“People are emotional, irrational and impulsive, but they also play a crucial part of any data strategy.” - Humanizing Data Strategy: Leading Data with the Head and the Heart, Tiankai Feng
With over 11 years of experience as a data professional across agency, corporate and consultancy roles, I’ve found that the human side of data is not only the most challenging but also the most rewarding aspect of the field.
While the importance of the human side of data is widely acknowledged in theory, there’s a surprising lack of practical guidance on how to navigate it. This gap inspired me to write Humanizing Data Strategy: Leading Data with the Head and the Heart, released in July 2024.
In the book, I introduce the 5Cs framework — competence, collaboration, communication, creativity and conscience — using real-life stories and examples to demonstrate how empathy and compassion can drive a sustainable data strategy.
At Thoughtworks, I’ve had the privilege of working with clients across various industries, and many of the challenges we address stem from human factors in data. Here are some practical ways to overcome these issues by humanizing your data strategy.
1. Pair data literacy with business acumen
Data literacy has been a key focus for the past decade, with organizations investing heavily in upskilling their workforce to read, analyze and communicate with data. While this is a significant step forward, it has been somewhat one-sided.
It’s not enough to train the entire organization in data literacy — data professionals must also develop their business acumen. Data can only drive real value when both sides understand each other, eliminating the pressure on business experts to bridge the gap alone.
However, improving business acumen needs to be targeted and cost-effective. It's crucial to identify the specific business domains where deeper knowledge is required for certain individuals while also building domain-agnostic expertise across teams.
The most effective way to enhance these skills is through a balanced approach. This involves blending internal training — demonstrating how theoretical concepts apply in practice within your organization — with external resources such as live or on-demand learning. This strategy strikes the right balance between general knowledge and organization-specific expertise.
2. Leverage co-creation beyond service and self-service
Data collaboration has evolved significantly over the past few years. We’ve moved from a centralized model, where a dedicated data team provides services to others, to fully decentralized approaches, and now toward hybrid frameworks like data mesh that aim to balance the best of both worlds.
While the principles behind service and self-service models are promising, in practice they often fall short. Centralized services can be slow and self-service capabilities may not meet expectations. This mismatch creates friction in data collaboration efforts.
The solution lies in shifting from a transactional service mentality to one rooted in trust and partnership: co-creation. Co-creation emphasizes two key elements — working together to create something new and fostering equal collaboration among all parties involved.
Regardless of your data operating model, fostering a co-creation mindset through shared goals, clear responsibilities and recognition for contributions can help ease collaboration challenges and drive a more motivated, unified effort toward data success.
3. Address real issues by "following the pain" for impact
The most effective way to foster a strong culture of collaboration around data is by directly addressing real business problems. All too often, data teams get caught up in the excitement of cutting-edge, state-of-the-art solutions while business teams are still grappling with basic data challenges that remain unresolved.
By embracing the philosophy of “following the pain,” data efforts can stay focused on solving tangible business issues while building stronger relationships and advocacy within the organization.
Here's how it works in three simple steps:
Identify a frustrated data stakeholder: Find someone in the organization who is facing ongoing data-related frustrations. (Trust me, there’s always someone!)
Clarify the problem: Collaborate with them to clearly define the problem. The person will likely have a strong sense of what the solution should look like, offering you precise requirements to work from.
Co-create the solution: Work together to build the solution. The business team will not only appreciate the immediate resolution but will also become strong advocates for future data initiatives. This leads naturally back to step one — find the next pain point and repeat the process!
Though it sounds simple, the common pitfall is that data teams often aren’t proactively engaging with business teams to discover these pain points. Changing this approach can significantly boost the impact and visibility of your data efforts.
4. Collaboratively discover your data purpose
There are numerous exciting opportunities to explore with data, but not all of them may be the most suitable for your organization. How can you identify the right data initiatives that will drive meaningful impact?
Inspired by the Japanese philosophy of “Ikigai” — finding your purpose or reason for living — here’s a framework to help you uncover your organization’s “data purpose”:
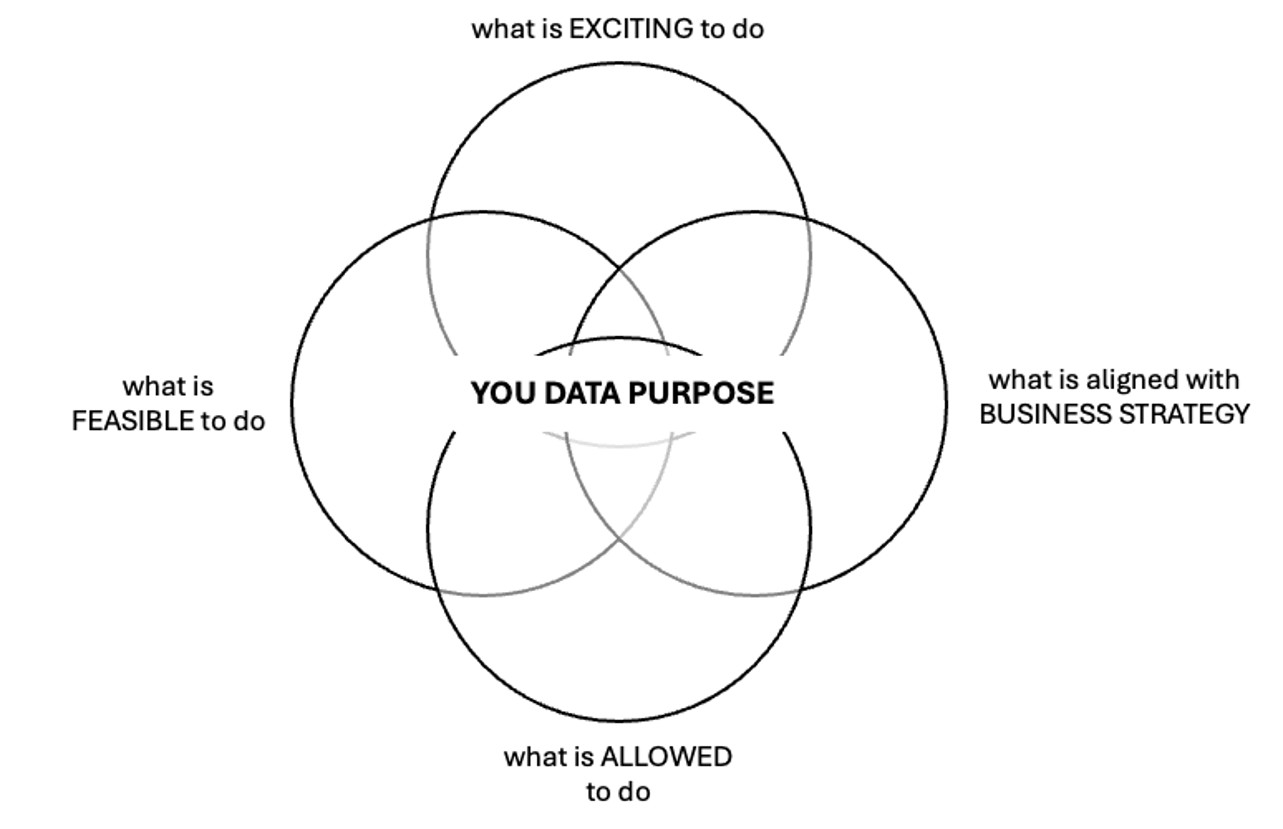
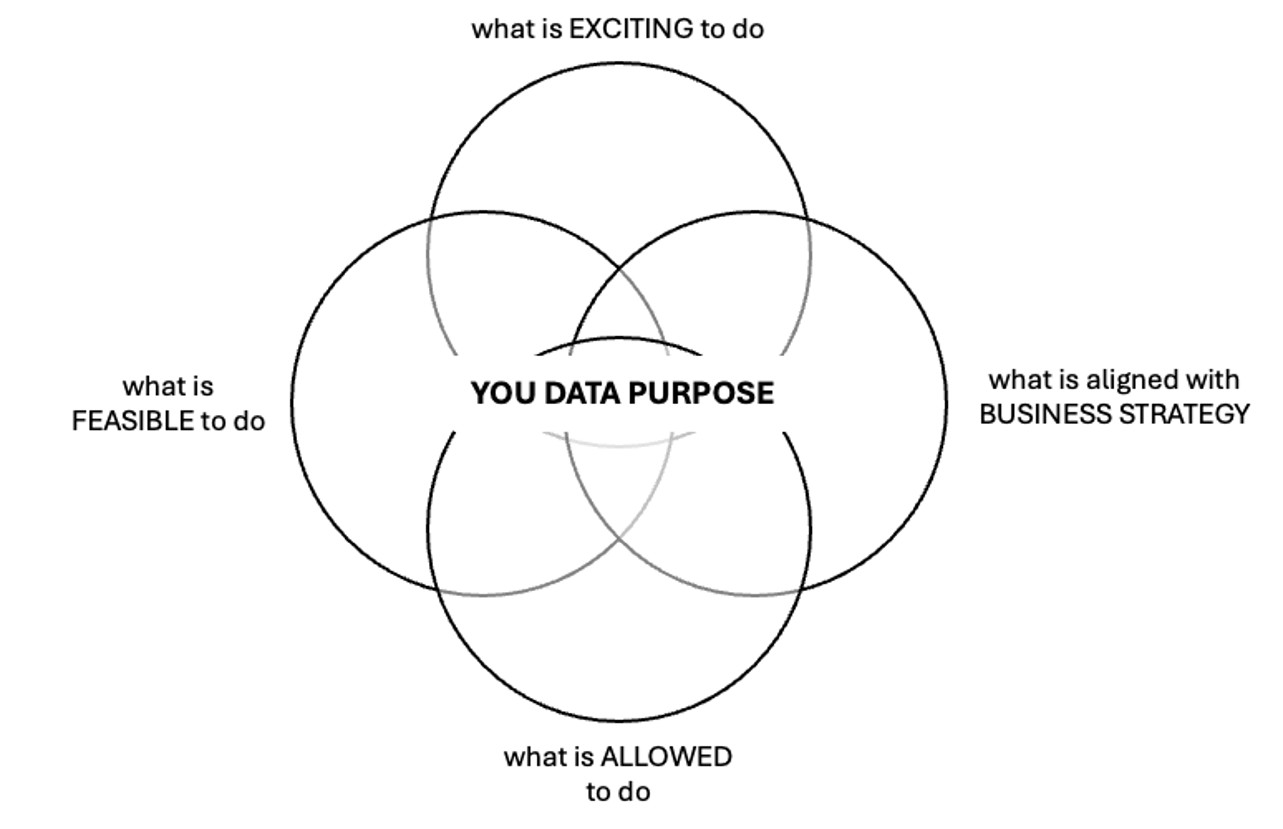
What is EXCITING: Everyone has unique ideas about what to do with data, driven by their individual passions and interests.
What is ALIGNED WITH BUSINESS STRATEGY: Ensure that your data initiatives align with the organization’s business objectives and strategic goals.
What is ALLOWED: Consider both internal and external constraints, typically arising from regulations and policies.
What is FEASIBLE: Conduct an honest assessment of your current maturity and capabilities to implement the necessary technical solutions.
By evaluating your data decisions against these four criteria, you can engage various stakeholders from business, legal and IT departments. This collaborative approach helps define the right data purpose upfront, preventing the discovery of unexpected limitations and risks during implementation.
5. Align organizational and personal data value perspectives
Everyone recognizes the value of data and numerous methods exist to calculate or estimate its actual worth. While the organizational value of data — often expressed in financial metrics — is undeniable and important, it often fails to inspire people to take action.
This disconnect arises because we do not delve deeply enough to understand the personal motivations of different contributors. Their individual ambitions often do not align with the rational presentation of data value.
For instance, while higher profitability is a clear business goal tied to increased productivity, the personal benefit may be that certain individuals finally spend less time on tedious tasks, such as manual data cleaning, allowing them to focus on more meaningful work.
To foster genuine engagement, it’s essential to reframe business value into personal value, addressing the specific needs and ambitions of key stakeholders. This approach transforms rational involvement into emotional commitment, which is a powerful catalyst for successfully implementing any data strategy.
6. Harness creativity as a driver for innovation
Picasso once said, "The enemy of creativity is common sense," a sentiment that particularly resonates in the field of data, even if it isn’t usually seen as a field where creativity is important. However, all organizational and technological innovations stem from human creativity; it’s a muscle we all possess but often underutilize.
To harness the creativity of data professionals for innovation, data leaders must focus on three aspects:
Mindset: Encourage creativity as essential for innovation and growth within any data strategy.
Practice: Provide space and opportunities for experimentation and foster psychological safety to embrace failure.
Habit: Establish creativity as the default by regularly acknowledging successes and best practices.
Ultimately, the key to creativity lies in translating abstract concepts into actionable steps through meaningful interactions with all contributors to a data strategy.
7. Manage data responsibly through cross-functional collaboration
"With great power comes great responsibility" — a saying from the Spiderman comics — aptly applies to data. While having vast amounts of data grants significant power, it also brings risks that must be addressed responsibly.
Today, we can categorize data concerns into four key types:
Data privacy: Managing personally identifiable information (PII) securely and ethically.
Data security: Preventing sensitive information from falling into the wrong hands to avoid irreversible damage.
Data ethics: Ensuring data usage is ethical to prevent discrimination against specific groups.
Ungoverned AI: Training AI models with unbiased data to avoid harm to individuals or society.
These concerns are increasingly pressing, and it’s unrealistic to expect a few individuals to address them all. Cross-functional oversight involving stakeholders from HR, legal, information security and IT is essential for a comprehensive approach to risk prevention.
While the drive for innovation and cost savings may tempt us to automate and remove humans from the process, having human oversight — especially at the outset — is crucial. Only as data maturity and confidence grow should we gradually reduce human involvement.
Final reflection
In the realm of data strategy, let automation handle tedious and routine tasks, while we retain critical thinking and human interaction. The future of data is in our (human) hands. Good luck!
All content in this blog post is inspired by the book Humanizing Data Strategy: Leading Data with the Head and the Heart, by Tiankai Feng.
Disclaimer: The statements and opinions expressed in this article are those of the author(s) and do not necessarily reflect the positions of Thoughtworks.