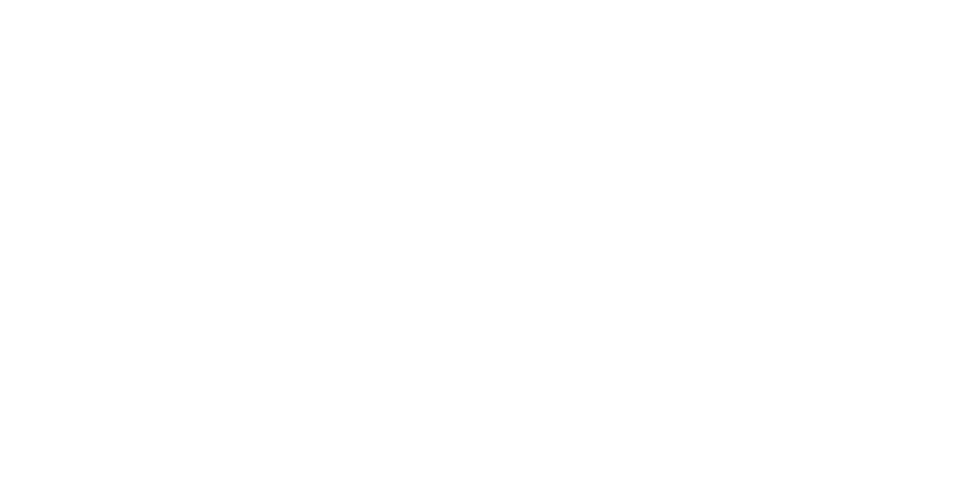
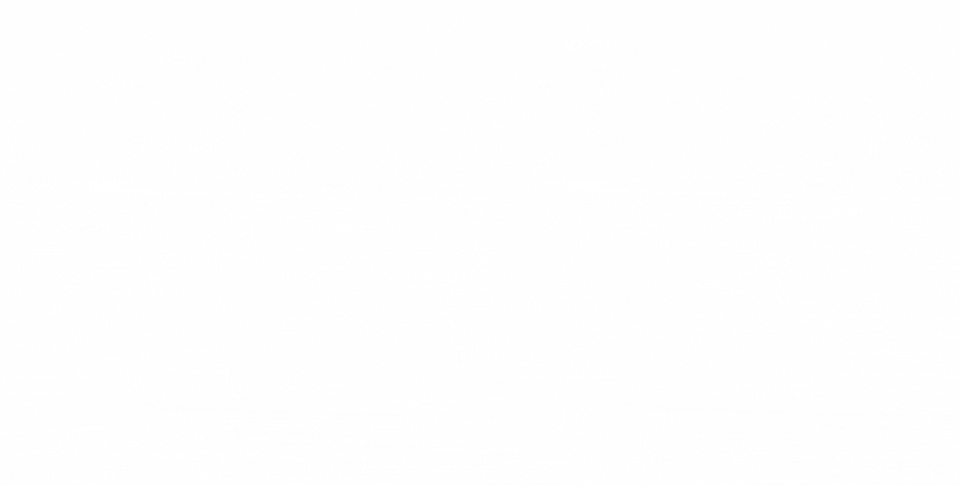
And with each year, it seems, the stakes get higher. “There’s a much stronger push towards digitization and self-service capabilities that has been driven by the pandemic,” says Emily Gorcenski, Principal Data Scientist and Head of Data, Germany at Thoughtworks. “Before, a lot of data analysis was done in hallways, around water coolers and in morning meetings, so it was a lot easier to get feedback on ideas and concepts.”
At the same time, Gorcenski adds, “conventional modes of data engineering and data architecture have largely failed to deliver on the promises that were made when the Big Data revolution happened. Part of the reason is that these centralized structures simply don’t scale with the number of use cases. We’re not bound by our imagination. We’re not limited by our ability to seek insight. We’re limited by our ability to find high quality data that we can trust.”
“Whether you’re looking at government or other services, the demand that people are putting on their digital channels, and the data infrastructure that supports that, has been rising exponentially,” agrees Prasanna Pendse, Head of Technology, India, at Thoughtworks. “It started even before the pandemic in the financial services industry, with more scrutiny of data governance, and the traceability of information by regulators. People are seeing data capabilities aren’t scaling to what they need, and realizing something needs to be fixed.”
A new crop of data demands is also being driven by one of the most fundamental technology shifts of our time - the rise of ‘coopetition,’ and business platforms being drawn (ready or not) into broader ecosystems.
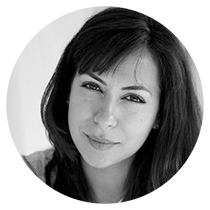
“As organizations are pulled into these ecosystems, sharing data becomes more important, and difficult, because they have to do it across trust boundaries. Even managing your own data is a challenge, and now you need solutions that go beyond the bounds of a particular organization.”
Zhamak Dehghani
Director of Emerging Technologies, Thoughtworks North America
“We’re seeing this hyper-convergence, with the rapid emergence of ecosystems in industries like healthcare - where COVID-19 has ushered in close collaborations between providers, payers and virtual healthcare technologies,” says Zhamak Dehghani, Director of Emerging Technologies, North America at Thoughtworks. “As organizations are pulled into these ecosystems, sharing data becomes more important, and difficult, because they have to do it across trust boundaries. Even managing your own data is a challenge, and now you need solutions that go beyond the bounds of a particular organization.”
These pressures make it crucial to be able to rapidly access and experiment with a critical mass of relevant and trustworthy data - a capability that most enterprises still lack.
“For half a century we’ve been stuck in the bootstrapping phase of becoming data-driven at scale - getting access to data at scale in the first place to build data-dependent solutions,” Dehghani notes. “I see it all the time at conferences - data scientists, who are the prime users of the data, talking about this model or that model, then ending their presentations by saying “but we don’t have access to the data at scale.”
Enterprises are learning, in many cases the hard way, that “data itself has no value besides what you can do with it, and what actions you can take from it,” Gorcenski says. “Those decisions require people. Before the pandemic it was easy to shift that role to a data analyst and let them come to you with those conclusions. But now there’s much more of a need to have those insights at your fingertips with a self-service capability.”
There is a clear path to capturing data’s full potential. But it requires a degree of technological and organizational change - and also, Gorcenski notes, a willingness to go where the data takes you.
“Data should challenge our assumptions and instincts from time to time,” she says. “And if it doesn’t, then there’s something wrong. Why are we even bothering to collect all this data if we’re just going to go with our gut anyway? We need to give data a bit of control over our destinies, which can be scary - and if you don’t trust your data you’ll never do that. In order to trust your data you need a clear chain of responsibility showing who’s generating it, who’s processing it, what it means, where it’s coming from, what it means historically and in the current context. All that is necessary to get to a point where you allow data to make recommendations, and drive decision-making.”
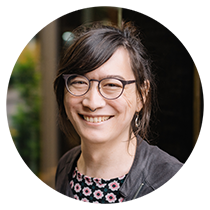
"Data should challenge our assumptions and instincts from time to time. And if it doesn’t, then there’s something wrong."
Emily Gorcenski
Principal Data Scientist and Head of Data, Thoughtworks Germany
i. Data lakes everywhere (and not a drop to drink)
The failure to achieve this state of trust is rooted in data’s historical trajectory.
“We have to challenge this very fundamental assumption that for any company or business unit to engage in data-driven experimentation they must have access to centralized data to get any meaning out of it,” Dehghani says. “That paradigm has become a blocker to scale in any meaningful way. which impacts how we build organizations and teams, and leads to how technology has been built bottom-up.”
The bias towards centralization, in the form of data warehouses and later on data lakes, means teams that are not intimately familiar with the data, its origin or its usage are centrally created and made responsible for it. This leads to blockages and some of the truthfulness of the data being ‘lost in translation.’
A typical data lake/organizational structure
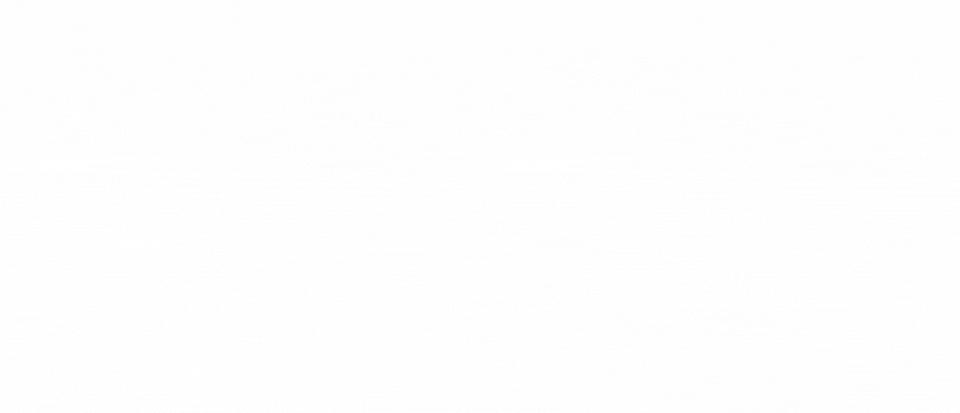
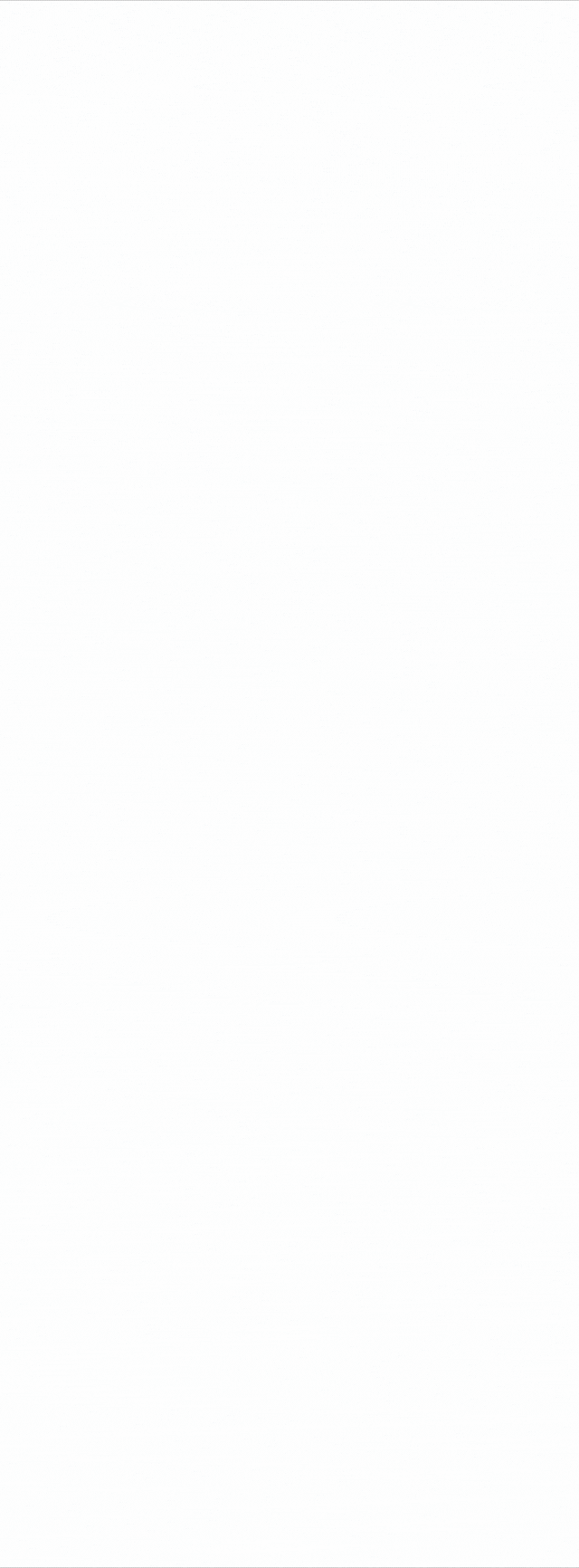
Centralization in effect divorces data from the operational units that often generate it and need it the most. “The organizational structure leaves the data team sitting in a corner,” says Pendse. “Yes, everything might flow through it, but from an organizational perspective the teams are not particularly well-aligned with growth priorities. Part of that is also the way these teams are defined, as business intelligence groups or something similar. This mindset is that of descriptive analytics, which is ‘tell me what’s been happening.’ It’s difficult to shift to the predictive, and eventually prescriptive, way of doing things.”
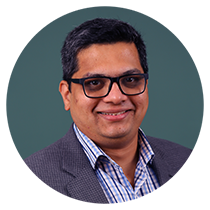
"The organizational structure leaves the data team sitting in a corner. Yes, everything might flow through it, but from an organizational perspective the teams are not particularly well-aligned with growth priorities."
Prasanna Pendse
Head of Technology, Thoughtworks India
This operating model is also inherently inflexible. “If you have one massive, central data monolith, any change in how you work with it becomes a massive project in its own right,” Gorcenski says. “Data is all about reacting to what’s happening in the world. Your data’s going to change, and you want your data to change, you want new customers and new markets, so you need to build a structure that is reactive to change and adaptable. If you have new controls, it should be a minimal amount of work to implement them.”
To move from ‘having’ data to using it as a basis for products, personalization and better customer experience - freedom to experiment is essential. Monolithic data architecture can make this a monumental task, extending the gap between theory and action. “There’s a tooling aspect to it that dictates the cycle time it takes to make decisions,” Pendse explains. “In a lot of traditional companies a single experiment will take six months to run and is probably running only within a certain limited area, not in parallel with other experiments.”
ii. Shifting towards a mesh
The business case is clear: instead of creating lakes, or silos, organizations should pursue a nimbler approach to data, bringing it closer to parts of the business where it’s directly relevant.
This can be achieved by applying two core principles - domain-oriented data and data as a product. Domain-oriented ownership and distribution breaks data architecture down around individual functions while maintaining overarching connectedness and integrity. Utilizing data as a product, and not just a resource, becomes something that’s a pleasure to consume and use. These practices are the basis of a data architecture designed for a resilient, and fast-acting, digital business: Data Mesh.
Data mesh
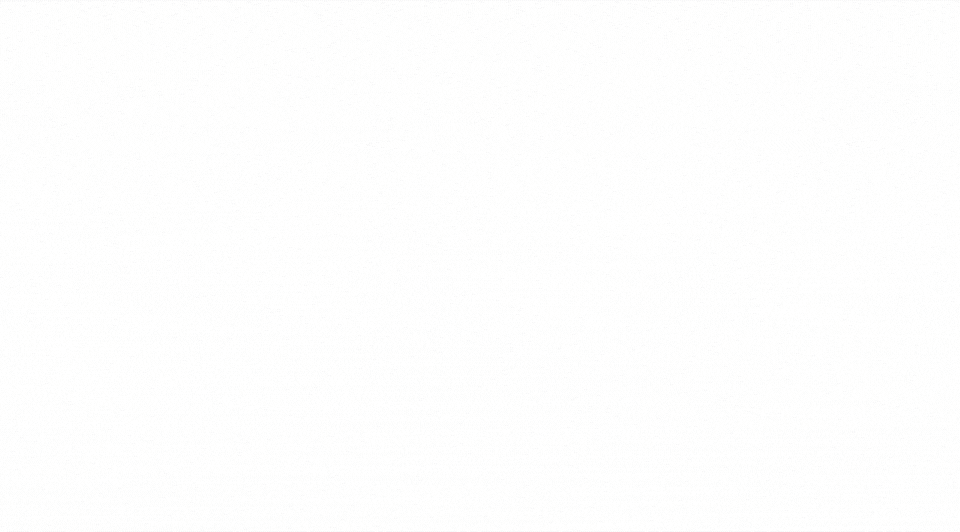
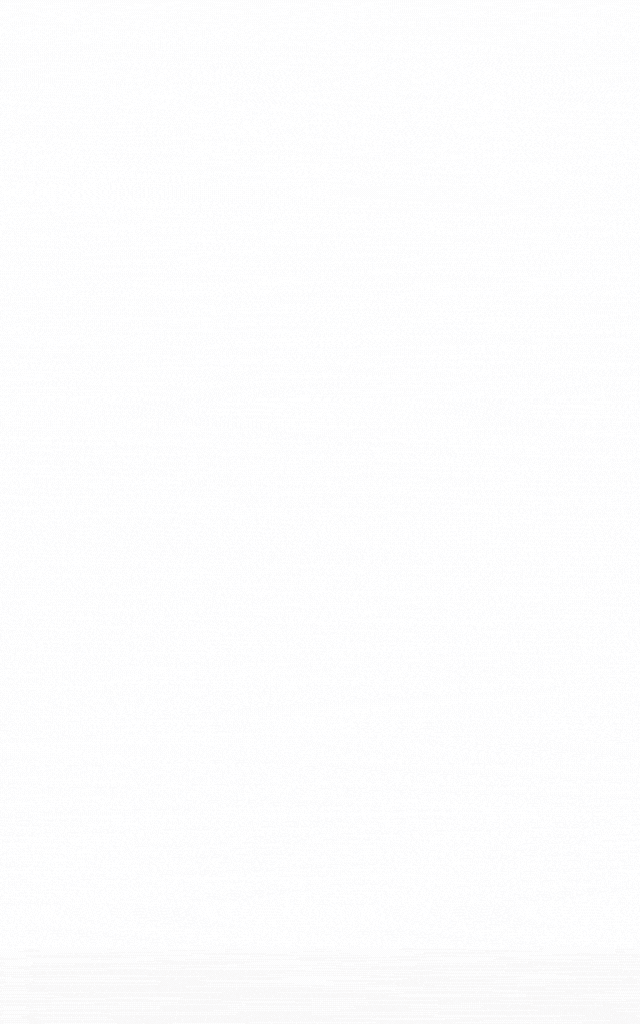
“Data Mesh looks at the root cause of the inability to use data at scale, and tries to address it,” Dehghani explains. “For many years we’ve decided to decompose this big problem of data into monolithic solutions and teams, within certain technical boundaries, but haven’t been able to grow faster or scale out experimentation quicker. Data Mesh learns from the operational world, where digital companies have decomposed their business around domains, and continues that journey with data, giving the control and sovereignty to the people who are best positioned to generate and share it. It’s a natural progression.”
Data Mesh doesn’t necessarily mean centralized data repositories will disappear, Gorcenski notes. “Data lakes and data warehouses will probably never truly go away,” she says. “What is going to happen, and what the Data Mesh concept is all about, is separating these concerns into domains. It’s up to the domain to decouple the product and the infrastructure in a way that eliminates bottlenecks, but allows you to create the data products that make sense. It’s not about creating one grand model for how you access data, it’s about the principle of making it easy to get access to data wherever it resides, and then building out your infrastructure to support that.”
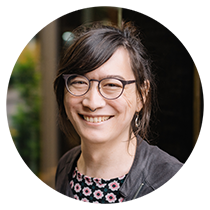
"It’s not about creating one grand model for how you access data, it’s about the principle of making it easy to get access to data wherever it resides, and then building out your infrastructure to support that.”
Emily Gorcenski
Principal Data Scientist and Head of Data, Thoughtworks Germany
A distributed approach has a number of built-in advantages. One is that it mitigates the risk of keeping all your data in one basket, which can rapidly become a single point of failure whenever it’s inundated with requests or subject to an attack.
“If you look at the defensive side of things where your servers are brought down because of too much demand, actually your own success becomes bad news,” Pendse points out. “Not only do you lose money that’s not coming in because the door is shut, but it also opens up security vulnerabilities, which creates other risks.”
Distribution also naturally makes data more accessible by channelling it directly to teams, “giving ownership to people that understand the data and are best positioned to control it,” Dehghani explains. “Of course, you also have to have these people talk to each other under some kind of federated construct, because you won’t only get value out of a single data domain. Higher order intelligence is created by joining and correlating data from different domains.”
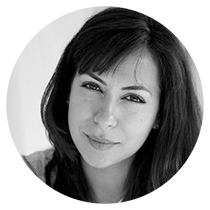
“Data as a product is very different from data as an asset. What do you do with an asset? You collect and hoard it. With a product it’s the other way around. You share it and make the experience of that data more delightful.”
Zhamak Dehghani
Director of Emerging Technologies, Thoughtworks North America
Applying product thinking to data is key to making sure domain teams remain connected, and incentivized to share. “Data as a product is very different from data as an asset,” says Dehghani. “What do you do with an asset? You collect and hoard it. With a product it’s the other way around. You share it and make the experience of that data more delightful, and you want more customers.”
“In the typical model anyone who’s building a technology product is generating data as a by-product,” adds Gorcenski. “We want to switch that around and really think of data as a product at every stage of the way. When we’re driving deeper insights and better data for our products, we can’t simply view data as an accumulation of several little transactional bits. Data is no longer your system working when it takes in inputs and emits outputs, but when it does that and generates data sets that reflect the reality you’re seeing - and that you can use to generate feedback cycles within the organization, to answer questions like: Are we selling the right things? Are we reaching the right consumers? Are we making the right product with the right levels of efficiency?”
Dehghani notes this requires the development of “self-serve” data infrastructure as a platform, which transfers autonomy to domain teams and enables different ‘pools’ of data to be accessed and shared as required, in a secure, compliant and distributed way.
iii. Laying the technology foundation
A self-serve platform positions data to be put to immediate use, rather than passively stored and accessed before undergoing other processes, as in a data lake. “Modern platform architectures are very good at getting rid of a lot of the process and noise that happens with data engineering, allowing us to get much closer to the data and to get those insights out much faster,” Gorcenski explains. Such architectures have proven a powerful enabler for enterprises that position teams to access standardized data capabilities that can be assembled to create different products.
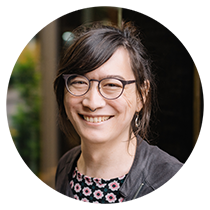
“Modern platform architectures are very good at getting rid of a lot of the process and noise that happens with data engineering, allowing us to get much closer to the data and to get those insights out much faster.”
Emily Gorcenski
Principal Data Scientist and Head of Data, Thoughtworks Germany
When developing a data platform, enterprises won’t always be starting from scratch. Dehghani notes that existing cloud technologies can act as a “utility layer,” providing the storage and streaming capabilities and standards upon which more mature layers of the platform are built to support interactions with distributed architecture and decentralized teams.
At most organizations, “the utility later is there, but it’s been built to assume data is going to be centralized, and there’s a layer of technology that’s absent around the orchestration of the distribution of data,” she explains. “If you decide to put ownership of the data in the hands of different domains, not a central group of hyper-specialized data engineers, you need to raise the abstraction of the platform to a level that a generalist developer can also get the analytical data they need to build a microservice or application. That shift of power, from the specialist to the generalist being able to generate meaningful, useful data, requires engineering commitment.”
Data platform model
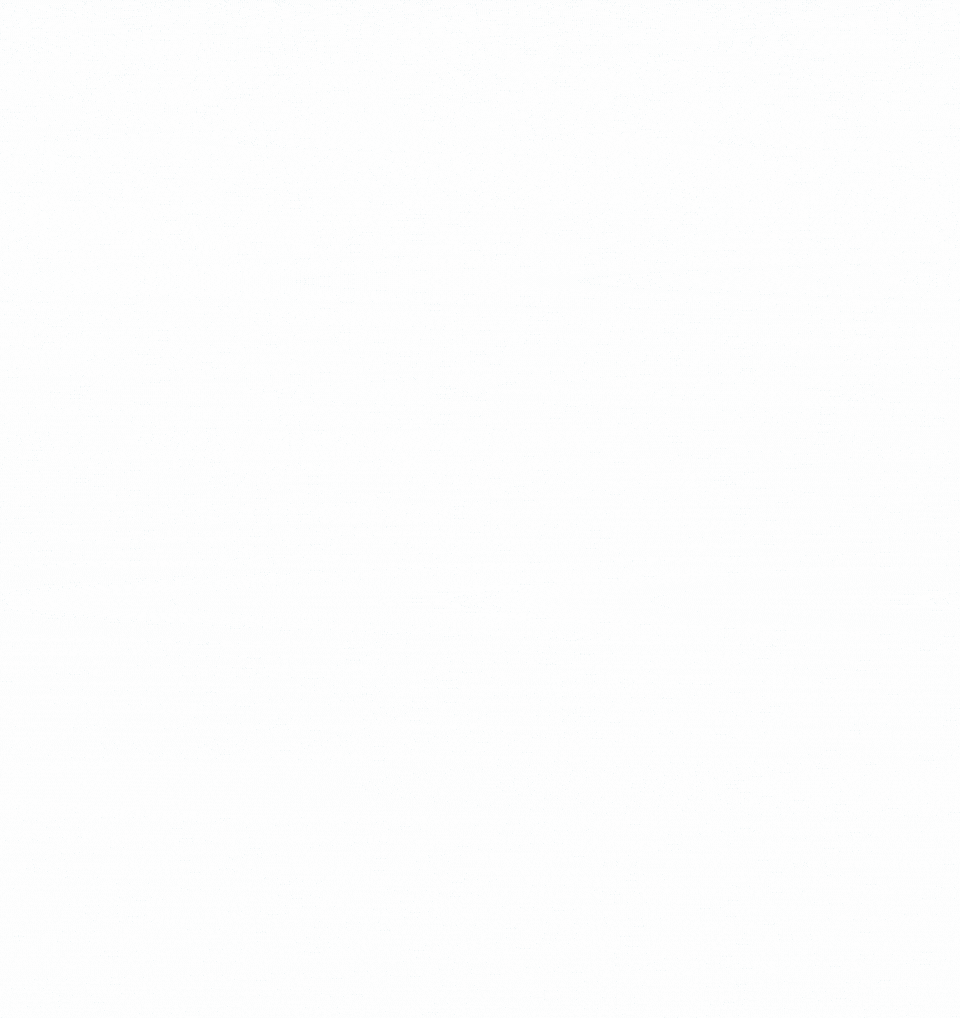
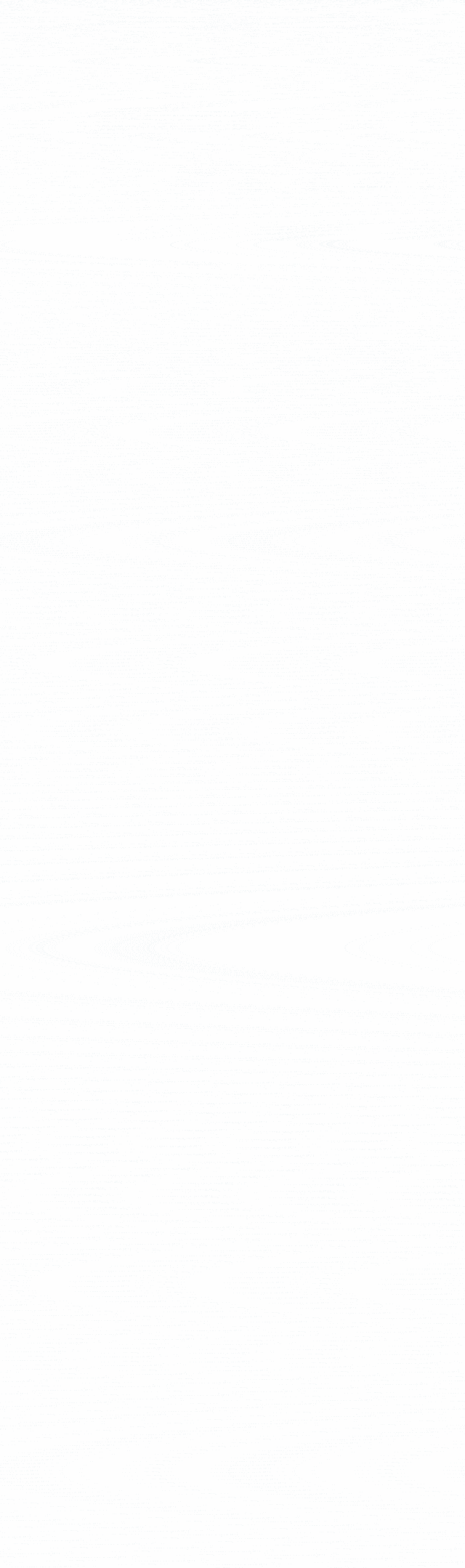
In a mesh “the technology isn’t vastly different, but how you manage and see data will certainly change,” says Pendse.
Many organizations are still wedded to perceptions that storage is an expensive and limited resource; that duplication must be avoided at all costs; and that creating a new data repository is likely to be a two or three-year effort. But advances in infrastructure and practice mean in making data infrastructure decisions, these should no longer be the enterprise’s primary concerns.
“The mindset has to shift to ask: What is the fit for purpose mechanism to achieve my objective, and how do I create it in a decoupled way so that I can optimize our speed? With the infrastructure acceleration, tooling and automation that’s now available, you’re able to spin out a new data domain, make it self-serve, even add access controls and things like that quite quickly,” Pendse says. “The first time around it might take you a few months, but after that it could be just a few minutes.”
iv. Aligning people and strategy
Data architecture can be complex, but the biggest bottlenecks on the road to becoming more data-driven have less to do with technology or engineering than culture and people.
“The truth is, people look at data and particularly data governance and their general reaction is to groan,” Pendse says. “It’s seen as boring, something they have to manage, a burden. Leaders may believe in it, but they can’t get buy-in from their teams. These perceptions have to change, so people are interested, want to use and consume the data, and are excited about the possibilities.”
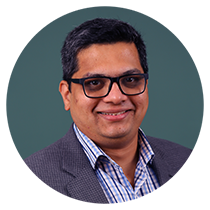
“The truth is, people look at data and particularly data governance and their general reaction is to groan. These perceptions have to change, so people are interested, want to use and consume the data, and are excited about the possibilities.”
Prasanna Pendse
Head of Technology, Thoughtworks India
To make that case, “a clear and comprehensive data strategy is the first thing you need to have in place,” says Gorcenski. “That needs to come through a culture of evangelization of what data means and why it’s valuable to the organization, whether it’s for regulatory reasons, process control, or aspirational, to create more insights or build more products. It might come from the C-level but has to be embraced by all the key players in the organization, right down to the people writing the code.”
Defining the strategic purpose of data also makes it easy to decide what data and related solutions to prioritize. “We always recommend working backwards - start with your bets, your strategic goals as a company, turn those into actual use cases and projects, and then identify the data products and datasets you need to unlock those use cases - where they come from and which teams own them,” Dehghani explains.
To encourage those teams to work with data in the right way, incentive structures may also need to change to reflect the focus on data as a product, measuring the value it is generating, or how often it’s consumed by end users, instead of how much data is processed or generated. Pivots like these, and the loss of control, can cause discomfort among those who were the guardians or ‘owners’ of data historically - but Dehghani believes they can be quickly won over.
“The people who have traditionally been responsible for data platforms have often been in a world of pain themselves,” she explains. “They’ve been stuck in a model where they’re struggling to make customers happy, or give people access to data, consuming data from upstream sources who may not be motivated to make it meaningful or trustworthy. You can give them the tools to show they don’t have to pave the path by themselves, and that they’ll be rewarded by the number of people using data products. There are intrinsic incentives when these people realize the power data has to optimize a business, product or application, to really embed intelligence throughout the organization. That’s when they become part of the solution.”
“You need to actually demonstrate benefit to people as you do this – it can’t just be a forced approach,” agrees Pendse. “For example, at one bank where we put in an access control system, there were worries everyone would be angry about it because they no longer had as much access to data, or needed to get a request to get it. But that ended up not being the case because the new system gave consistent data, it was more responsive and didn’t go down like the old one did. People gravitated towards the system because it worked.”
Gorcenski recommends testing new data models around individual departments or domains, who can fine-tune the approach and eventually act as ‘ambassadors’ to the rest of the enterprise.
“You need to start fairly small and pick the learnings, and have really close feedback cycles to figure out what works and what needs to be adjusted,” she explains. “Give those teams free rein to build things and to bypass the change management policies that are in place. Then you need to look at the goals they’re accomplishing, whether they’re achieving them according to your strategy, and realizing the benefits. Find the right people to be the champions, and empower them by giving them the time and space to go make that change, and then promote within the organization.”
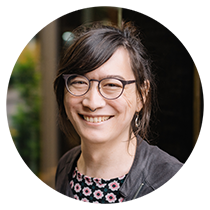
“You need to start fairly small and pick the learnings, and have really close feedback cycles to figure out what works and what needs to be adjusted. Find the right people to be the champions, and empower them by giving them the time and space to go make that change, and then promote within the organization.”
Emily Gorcenski
Principal Data Scientist and Head of Data, Thoughtworks Germany
v. Embedding security and governance
Allowing all this freedom may seem problematic, given business leaders remain highly, and correctly, concerned about any potential weaknesses in data security and governance. Decentralization can be seen as risky, because it removes a single gateway or point of control.
Top data security concerns of CIOs/IT leaders
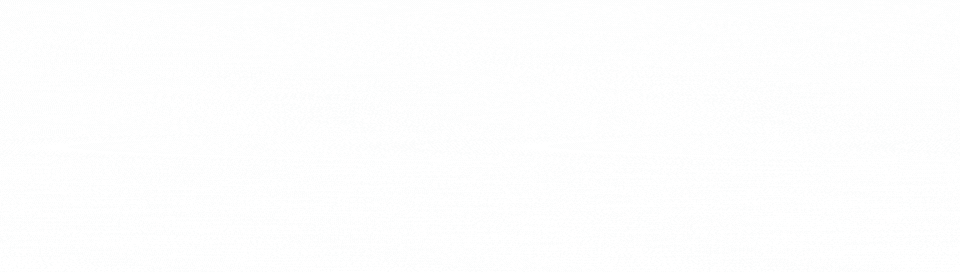
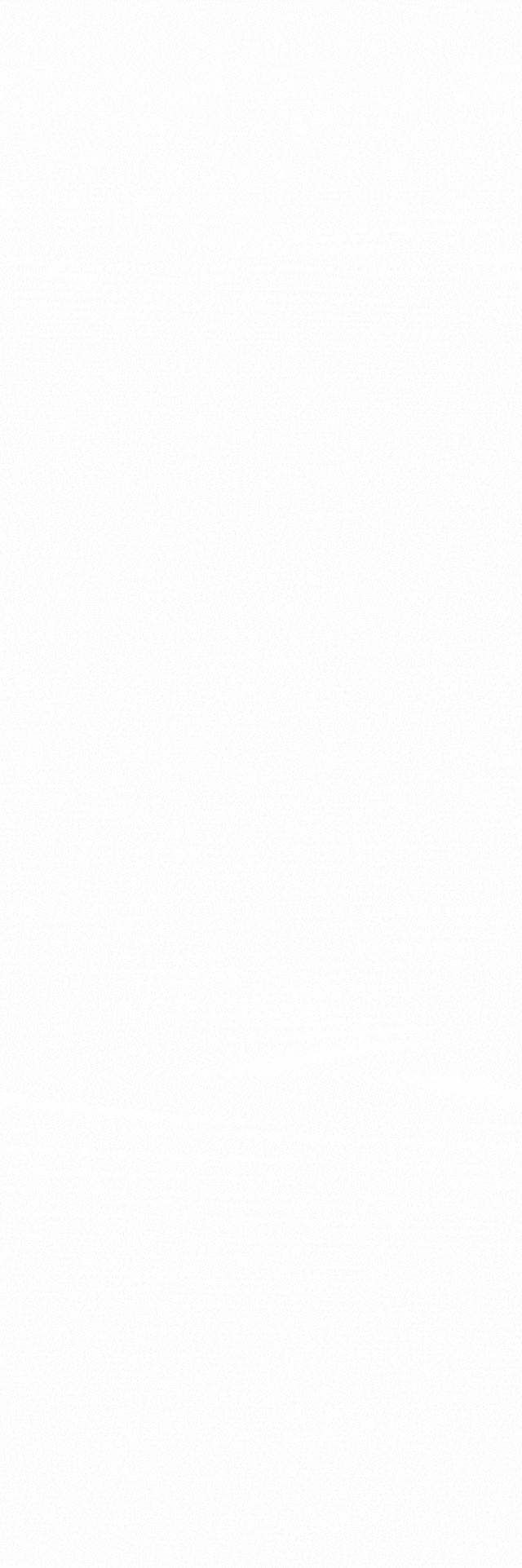
But according to Thoughtworks experts, distributing data closer to teams actually has positive governance impacts. “In the traditional approach data governance tooling, because of its centralized nature, has issues with data performance and length of processes,” notes Pendse. “With a mesh, those go away and people feel more productive. It enhances quality on the producer’s side by giving more granular control to the people who are actually creating the data, who know it the best, so the accuracy of how they tag that data goes up from the compliance perspective. It also improves quality for consumers by giving them control over how they want to consume the data, without having to doubt whether it is what it claims to be or not.”
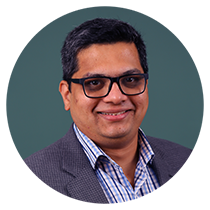
"A mesh enhances quality on the producer’s side by giving more granular control to the people who are actually creating the data, who know it the best, so the accuracy of how they tag that data goes up from the compliance perspective."
Prasanna Pendse
Head of Technology, Thoughtworks India
Dehghani sees clear parallels with the security and governance approaches adopted in moving computation from data centers to the cloud, where there is a transition from perimeters and ‘walled gardens’ to zero-trust architecture in which everything is essentially open, but every endpoint has built-in security, and the identity of every actor is constantly verified.
“The same thing applies here,” she says. “In the past there’s been a single, centralized body accountable for data being secure, available and modelled, and it becomes this bureaucratic, rather dysfunctional unit that gets in the way of innovation and isn’t really able to secure the data either. The inverted model of that is that the governance function becomes a federation, because once you decentralize the ownership, those owners have accountability in both executing data management policies as well as contributing to what those policies are. At the same time, it’s important that you also have elements of platform and automation that are very, very powerful.”
“Governance should be an enabling, not a restrictive force,” notes Gorcenski. “A lot of companies view privacy, compliance and security as cost centers, not value-drivers, and we’re so focused on making sure data is compliant and secure that we’re not thinking about the impact that’s having and what we’re preventing people from doing. We need to start from the idea that yes, compliance is a challenge, but there are good tools out there, and we can architect our systems to be compliant and to build trust, which will then give our teams free rein to build better products. You need to train not just data people but everyone who works with data, to be able to spot these issues and have a forum in which they can raise concerns and get answers to things. Building a culture of data privacy within the organization is crucial.”
“If your risk management strategy is just to never take on risk, sure, you might get away with it,” she adds. “But you’re not going to innovate, and you’re not going to recognize the value of your data.”
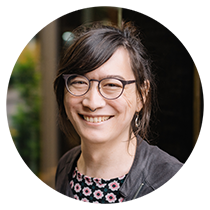
“If your risk management strategy is just to never take on risk, sure, you might get away with it. But you’re not going to innovate, and you’re not going to recognize the value of your data.”
Emily Gorcenski
Principal Data Scientist and Head of Data, Thoughtworks Germany
vi. Dealing with the data talent shortage
In implementing security and other policies around data, businesses often fret about a shortage of expertise – and indeed studies show demand for data skills continues to outstrip supply.
However as Gorcenski points out, companies are often “sitting on data talent that they don’t realize they have” - people who may have a strong interest in data but that have been prevented from interacting with systems or working with developers because these tasks don’t fall under their formal role.
Biggest technology skills shortages for businesses
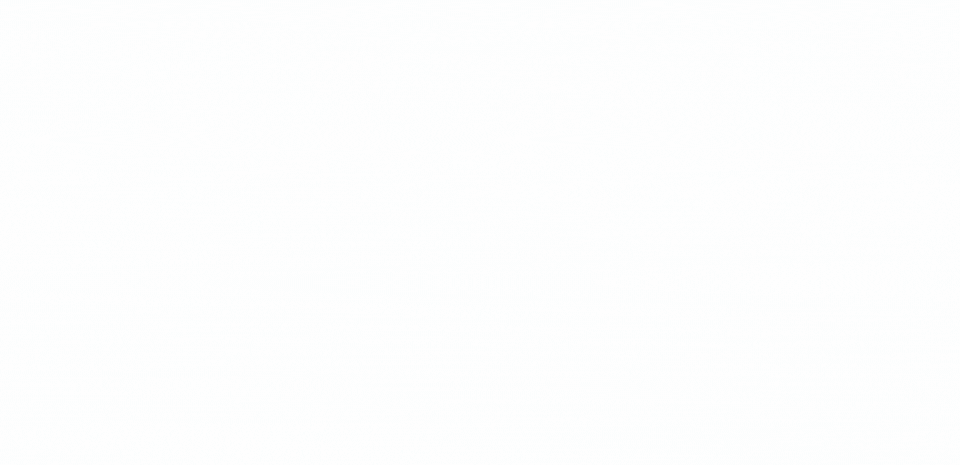
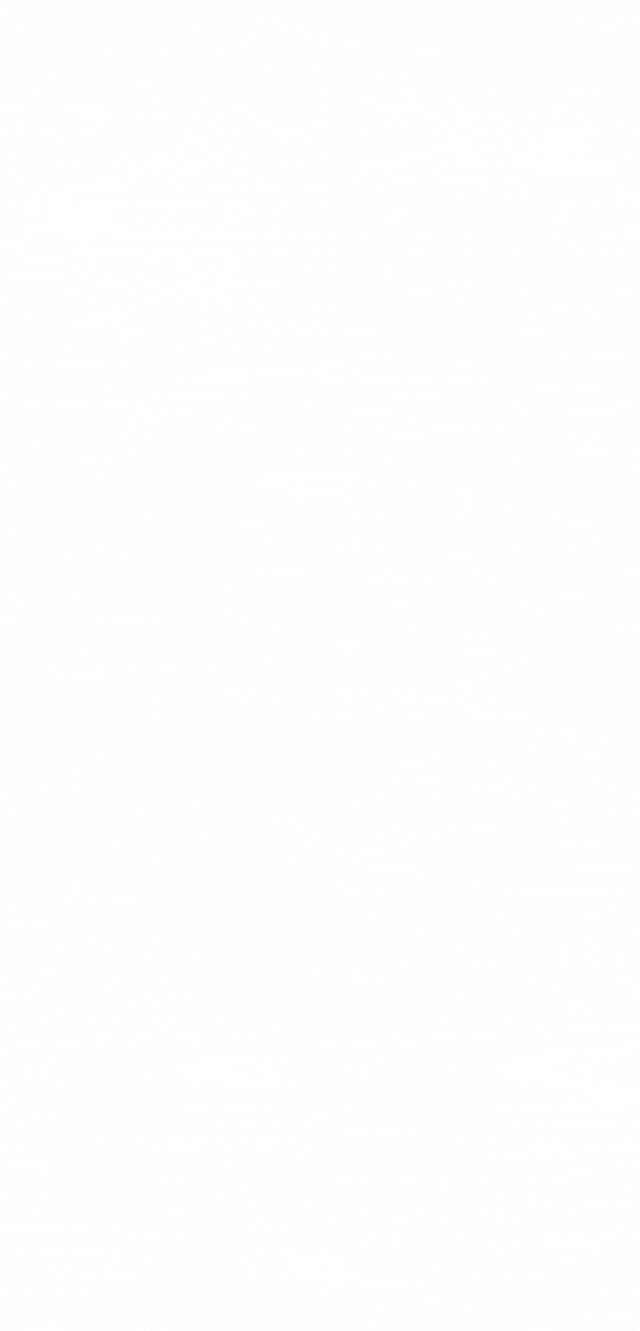
“The Data Mesh concept is about federating responsibilities more into the domain teams, letting people play in these sandbox environments, giving them access,” she says. “You’ll be surprised what they come up with. We just need to get people more hands-on experience with data systems, de-mystify them, make them less scary and less tightly controlled. It’s easy to spin up new environments now - let’s just do it and get people playing and poking around. It’s okay to break test environments. That’s what they’re for.”
Pendse notes efforts to train or reskill existing talent can often produce more return on investment than racing to recruit new data specialists. “Data engineering is a different mindset than application development, but it’s not impenetrable,” he explains. “You just need some mentors to show you the ropes, go through some training, make some mistakes and eventually you’ll get good at it. Training some of the people who come in as application developers into the data engineering space has worked for us.”
Similarly, data science “is not rocket science,” he adds. “We used to look for people who had PhDs, but the building blocks of what you need to do at a skill set level actually come from college-level math, so we’re looking at how to leverage fresh graduates to go a little bit further.”
Ultimately, Dehghani is confident that the development of data platforms will disguise complexity to the point that the need for specialized data skills will be reduced, while advances in data science will cut the amount of modelling that companies need to do from scratch.
“There will be many reusable models that just need to be customized and tailored to understand the data for your business. And if you have platform capabilities that allow you to quickly train these models with different datasets and observe their behavior, it becomes a general engineering practice, solved like any other engineering problem,” she says. “This will enable advances in mobilizing a larger population of engineers and practitioners, rather than trying to create more specialized data scientists. Without insulting the specialists, I hope that even the data engineer label disappears as more people develop data capabilities, with the abstraction of accidental complexity enabling the up- and cross-skilling of a broader section of the workforce. That’s the data platform, data rich paradigm.”
vii. Brace for brave new approaches
The emerging platform paradigm is far from the only reason for optimism about how businesses will meet the data challenge in the future.
“There will be of course a bit of a battle between people that want to move towards more democratized availability of technology and data, and the people that hold the power right now,” Dehghani says. “But I’m already seeing the technical movements, talking to different hardware providers about the next model of computing to suit large sets of data that are dispersed. I'm very hopeful we will have a next generation of technologies that really turns the data problem on its head and solves it very differently than we have in the past. The response of the industry has been overwhelmingly positive in terms of Data Mesh and how enterprises can apply it.”
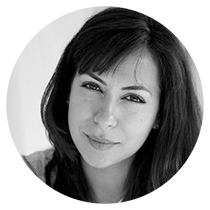
"I'm very hopeful we will have a next generation of technologies that really turns the data problem on its head and solves it very differently than we have in the past. The response of the industry has been overwhelmingly positive in terms of Data Mesh and how enterprises can apply it.”
Zhamak Dehghani
Director of Emerging Technologies, Thoughtworks North America
According to Pendse, while the focus is often on software and services many of the more exciting recent developments have been on the hardware side. “The whole fabric of computing is changing, with fit for purpose chip design,” he says. “Then there are developments like non-wallet IO memory, which basically means if you shut off your computer, your RAM doesn’t go away – persistent memory in other words. What happens to the idea of a database if an application is persistent even when the server shuts down?”
Gorcenski, meanwhile, sees massive potential in the vast amounts of data left untapped in the Internet of Things (IoT) space – and in enterprises striving to do genuinely new things with data, rather than emulating the approaches of luminaries like Google or Facebook.
“We need to look at how to use data to disrupt our own industries, not to do what Google is doing, but to do what nobody’s done before,” she says. “We need to stop thinking of other businesses as living in different worlds and start to see them as potential partners, finding ways to augment each other with data. Collaboration creates a better business ecosystem than competition in many cases. Recognizing those benefits requires bold thinkers who are willing to do challenging and complicated things and make that investment. It’s not going to happen in a quarter or a year, but it certainly is possible. There are more unsolved data problems than there are solved ones.”
Perspectives delivered to your inbox
Timely business and industry insights for digital leaders.
The Perspectives subscription brings you our experts’ best podcasts, articles, videos and events to expand upon our popular Perspectives publication.