Realizing value from data and AI platforms
The infrastructure supporting growth and innovation
Reliable access to credible, up-to-date data is now critical to virtually everything enterprises need to do, as underscored by the rapid rise of artificial intelligence (AI) — including generative AI (GenAI) — in business operations and decision-making. Even if organizations aren’t planning on building GenAI applications in the short term, the availability of high-quality data is still a prerequisite for the delivery of other digital initiatives and services.
While data is creating unparalleled opportunities for businesses, the accompanying challenges often prove too big to ignore. Many companies remain bound by internal silos and multiple isolated data platforms that leave valuable intelligence locked up and hard to use.
Turning data stores into strategic assets requires focus on making data findable, accessible, trustworthy, interoperable and reusable, all in a secure and privacy-sensitive fashion. Data platforms provide the only viable foundation for this approach.
The term “platform” means many different things, although in all cases we recommend a product thinking mindset. By integrating various data resources and ensuring they can be seamlessly accessed and applied, they provide the different building blocks now needed to form a comprehensive digital strategy.
Once in place, your data platform enables you to gather data insights, create reliable AI systems, control risk and much more. Your data platform can also be a key component in creating, managing and enforcing data governance, one of the biggest challenges faced by many organizations.
Having a robust data platform which facilitates open sharing while preserving privacy allows enterprises to participate with other organizations in thriving data ecosystems to produce greater industry, even societal, impact. This is a key trend we see expanding over the next few years that could facilitate more digital innovation and potentially create a sea change in how data is stored and exchanged — but only if a shift towards standardization takes place and enterprises learn to guard their data assets less jealously.
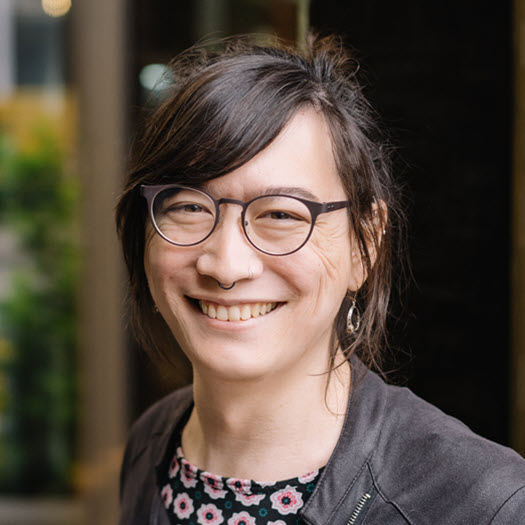
Data doesn’t carry any intrinsic value on its own. Its value relies on you having a purpose — and a process — for it.
Data doesn’t carry any intrinsic value on its own. Its value relies on you having a purpose — and a process — for it.
Signals
A rise of integrated data and AI platforms. These systems present the analysis as the primary benefit, and the data just comes along. This represents a fundamental change in the way of thinking about these solutions.
Data ecosystems going beyond the hype to generate tangible business results. Tech research and advisory firm Gartner sees data ecosystems moving past the peak of the hype cycle and firmly entering the mainstream within the next decade. Our experience on the ground points to the same trend, with growing demand for organizations to share and pool data resources — enterprises are today proving more willing to take the plunge.
Data ‘clean rooms’ gaining popularity. A number of vendors including Infosum, AWS, Google and Snowflake have developed data ‘clean room’ offerings designed specifically to enable the secure intra- and inter-organizational sharing of privacy-compliant, anonymized data efficiently and at scale.
The creation of open data sharing standards and infrastructure gathering momentum. As exhibited by initiatives like the Open Data Standard for the Apparel Sector and OSDU™ Forum, some organizations are attempting to circumvent the data interoperability challenges perpetuated by incumbent vendors by championing open protocols for the secure exchange of valuable data on supply chains and other industry-critical functions.
Mechanisms for privacy-aware sharing of user data. Data privacy is increasing its mindshare among users. We have developed a solution called Anonymesh to help address this challenge. Organizations are also building personal data stores such as Solid Pods that organize data storage around users, instead of the organizations that collect them.
Governments setting the open data agenda. Countries worldwide are embracing open data initiatives that encourage free access and the use of government-collected information to improve public services and create economic opportunities. The UK government, for example, is building an Integrated Data Service (IDS) to facilitate cross-departmental data exchange. Meanwhile, Singapore’s open data initiative enables developers to use real-time datasets from government agencies to develop their own applications.
Trends to watch
Adopt
-
Techniques to draw cause and effect relationships between the input data and the outcomes of a machine learning model, which allows a model to be more generalizable and require less training data to perform effectively.
-
A formal agreement between two parties – producer and consumer – to use a dataset or data product.
-
Also known as self-sovereign identity, decentralized identity (DiD) is an open-standards-based identity architecture that uses self-owned and independent digital IDs and verifiable credentials to transmit trusted data. Although not dependent on blockchains, many current examples are deployed on them as well as other forms of distributed ledger technology, and private/public key cryptography, it seeks to protect the privacy of and secure online interactions.
-
More granular access controls for data, such as policy-based (PBAC) or attribute-based (ABAC) that can apply more contextual elements when deciding who has access to data.
-
Systems, both human and machine, originally designed to be decentralized have become more centralized over time. Re-decentralization refers to the conscious effort of moving those systems back to a decentralized model.
Analyze
-
Technologies enabling the direct interaction of devices and information sharing between them, usually in an autonomous fashion. This enables to decision making and action with little or no human intervention.
-
An emerging set of techniques to certify the provenance of data and to govern its use across an organization. This could prove transformative in the effort to track and enhance progress towards sustainability targets.
Anticipate
-
Tools and techniques are emerging that support incorporating responsible tech into software delivery processes, primarily focusing on actively seeking to incorporate under-represented perspectives; some examples include Tarot Cards of Tech, Consequence Scanning, and Agile Threat Modeling.
Adopt
-
A precise technical description of a data product that enables its provisioning, configuration, and governance.
Analyze
Anticipate
-
A data architecture style where individuals control their own data in a decentralized manner, allowing access on a per-usage bases (for example, Solid PODs).
The opportunities
By getting ahead of the curve on this lens, organizations can:
Enable AI and GenAI initiatives. High quality data is a fundamental requirement for any artificial intelligence initiative. Forbes called data quality “the real bottleneck in AI adoption”.
Improve compliance posture and reduce risk. With automated embedded governance policies created and enforced via the underlying platform, you reduce the gap between written polices and what actually gets implemented on the ground.
Achieve significant savings by eliminating redundancy. Enhanced data sharing and creating a platform that provides a single point of availability allows organizations to retire tech infrastructure that’s duplicated across different parts of the organization, substantially reducing the costs of technology infrastructure and upkeep.
Gain a competitive advantage with improved insights. Integrating quality data across the enterprise can highlight previously unnoticed areas of inefficiency or friction, as well as provide a more holistic view of complex processes like the supply chain or customer journey. The resulting context and discoveries can help the organization understand their workflows and customers better, granting the enterprise an edge over competitors whose data resources remain more piecemeal and isolated.
Develop new sources of value. As data interoperability capabilities mature and open standards gain more widespread acceptance, more opportunities to capitalize on data assets will arise in the form of data marketplaces and networks. While some large organizations and industries have begun creating their own data ecosystems — such as those emerging around open banking and data sharing in insurance — there is still room to expand these to other sectors and the wider community.
Ramp up time-to-market. The availability of high quality data, especially as a basis for GenAI, is poised to accelerate and enhance many painstaking aspects of the product development process. One example is how the ability to sift through and summarize vast amounts of information and create synthetic customer data is helping organizations substantially reduce the lead time needed for market research. We believe large enterprises will have an edge over startups in pursuing these opportunities if their vast data reserves are used effectively.
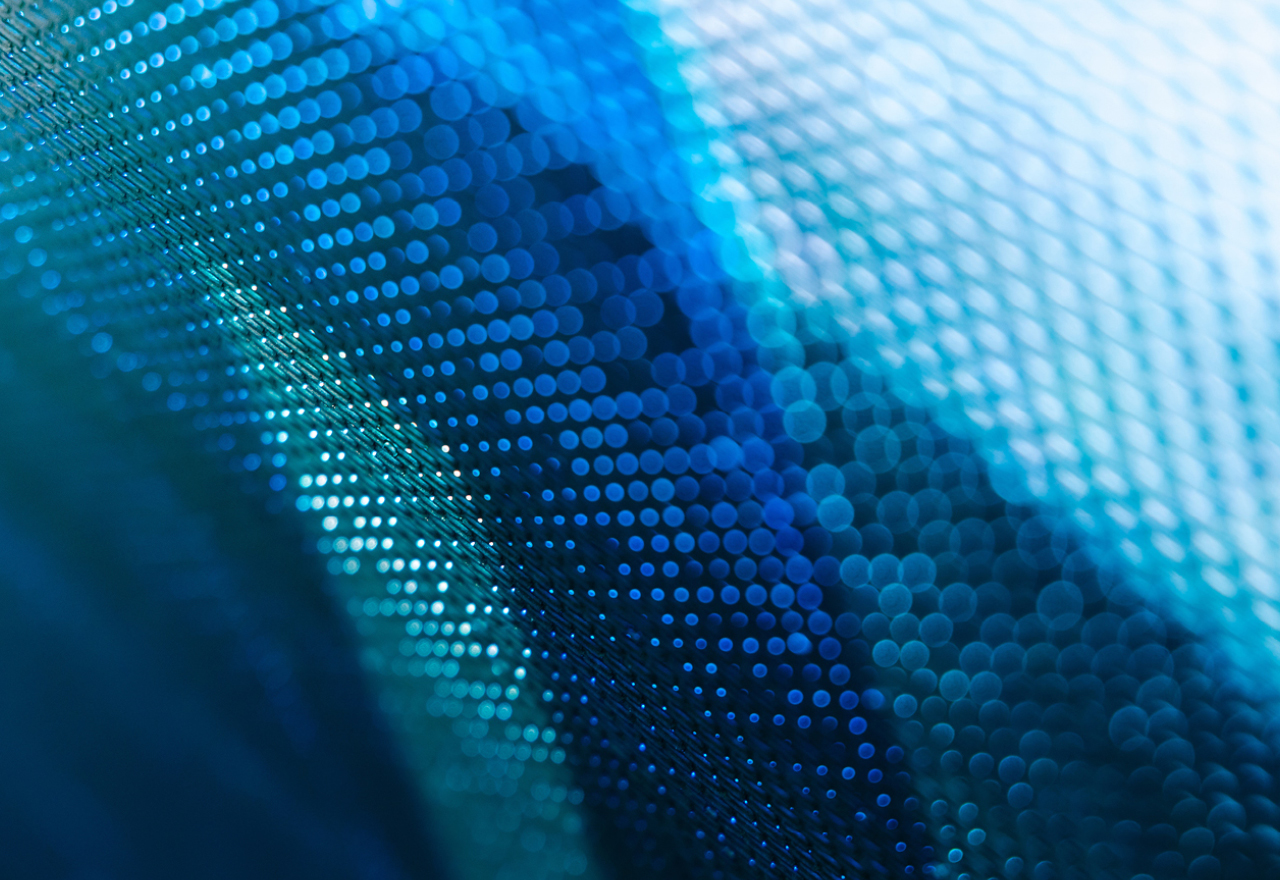
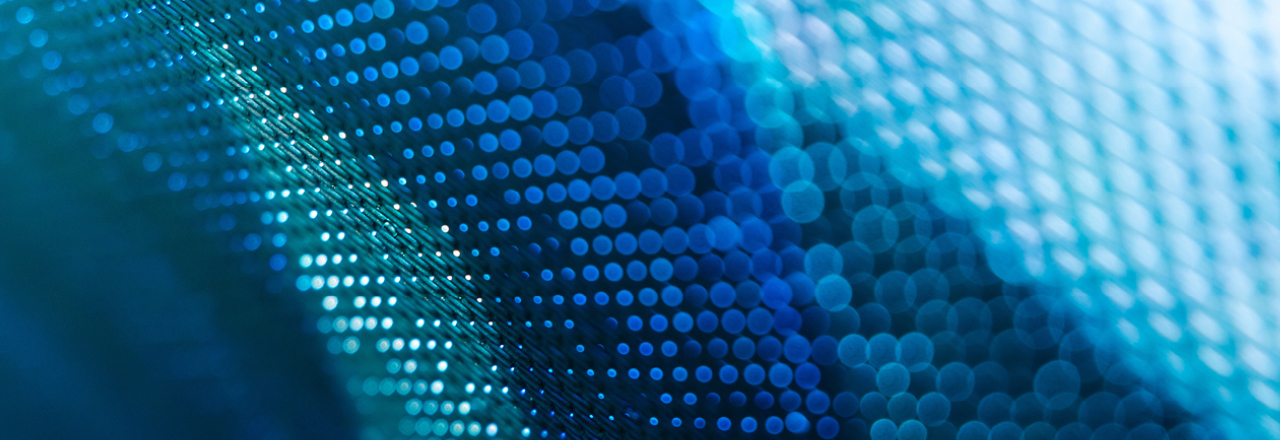
What we've done
Helping ITV better leverage data with a data mesh platform
In response to the paradigm shift of digital streaming, ITV set a new vision: to become a digitally-led media and entertainment company that creates and delivers standout content to audiences wherever, whenever and however they choose. An expert team of Thoughtworkers began co-developing a cloud-based data mesh on AWS and Databricks, a process that would enable ITV to bring its new data strategy to life and embed agile ways of working across its diverse business units.
ITV’s data mesh platform enables teams to quickly onboard their data and make it discoverable and easily accessible across the business. The time taken to provision data products using the platform has gone from three weeks to just a few hours — driving the adoption and expansion of the mesh across ITV’s operations.
Actionable advice
Things to do (Adopt)
Implement privacy-enhancing technologies (PET). These technologies provide increased privacy or secrecy for the persons whose data is processed, stored and/or collected by software and systems. They are often used as a part of this processing and modify the normal ways of handling (and often hoarding) raw or plaintext data directly from users and internal participants, such as employees. By increasing the privacy offered, you are both reducing the owned risk and providing users with better choices about how they'd like their data to be treated.
Enhance data governance and privacy policies. If data is not sufficiently protected and governed internally with clear principles around issues such as privacy and consent, it becomes too risky to take any steps that expose it to the outside world. Before considering wider data sharing and collaboration, enterprises need to clearly define the scope of access and influence over data that various roles have, and embed compliance with policies-as-code into their data platforms.
Streamline data processes and the path to production. Practices like DataOps and MLOps offer techniques to speed up key aspects of the production cycle and improve developer experience, with shorter feedback loops and guardrails that ensure risks are still mitigated.
- Embrace data mesh to deliver insights at scale. Experimenting with data mesh architecture can provide the integration and accessibility needed for various teams to make the most of the data in their domains. This will improve visibility over processes and give teams the ability to rapidly direct development to serve business needs, helping future-proof the enterprise.
Things to consider (Analyze)
Storing data differently. Emerging trends like data clean rooms and differential privacy, which preserves the anonymity of individual aspects of a data set by introducing ‘noise’ around it, can provide a stronger basis for the enterprise to house data in a trustworthy and compliant way while remaining able to put it to use.
Participating in data marketplaces. As more examples of open, pooled data marketplaces emerge, such as the version being advanced by the UK government, businesses should consider their appetite for participating in these initiatives and what the potential of participating in them might be. It’s important to examine questions such as: where might ecosystems enable the enterprise to create more value? And what, if any, capacity is there for the business to monetize data while remaining sensitive to security and customer privacy?
- Utilizing data product specification and data contracts. Open specifications which aim to set out and standardize how data is shared between or consumed by various parties are gaining traction, and they may need to be integrated into the organization’s data platform and wider strategy.
Things to watch for (Anticipate)
The dovetailing of data and responsible technology practice. Responsible tech principles provide an increasingly valuable roadmap for enterprises keen to extend and make optimal use of their data resources in a consistently ethical way.
- Decentralized personal data marketplaces that give consumers more sovereignty over their personal data are contributing to the development of a personal information economy. This trend will have significant implications for the way companies store, analyze and use information about their customers, and the subsequent development of enterprise ecosystems.