Have you ever experienced the blank canvas syndrome trying to create a new design for a t-shirt or just graphics for your presentation slides? We all have.
In the world of Artificial Intelligence (AI), generating images of people’s faces, for example, is a problem already solved and is nothing new at this time, really. However, an AI model only generating random images of people’s faces won’t solve your blank canvas syndrome if faces are not what you are looking for.
More importantly, we want to augment our design processes with the help of AI, not replace them with it.
Thanks to the recent breakthroughs in multimodal AI, we can actually put humans into control and get rid of the blank canvas syndrome by telling the AI what we would like to generate - from faces to abstract illustrations and anything in between.
Computational creativity with multimodal AI
Multimodal AI refers to models understanding multiple modalities, such as text and images. Thus, the input to the model could be text and the output an image. Or the input could be text and image together and the output just an image as an example.
On the text modality side, natural language processing (NLP) methods have developed exponentially thanks to the “transformer” model architecture. You can even fill your blank text canvas by generating believable text with models like GPT-3. On the image modality side, transformer models have also emerged creating promising results in computer vision tasks, such as image segmentation and generation.
So, if the latest transformer models can process text and images, could you train a multimodal model generating images portraying the meaning of the text input? That’s exactly what OpenAI did in 2021 by training a multimodal model called DALL-E, which is capable of generating all kinds of images from text input prompts. At the time of writing this blog post, OpenAI also announced a much improved successor model called DALL-E 2.
DALL-E is basically a GPT (Generative Pre-trained Transformer) model for text and images. The way it works is that during the training of DALL-E, both the text and the image are encoded into sequences of tokens (to simplify, tokens could be words of text and pixels of images, for example). Next, token sequences are fed concatenated to the model and the model learns to predict the next token in the sequence. After the model training, you can just give a text input to the model which then starts to predict the wanted image token by token.
Thanks to this multimodal training procedure, the DALL-E model can generate plausible images of different subjects expressed in the text, and even combine unrelated concepts in a credible way.
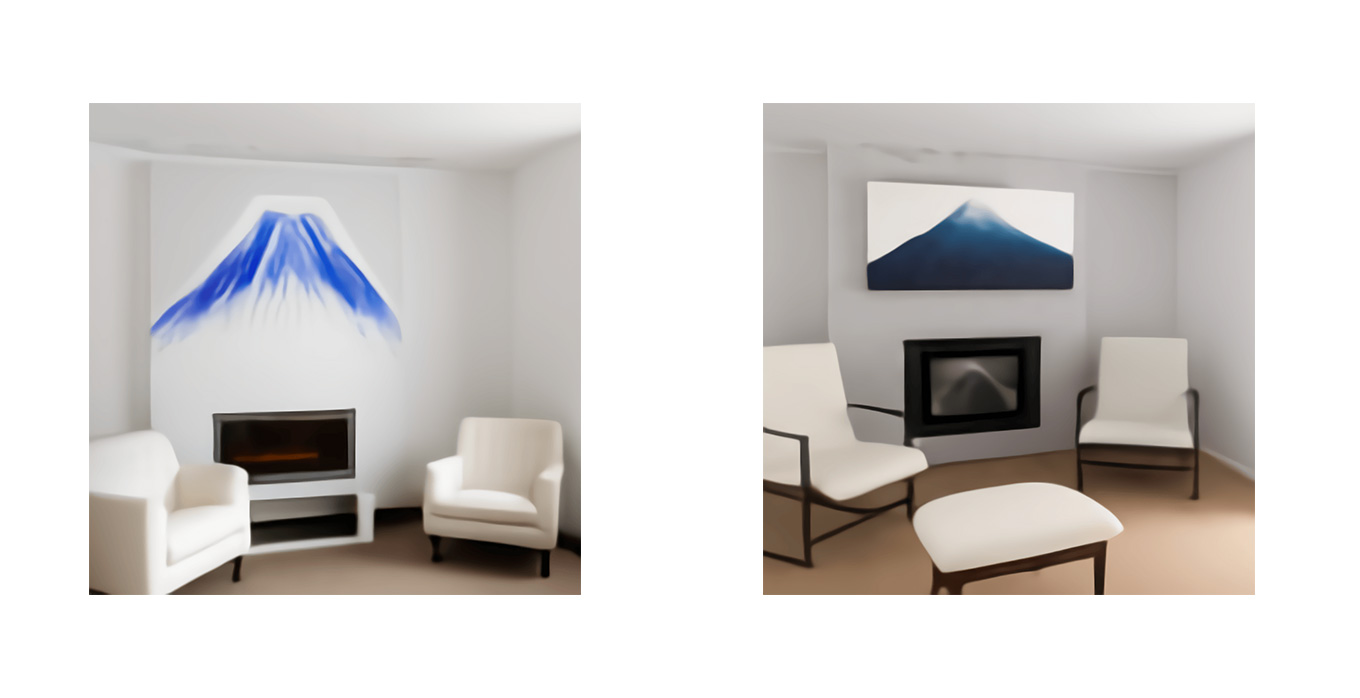
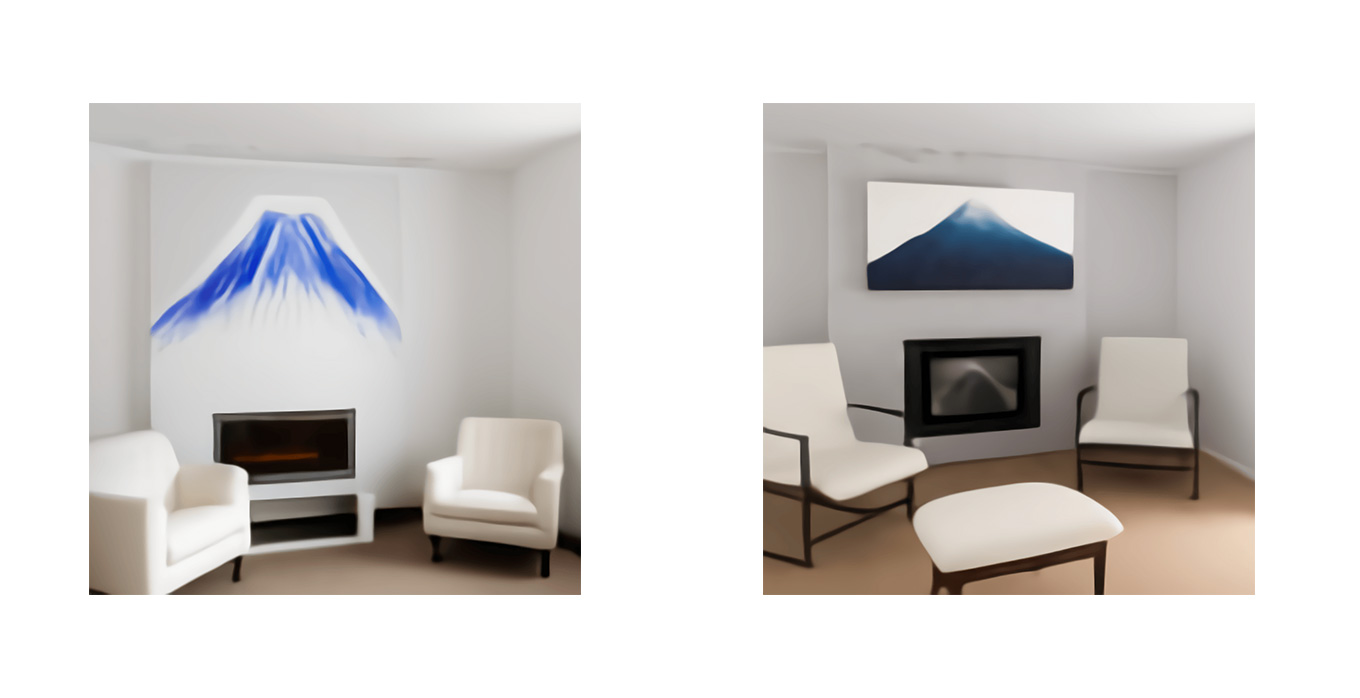
“A living room with two white armchairs and a painting of Mount Fuji. The painting is mounted above a modern fireplace.”
Images by OpenAI
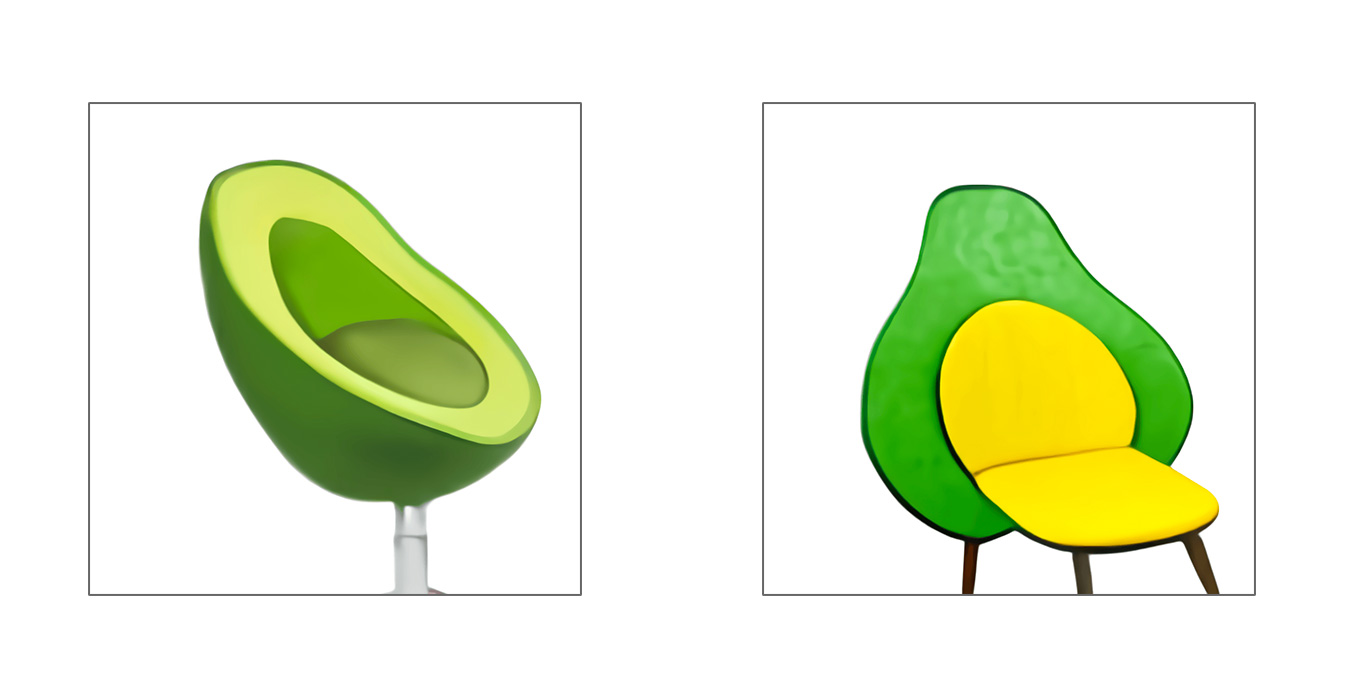
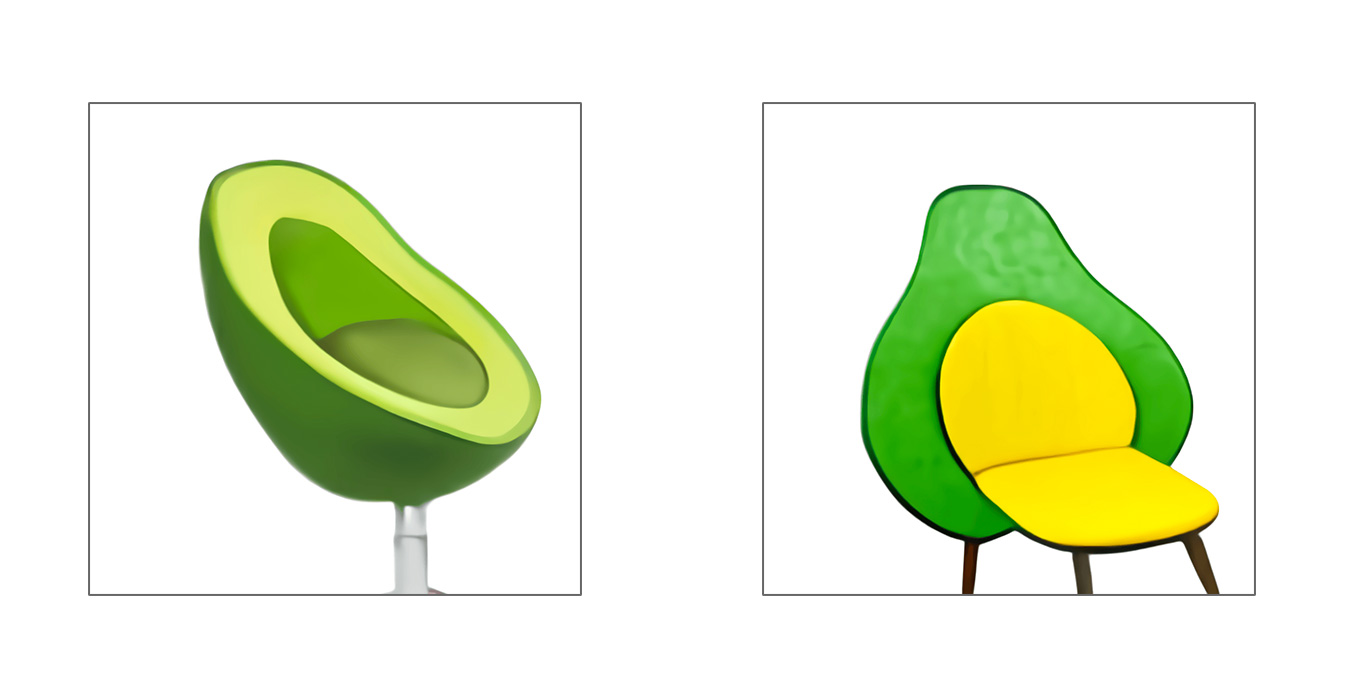
You could call this computational creativity. However, the natural limitation of the DALL-E model is that it can only generate subjects and concepts it has seen in its training data, since we are talking about a narrow AI after all.
Designing Finnish style coat of arms with AI
In Finland, every municipality has its own coat of arms which all share a common styling. Recently, hobbyists have designed coats of arms for different neighborhoods of Finnish cities which made it to the news too. Could you train a multimodal model, like DALL-E, to help you to design new Finnish-style coats of arms expressed in your natural language?
To keep this experiment compact, I used a technique called transfer learning to utilize an already pretrained multimodal model and then just further trained it with my own small dataset of Finnish municipality coat of arms images with text captions from Wikipedia. For the pretrained model, I chose OpenAI’s GLIDE, which is a partly open-sourced alternative to the DALL-E, which was actually never open-sourced.
After a few hours of training, the model learned the distinct styling and shape of Finnish coats of arms while trying to preserve the model’s original pretrained knowledge. As seen from the examples below, now we can combine the model’s original knowledge of different concepts with Finnish coat of arms styling to design completely new ones using our natural language.
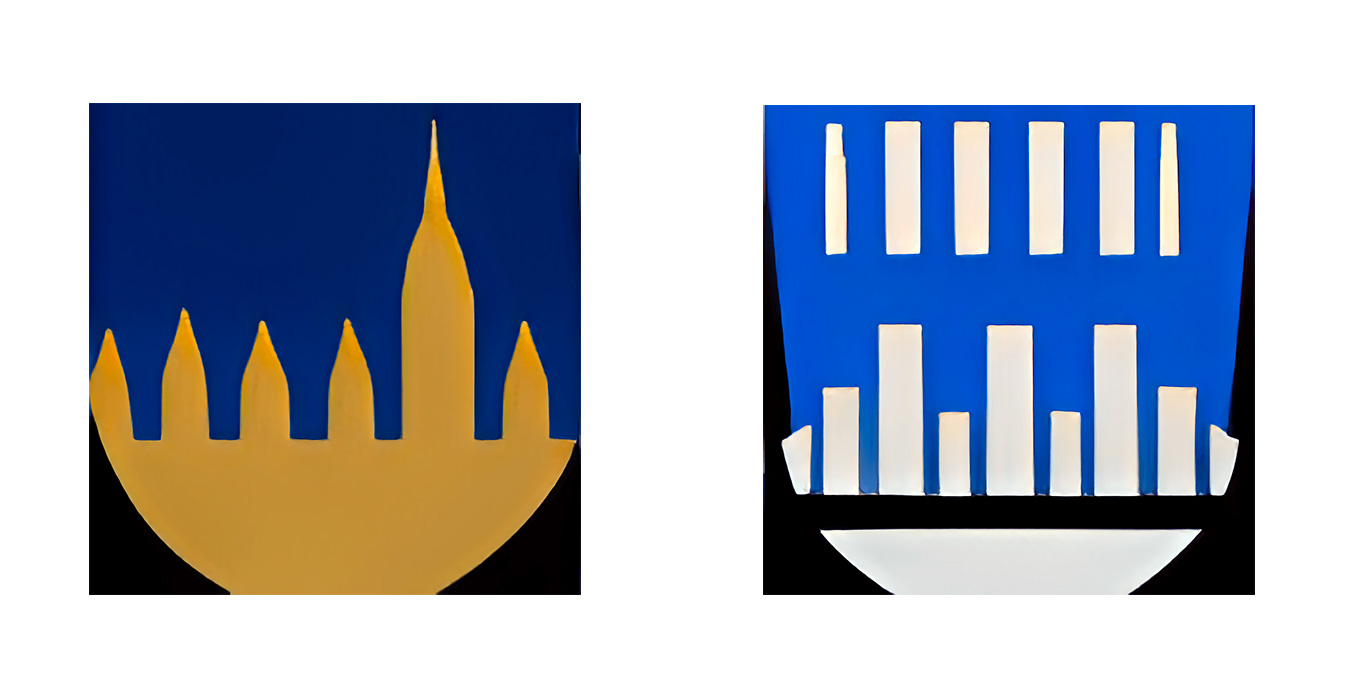
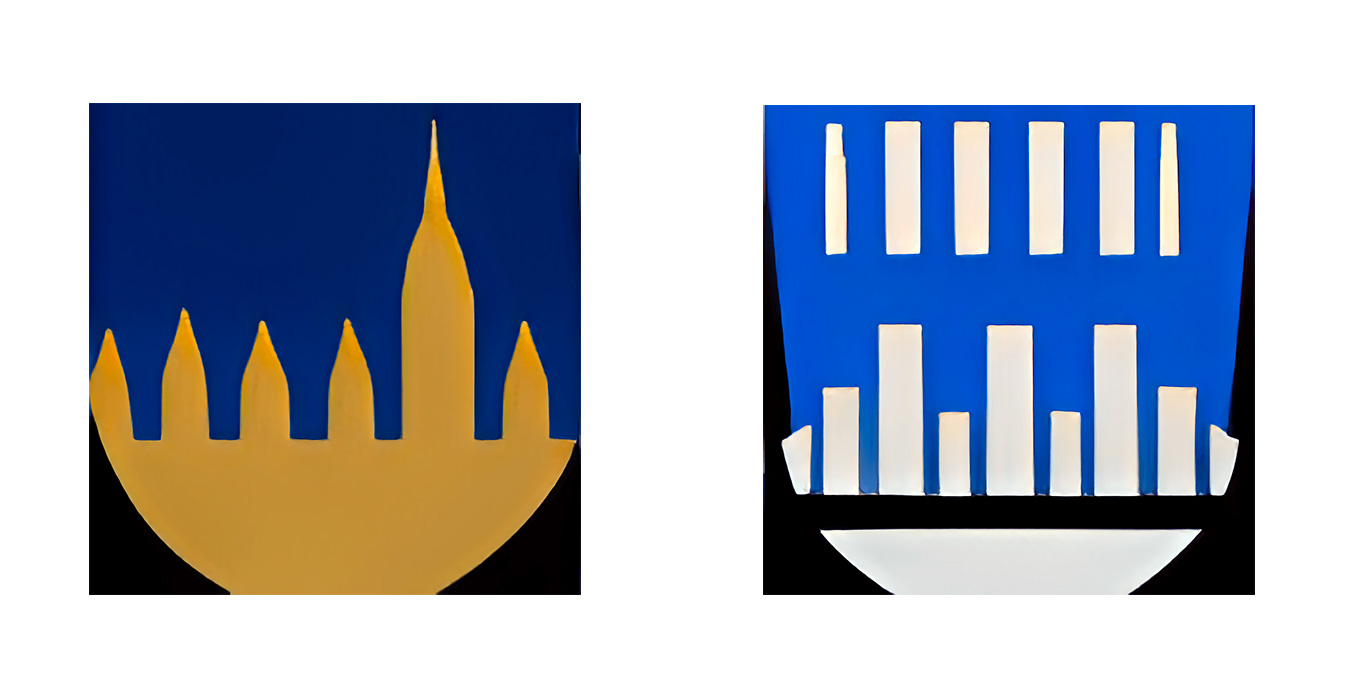
“A city skyline with skyscrapers on a coat of arms”
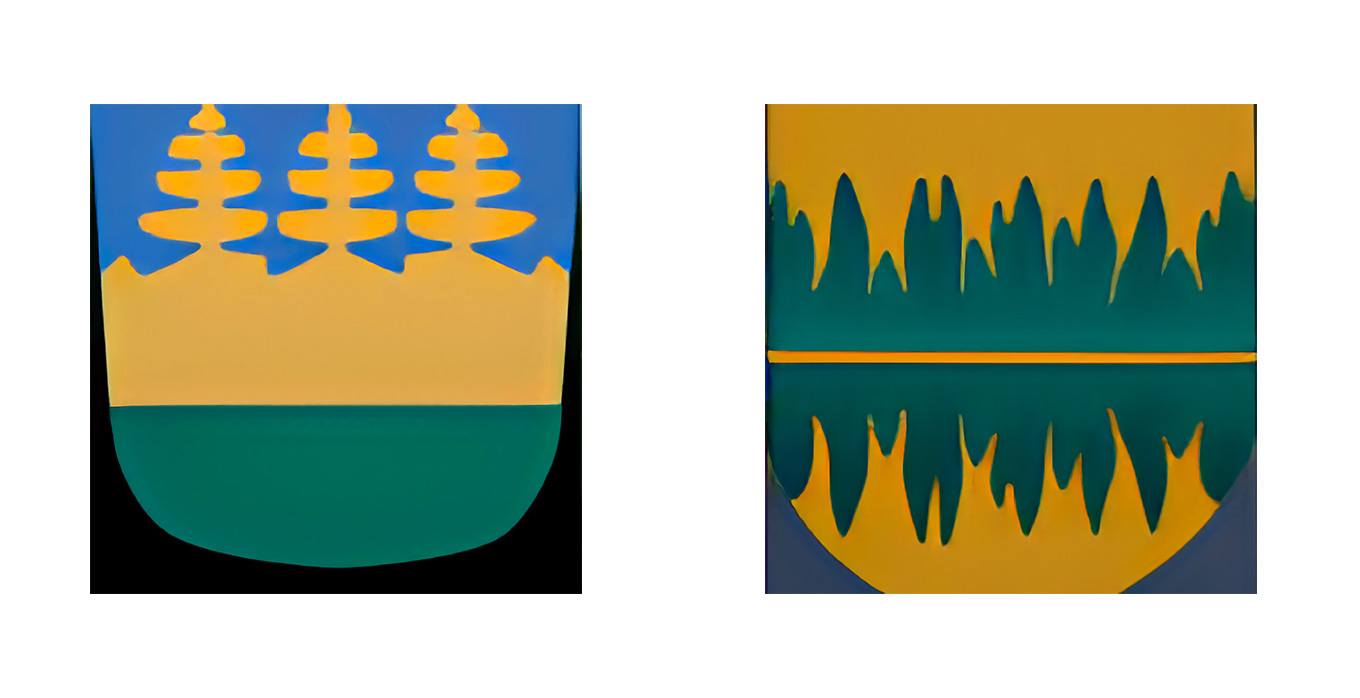
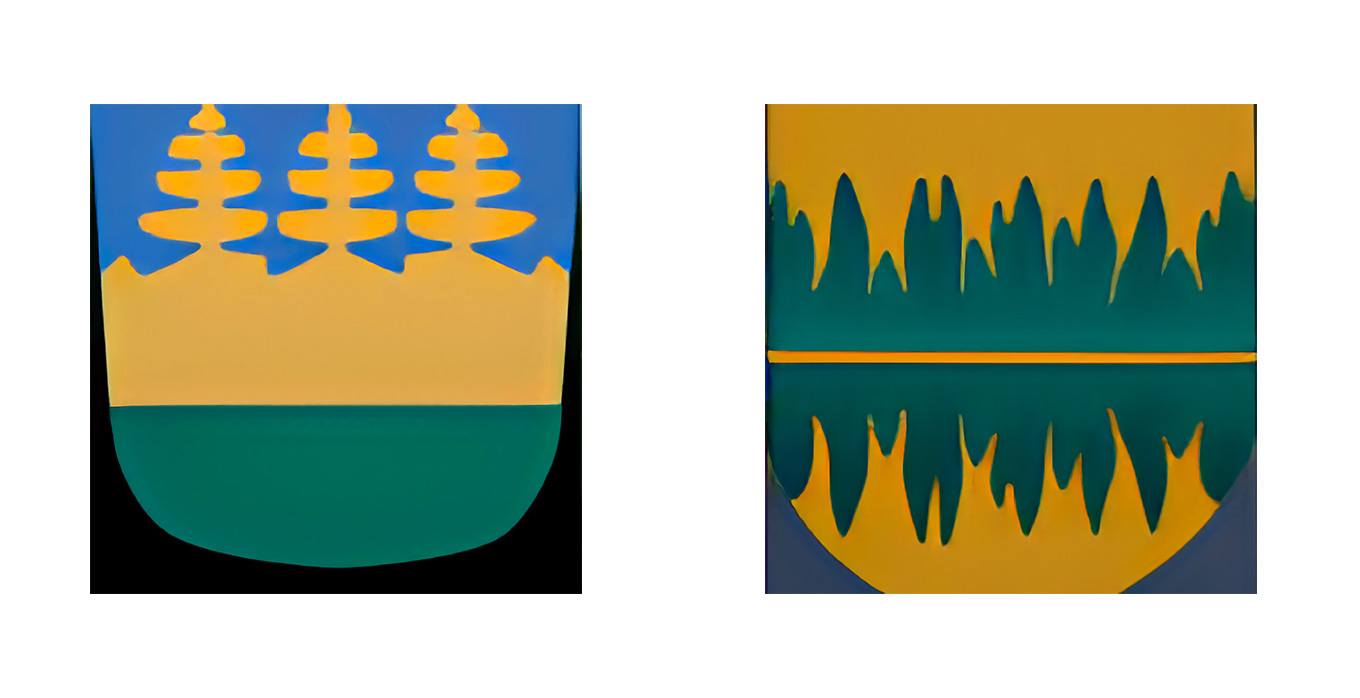
“A lake with forest on a coat of arms”
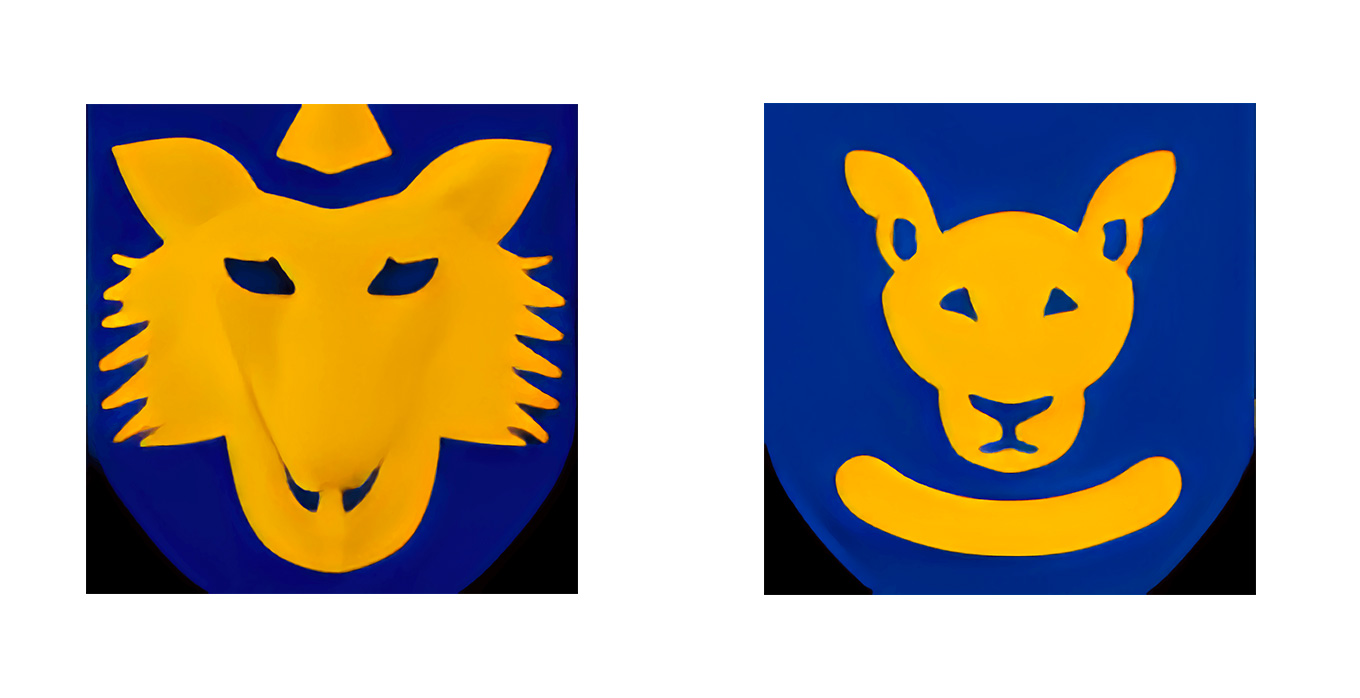
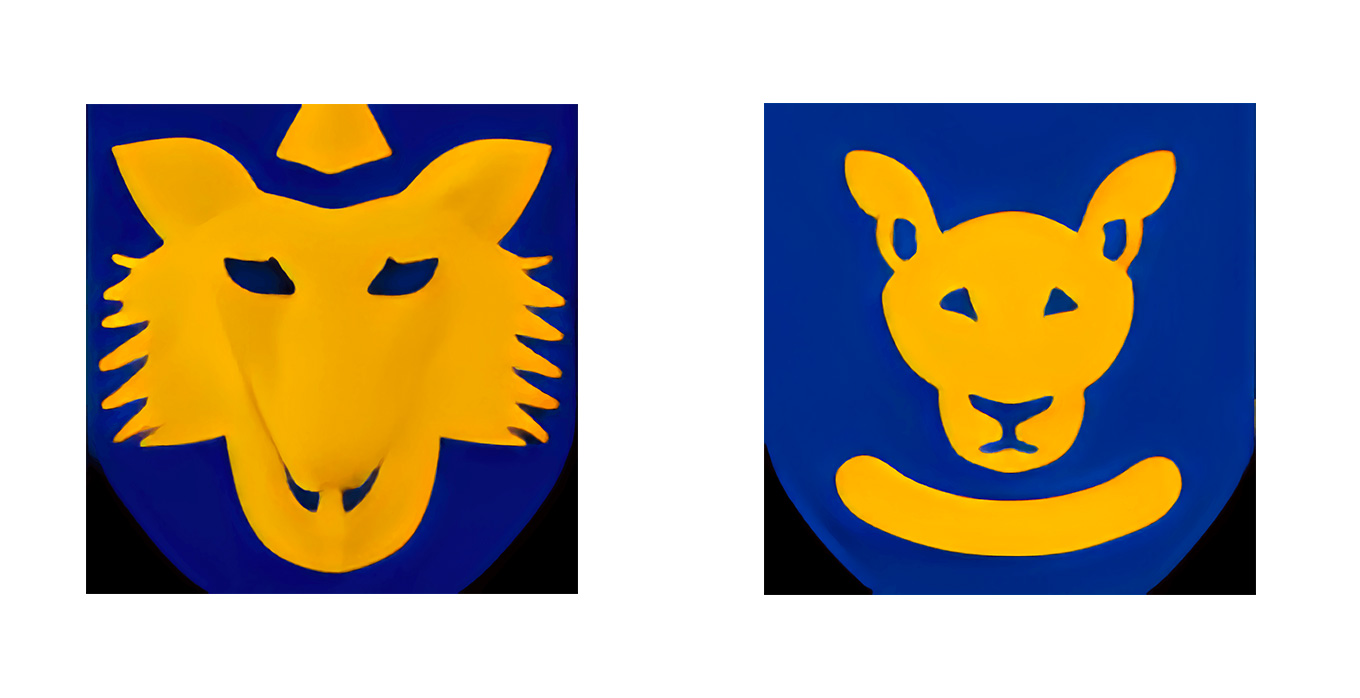
“A lion on a coat of arms”
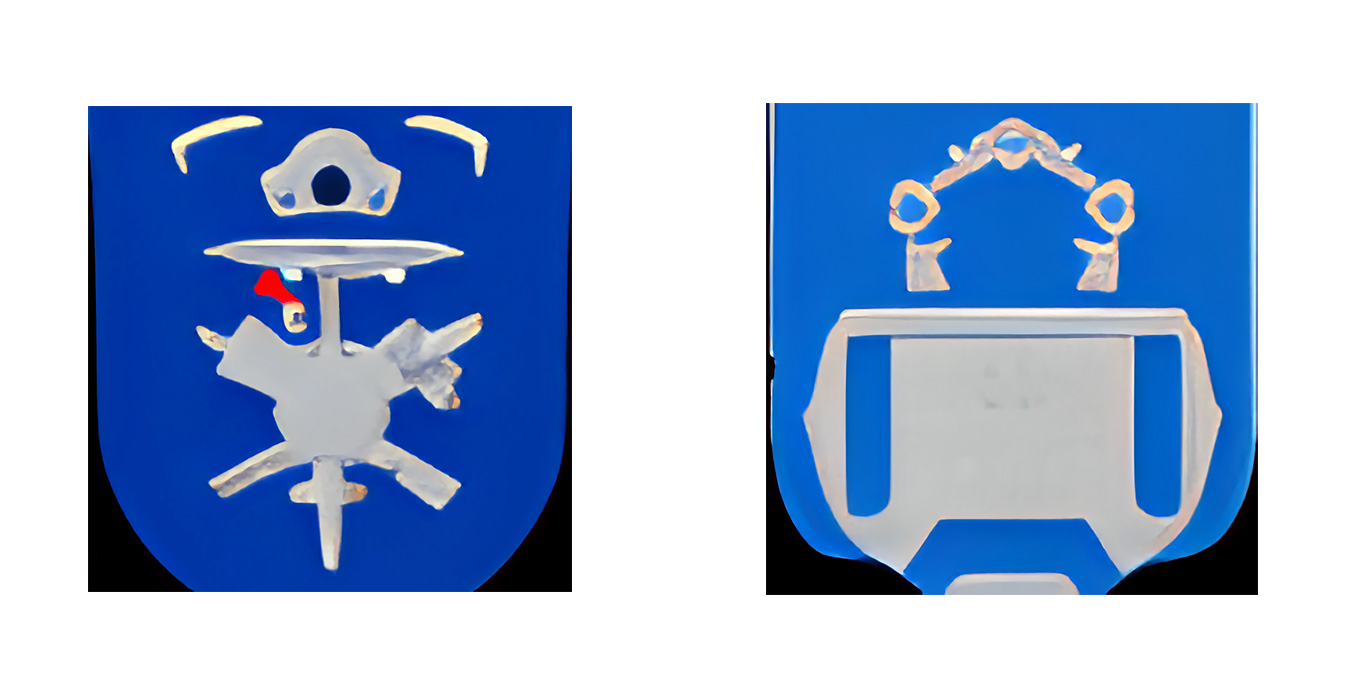
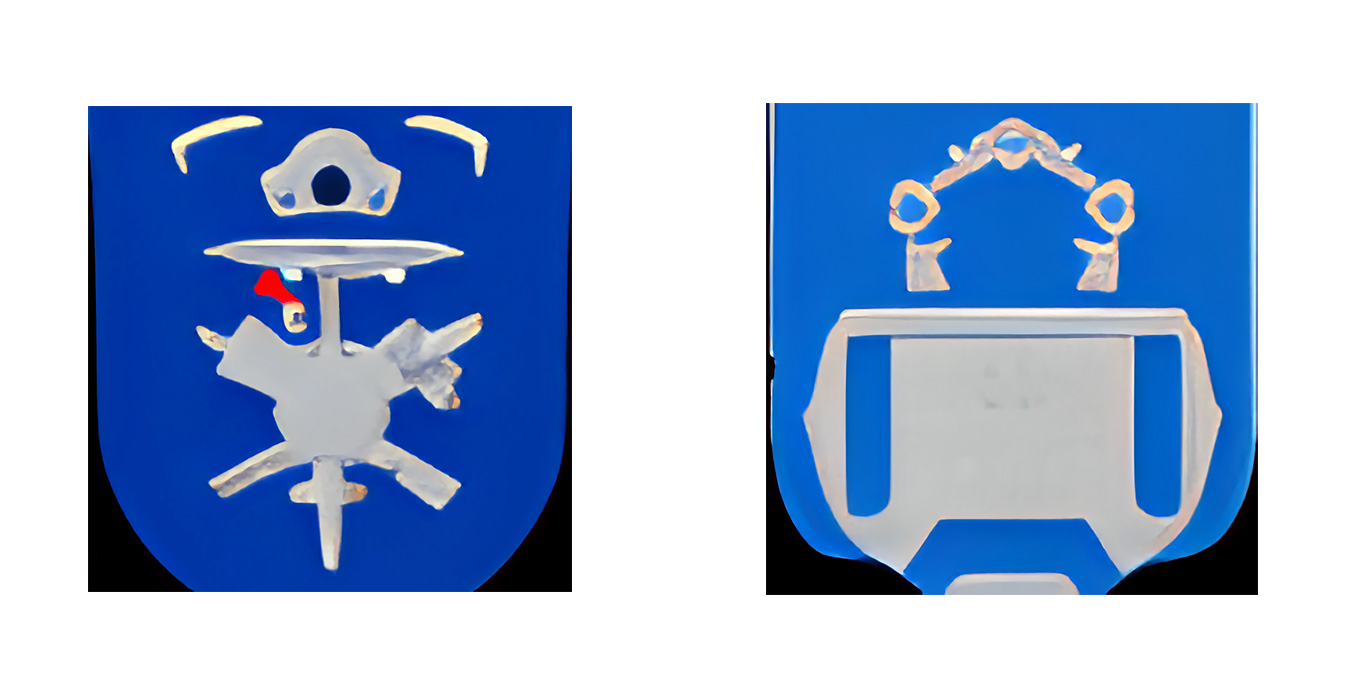
“A robot on a coat of arms”
Conclusion
Humans are really creative at coming up with totally new and even wacky concepts like “an armchair in the shape of an avocado”. In turn, computers are creative at coming up with syntheses from large amounts of data of even unrelated concepts.
By combining the powers of human and computational creativity, we can truly enrich our creative design processes.
In which areas would you like to see computational creativity being applied?
Disclaimer: The statements and opinions expressed in this article are those of the author(s) and do not necessarily reflect the positions of Thoughtworks.