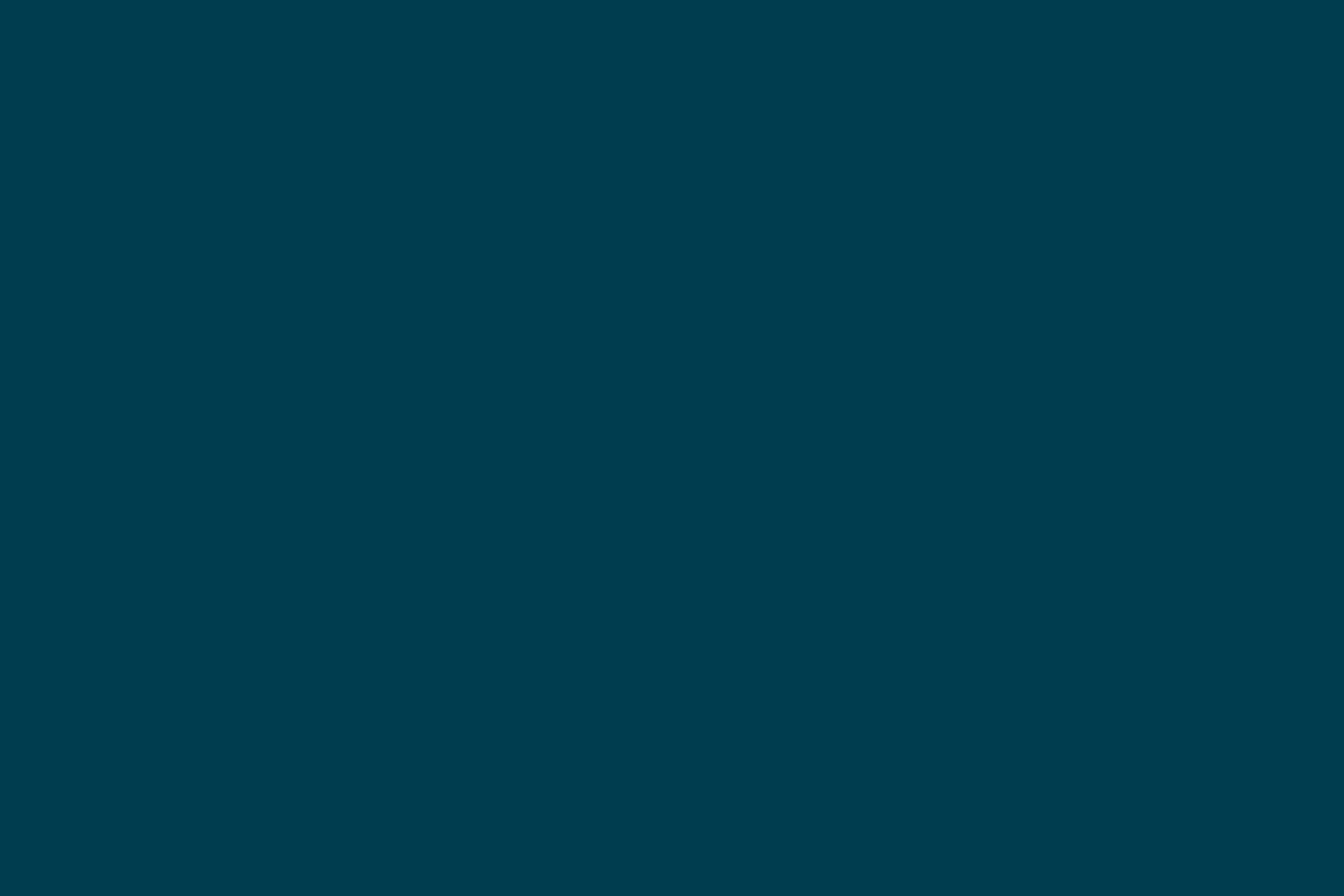
Data Science and Big Data: Two Very Different Beasts
It is difficult to overstate the importance of data in today's economy. The tools we use and actions we take consume and generate a digital version of our world, all captured, waiting to be used. Data have become a real resource of interest across most industries and is rightly considered the gateway to competitive advantage and disruptive strategy.
Along with the rise of data has come two distinct efforts concerned with harnessing its potential. One is called Data Science and the other Big Data. These terms are often used interchangeably despite having fundamentally different roles to play in bringing the potential of data to the doorstep of an organization.
Although some would argue there is still confusion over the terms data science and big data, this has more to do with marketing interests than an honest look at what these terms have come to mean on real-world projects. Data science looks to create models that capture the underlying patterns of complex systems, and codify those models into working applications. Big data looks to collect and manage large amounts of varied data to serve large scale web applications and vast sensor networks.
Although both offer the potential to produce value from data, the fundamental difference between data science and big data can be summarized in one statement:
Despite this declaration being obvious, its truth is often overlooked in the rush to fit a company's arsenal with data-savvy technologies. Value is too often framed as something that increases solely by the collection of more data. This means investments in data-focused activities center around tools instead of approaches. The engineering cart gets put before the scientific horse, leaving an organization with a big set of tools, and a small amount of knowledge on how to convert data into something useful.
Bringing Ore to an Empty Workshop
Since the onset of the Iron Age, blacksmiths have used their skills and expertise to turn raw extracted material into a variety of valuable products. Using domain specific tools, the Blacksmith forges, draws, bends, punches and welds the raw material into objects of great utility. Through years of research, trial and error the blacksmith learned to use choice gases, specific temperatures, controlled atmospheres and varied ore sources to yield a tailored product bespoke to its unique application.
With the Industrial Revolution came the ability to convert raw material into valuable products more efficiently and at scale. But the focus on scaling wasn't the acquisition of more material. It was on building tools that scaled and mechanized the expertise in converting. With this mechanization came an even greater need to understand the craft since to effectively operate, maintain and innovate at scale one had to deeply understand the process of converting raw material into products that answered to the always-changing demands of the market.
In the world of data this expertise in converting is called data science. The reason it takes a science to convert a raw resource into something of value is because what is extracted from the 'ground' is never in a useful form. 'Data in the raw' is littered with useless noise, irrelevant information, and misleading patterns. To convert this into that precious thing we are after requires a study of its properties and the discovery of a working model that captures the behavior we are interested in. Being in possession of a model despite the noise means an organization now owns the beginnings of further discovery and innovation. Something unique to their business that has given them the knowledge of what to look for, and the codified descriptions of a world that can now be mechanized and scaled.
Conversion Should Scale Before Collection
No industry would invest in the extraction of a resource without the expertise in place to turn that resource into value. In any industry, that would be considered a bad venture. Loading the truck with ore only to have it arrive at an empty workshop adds little strategic benefit.
An unfortunate aspect of big data is that we look to the largest companies to see what solutions they have engineered to compete in their markets. But these companies hardly represent the challenges faced by most organizations. Their dominance often means they face very different competition and their engineering is done predominantly to serve large-scale applications. This engineering is critical for daily operations, and answering to the demands of high throughput and fault-tolerant architectures. But it says very little about the ability to discover and convert what is collected into valuable models that capture the driving forces behind how their markets operate. The ability to explain and predict an organization's dynamic environment is what it means to compete using data.
Understanding the distinction between data science and big data is critical to investing in a sound data strategy. For organizations looking to utilize their data as a competitive asset, the initial investment should be focused on converting data into value. The focus should be on the data science needed to build models that move data from raw to relevant. With time, big data approaches can work in concert with data science. The increased variety of data extracted can help make new discoveries or improve an existing model's ability to predict or classify.
Fill the workshop with the skills and expertise needed to convert data into something useful. The ore brought here will become the products that define a business.
An earlier version of this article was first published on KDNuggets.
Disclaimer: The statements and opinions expressed in this article are those of the author(s) and do not necessarily reflect the positions of Thoughtworks.