Anonymesh is a concept we’ve been developing inside Thoughtworks. As Rajat Jain outlined in an article published last year, it’s a technique that addresses the challenge of easier data sharing and privacy: something often described as the privacy vs. utility trade-off.
We think it has the potential to play a significant role in how the world thinks about data in an era of growing privacy awareness and regulation. But it can only have an impact if the story told about it is clear and compelling. So, I spoke to Thoughtworks’ Global Head of Data Protection and Privacy, Erin Nicholson — who has helped develop the idea while working with a number of UK public sector clients — to get her perspective on anonymesh and to explain what it is and how it works.
What is anonymesh?
Richard Gall: What is anonymesh? Is it a method? An architectural pattern?
Erin Nicholson: Similar to data mesh, anonymesh is a method which focuses on decentralization and keeping data at source. By doing this, it facilitates secure information sharing among organizations, which is of particular importance in the public sector. It employs domain-driven design to address concerns such as trust boundaries, cost-effective data management and — most importantly, in my opinion — privacy.
By revealing only essential data and leveraging advanced privacy technologies like secure multi-party computation and federated analytics, anonymesh ensures secure connections between disparate data sets while respecting privacy principles without the need for new data warehouses which are expensive and unpopular with the public.
RG: What problem does anonymesh solve? And could you give an example of the sort of situation that led you to develop it (ie. what did you see happening?)
EN: I started my career in social work. My very first job was an admin assistant in a social work office, so I saw first hand the issues social workers face every day. Some of those issues are caused by data not being shared; not having key information when you need it can have a huge detrimental effect on the children who are being looked after.
If you look at the serious case reviews when something does go tragically wrong, almost every review has data sharing — or a lack of it — as a key element. People on the front line often don’t feel comfortable sharing as they have to make complex decisions based on data protection laws, safeguarding rules and data sharing agreements which they don’t have much visibility on. We designed anonymesh to ensure there are some inbuilt rules so if you picked from a drop down of reasons why information was being queried, for example, these would track back to the data sharing agreement, and reveal the information (if it was there). This takes the risk away from the practitioner and just gives them the information.
Thoughtworks developed something akin to this for Stockport Council, called Signposts. Signposts was a great success in bringing data sets together, but we couldn’t get as many data sets as we wanted to because data still had to cross a trust boundary. At the time, privacy enhancing technologies weren’t where they are today, which meant we couldn’t employ secure multi-party computation like we have with anonymesh. Signposts, and the learnings and success from it, really helped us develop the anonymesh proof of concept.
Anonymesh and data mesh
RG: How should we think about anonymesh in relation to data mesh?
EN: Both are decentralized approaches, which enables teams to perform cross-domain data analysis. However, with anonymesh it is across organizations (or within an organization if the data is highly confidential), and I believe it utilizes edge computing more than in data mesh; this is because the analysis needs to be done by an aggregator before it returns a query in order to ensure privacy of the remainder of people in the data set. We took the data mesh idea and built upon it, keeping privacy at the heart of that design.
RG: There are a number of interesting new techniques in the data and privacy field (like federated learning). How do these fit into anonymesh (if at all)?
EN: Anonymesh utilizes federated learning, multi-party secure computation, and edge computing. All of these techniques weren’t readily available when we looked at this problem in 2017 and so to have the opportunity to use them now is extremely exciting.
RG: Data clean rooms are an emerging product set aimed at tackling privacy issues. How does this fit into the anonymesh picture? Is anonymesh a response to this new class of products?
EN: I’m no expert in data clean rooms, but I believe those work with aggregated data, or at least where the query response would be aggregated. The use cases we’re looking at are where the query would be on an individual level. For things like public health data, clean rooms are an interesting proposition, but I can’t help but think you are just moving the problem elsewhere.
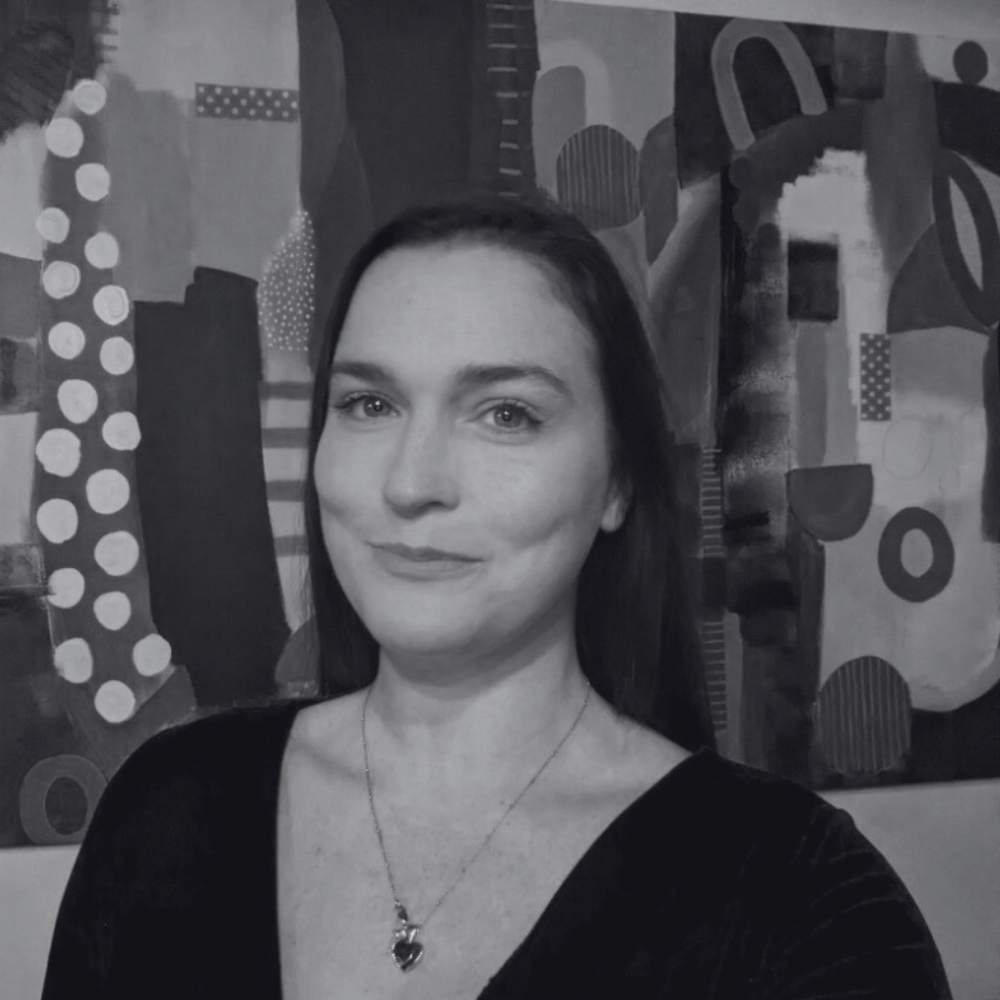
Anonymesh not only facilitates more efficient data sharing across and within organizations but also enhances the privacy and security of sensitive information.
Anonymesh not only facilitates more efficient data sharing across and within organizations but also enhances the privacy and security of sensitive information.
Addressing a changing regulatory landscape
RG: There's obviously some important legal dimensions and considerations at play in anonymesh. Could you explain what they are and how anonymesh is designed to respond to them?
EN: The legal aspects, while long-winded and difficult, are often agreed at a data sharing agreement level, between senior stakeholders and in-house legal teams. Often, what’s agreed in the data sharing agreements doesn’t reflect how the data is stored on the ground or how it can be shared technically.
There is a legal impetus to share — let's take the social care use case in the UK as an example. Here, there is a requirement for public bodies to share information for the purpose of safeguarding (in the Children’s Act 2004) as long as one applies data minimization and follows relevant data protection laws (in the UK there’s GDPR, for instance). The Information Commissioner’s Office (ICO) in the UK has said multiple times that people need to share this data, yet we continue to see the lack of data sharing as a barrier in most serious case reviews. This is often because people didn’t know how to share or didn’t feel comfortable — they thought they were doing the right thing. This really needs to change and we need to empower people on the front line to share data in a way which they know is allowed and won’t get them in hot water.
So, I suppose my answer is that whilst the legal aspect is complex and data sharing agreements can be a nightmare to establish, it’s the actual act of sharing by the end user where we need to see changes. Anonymesh is ultimately designed to help that person be secure and confident in that the information can be shared, and then able to share it in a way which maintains privacy.
RG: One final question: How would you summarize anonymesh? What’s the main pitch for anyone reading this?
EN: I’d put it like this: Anonymesh represents a forward-thinking solution to the perennial challenge of balancing data utility with privacy. By integrating advanced privacy technologies and a decentralized architectural approach, Anonymesh not only facilitates more efficient data sharing across and within organizations but also enhances the privacy and security of sensitive information.
Thanks to Erin for taking the time to talk to me. If you’d like to learn more about anonymesh, read Rajat Jain’s article here.
Disclaimer: The statements and opinions expressed in this article are those of the author(s) and do not necessarily reflect the positions of Thoughtworks.