Looking Glass 2024
AI everywhere
Leveraging cutting-edge breakthroughs to scale your business
Generative AI (GenAI) has captured the spotlight, but in reality it’s just one aspect of a much broader field that is advancing on multiple fronts. One of the things GenAI has proven is that AI can be made available, accessible and applicable to more people. This democratization has prompted a rush of experimentation and investments in everything from smartphone alternatives to startups working on the next ChatGPT.
What all this will mean for organizations at the day-to-day level is less clear. We are firm believers that AI is already and will continue to have a major impact on some processes integral to being a digital business, notably software development, enabling enterprises to build and bring products to market faster.
It’s important to understand that, for all the excitement, AI won’t always be a transformational force. Heavyweight commercial large language models (LLMs) are powerful but — at least for now — generally too expensive for most organizations to use for anything at scale. The buzz surrounding ChatGPT means that it can become a ‘hammer looking for a nail,’ with companies rushing to integrate it into processes when it may not actually be necessary, or the right tool for the job.
Organizations need to put a few fundamental building blocks in place before they can take advantage of the AI breakthroughs that seem to be emerging every day. One is a solid data strategy, as outlined in our data platform lens, that ensures a base level of relevant, credible and traceable data is readily available to feed into AI models. Without this foundation, an AI solution may simply enable the business to make misguided decisions faster.
It's also critical to employ tools like GenAI with a basic idea of what ‘good’ looks like for the outcome you’re trying to achieve. While these tools can be directed, they can’t be trusted to work without supervision, or to vet the quality of the results. Having a handle on the direction and the output of your AI systems is part of a responsible technology practice, and essential to avoiding unintended consequences.
Once these parameters are in place, we encourage organizations to start testing AI with possible use cases emerging in their operations. Like all innovations, it can be difficult to understand the full potential or range of applications until the technology is firmly in play.
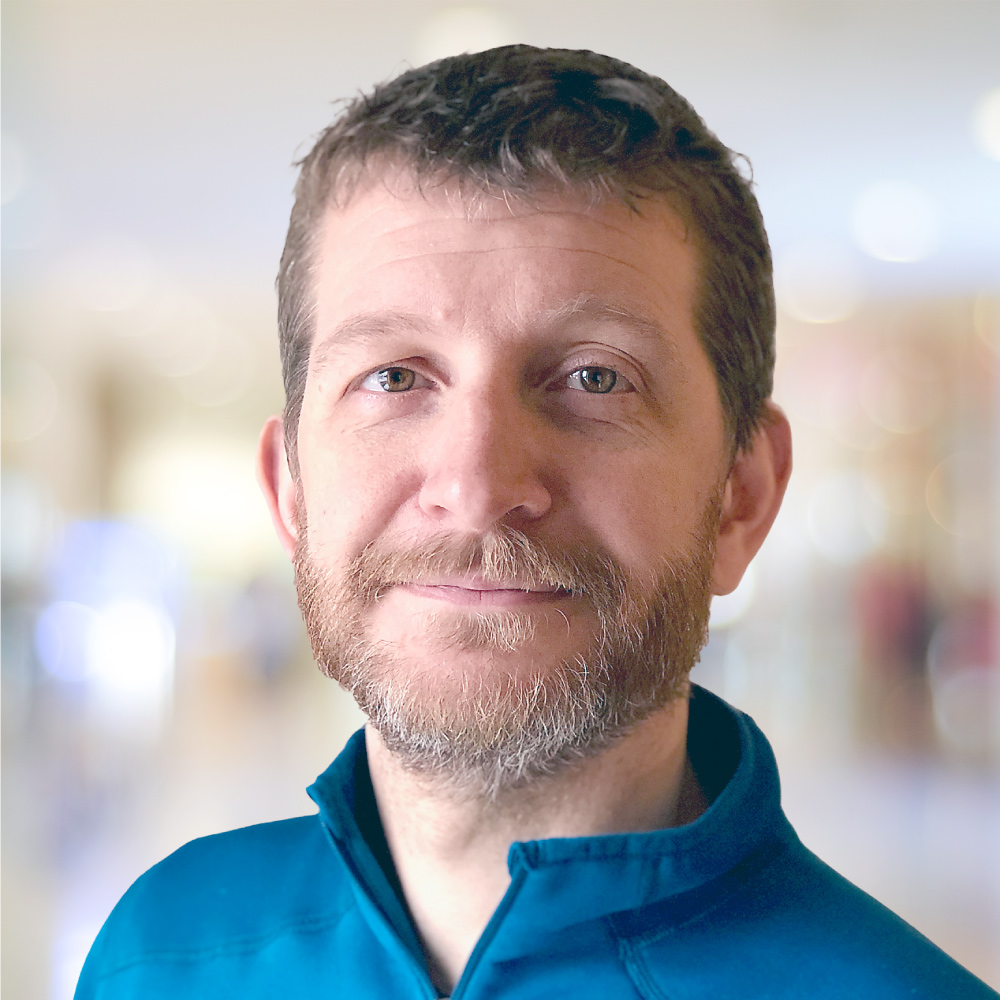
As AI integration becomes more sophisticated and the implications for getting things damagingly wrong multiply, the need for effective risk management grows too.
As AI integration becomes more sophisticated and the implications for getting things damagingly wrong multiply, the need for effective risk management grows too.
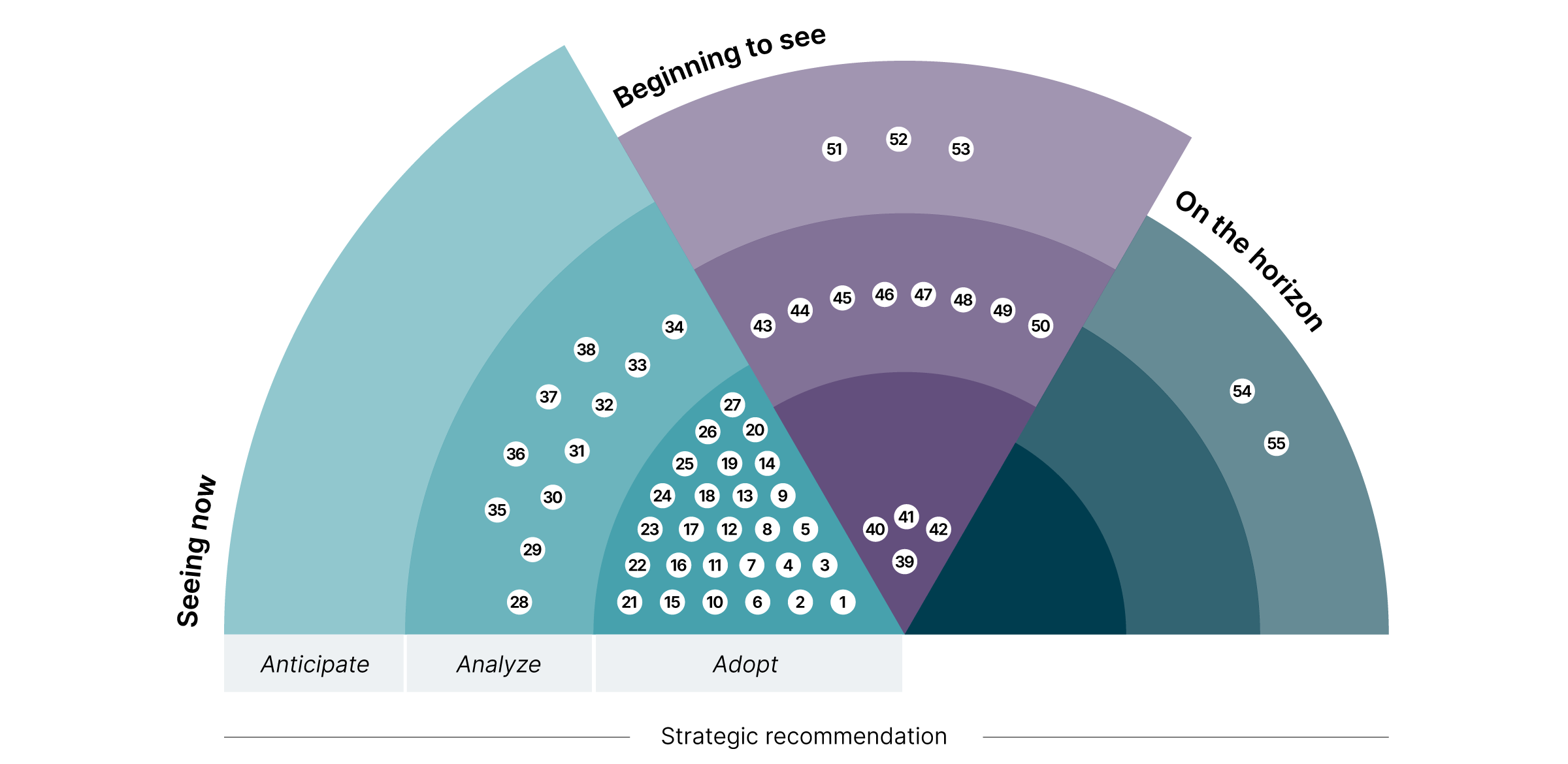
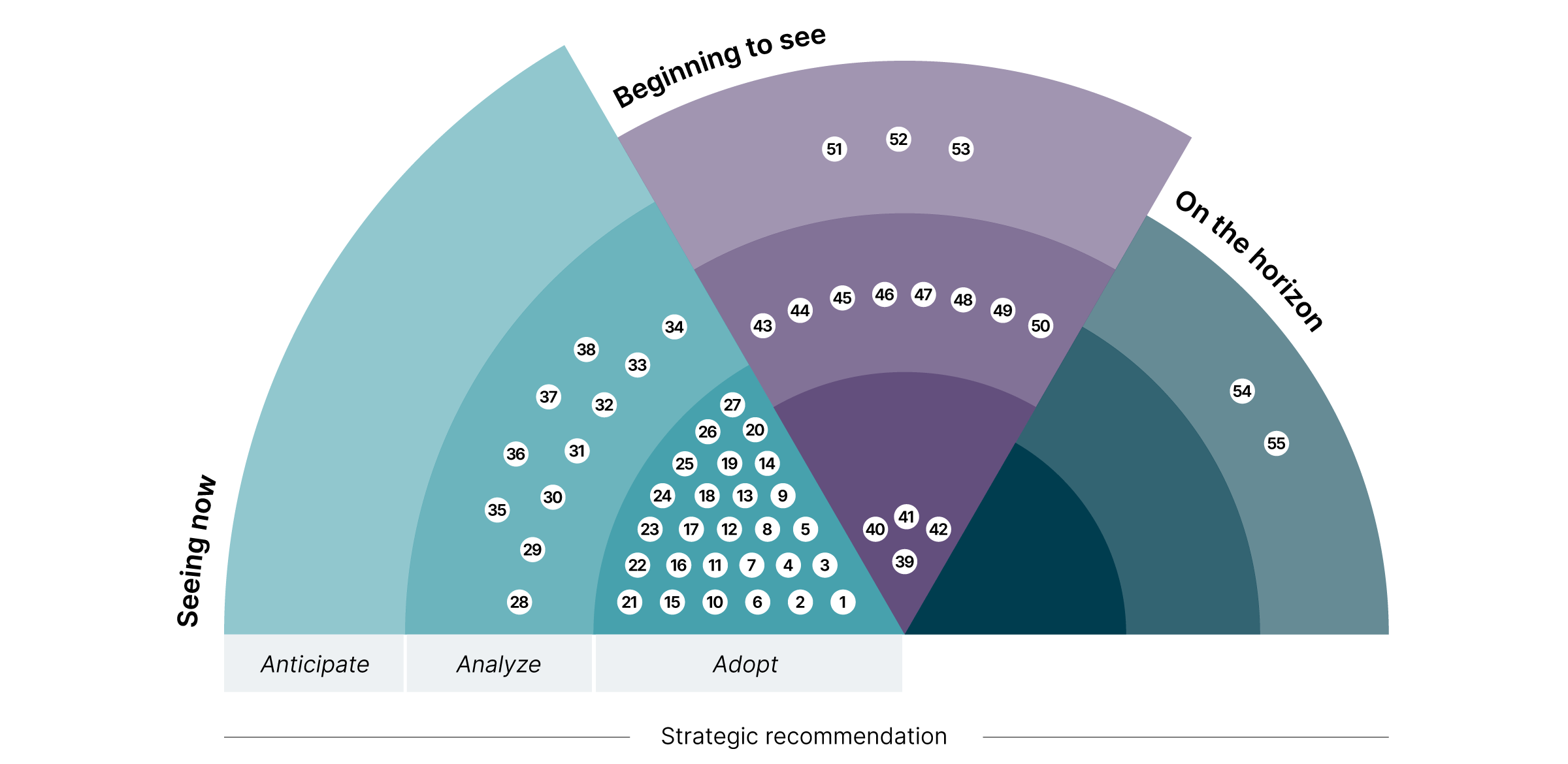
Trends to watch
Adopt
-
Functionality built into applications which combines the functionality of publicly available generative AI models with specific knowledge from outside the model, such as product information.
-
Techniques to draw cause and effect relationships between the input data and the outcomes of a machine learning model, which allows a model to be more generalizable and require less training data to perform effectively.
-
Also known as self-sovereign identity, decentralized identity (DiD) is an open-standards-based identity architecture that uses self-owned and independent digital IDs and verifiable credentials to transmit trusted data. Although not dependent on blockchains, many current examples are deployed on them as well as other forms of distributed ledger technology, and private/public key cryptography, it seeks to protect the privacy of and secure online interactions.
-
More granular access controls for data, such as policy-based (PBAC) or attribute-based (ABAC) that can apply more contextual elements when deciding who has access to data.
Analyze
-
Government regulation and guidance on the use of AI, intended to ensure responsible use and consequences of AI systems. This includes monitoring, compliance and good practice.
-
Combines AI tools and techniques with behavioral and management sciences for the purpose of upskilling and amplifying decision making and decision makers across a variety of complex problems from scenario planning to operations research.
-
AI-powered virtual assistants and non-playable characters that recreate human interaction within the metaverse.
-
Making AI easier to use by lowering the barrier to entry with shared context and other data that those who aren't familiar with prompt engineering may struggle with.
-
Technologies enabling the direct interaction of devices and information sharing between them, usually in an autonomous fashion. This enables to decision making and action with little or no human intervention.
-
AI model interactions that span different modes of communication. For example, a chatbot that understands and responds in both written and spoken language.
-
Systems that monitor metrics across complex distributed systems and take corrective action if a problem is detected. They are often used for security, but increasingly also for resilience and recovery in the face of an outage.
-
An emerging set of techniques to certify the provenance of data and to govern its use across an organization. This could prove transformative in the effort to track and enhance progress towards sustainability targets.
Anticipate
-
These are attacks on (or using) machine learning systems. Attackers may tamper with training data or identify specific inputs that a model classifies poorly to deliberately create undesired outcomes.
-
A collective term for systems and devices that can recognize, interpret, process, simulate and respond to human emotions.
-
Most terms of service (TOS) or end-user license agreements (EULAs) are impenetrable legalese that make it difficult for people without a law background to understand. Understandable consent seeks to reverse this pattern, with easy-to-understand terms and clear descriptions of how customers' data will be used.
Adopt
Analyze
Anticipate
-
An Artificial General Intelligence (AGI) has broad capabilities across a range of intellectual tasks, and is often compared to human-level intelligence. This contrasts with today's "narrow" AI which can be remarkable, but only for very specific tasks.
-
Machine learning algorithms adapted and executed on a quantum computing engine, generally used to analyze classical (non-quantum) data.
The opportunities
By getting ahead of the curve on this lens, organizations can:
Smooth and accelerate human-computer interactions. Advancements in natural language processing (NLP) are opening up new ways for people to communicate with machines, including through everyday conversations. This is both broadening the scope of people who can interact with these systems and making it far easier to plan and execute tasks such as summarizing information, providing self-contained answers to inquiries or context and information to support certain roles or even generating and curating content.
End the terror of the ‘blank page.’ Whatever the task or project, coming up with the initial idea(s) and making a start from essentially nothing is often the toughest part. AI can eliminate blank page paralysis by conducting research and providing a list of suggestions or insights that, even if far from the desired result or finished article, can serve as a jumping-off point or prod recalcitrant minds into action.
Automate tasks — not entire jobs. The need for human involvement to guide and ultimately evaluate AI output makes the wholesale outsourcing of roles to AI systems less likely than many people think. That said, there are a multitude of tasks that AI can automate or where it can augment human input, making the work more consistent and efficient. Any task that requires access to and analysis of a vast body of knowledge — such as a large number of research papers, or databases of medical or financial information — can be seen as a promising candidate for LLM assistance. The bar for what AI can do will constantly shift, but in general, as some have advised, it can be helpful to think of AI ‘not as software, but as pretty good people’ – that is, the equivalent of a competent research assistant or army of well-intentioned interns. There are some duties AI can be trusted with – but it certainly can’t be trusted with everything.
Revolutionize software delivery. There’s a misconception that in building software, GenAI’s main use is as a tool to ease coding, when in reality it can touch on all aspects of the development lifecycle. Potential applications beyond coding assistance include brainstorming with AI to improve our requirements and testing scenarios; improved incident response and debugging by translating natural language into queries over logs and metrics; product and strategy ideation; and searching unstructured institutional information to provide valuable context to developers. Based on our experiences, we believe AI-assisted software delivery has the potential to drive productivity increases of up to 30%.
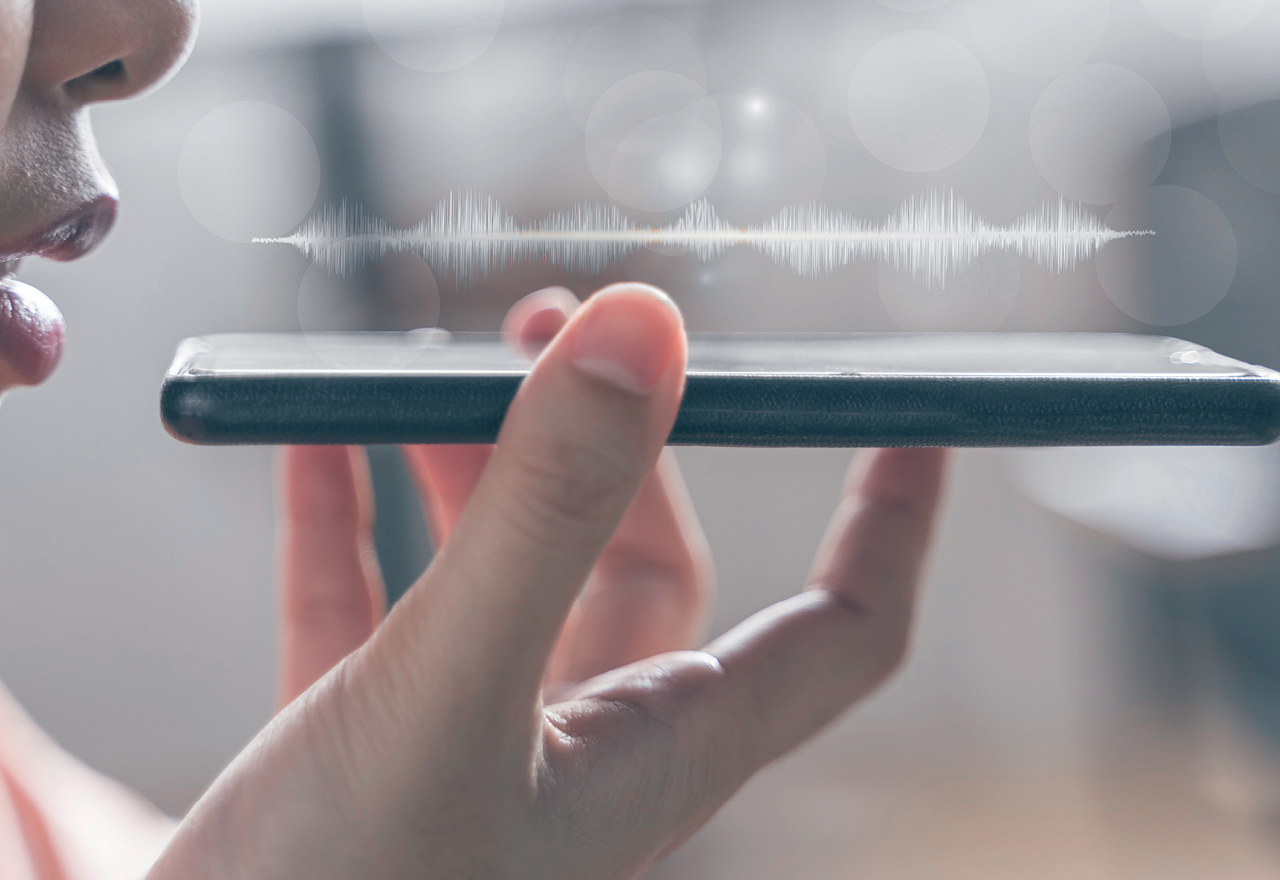
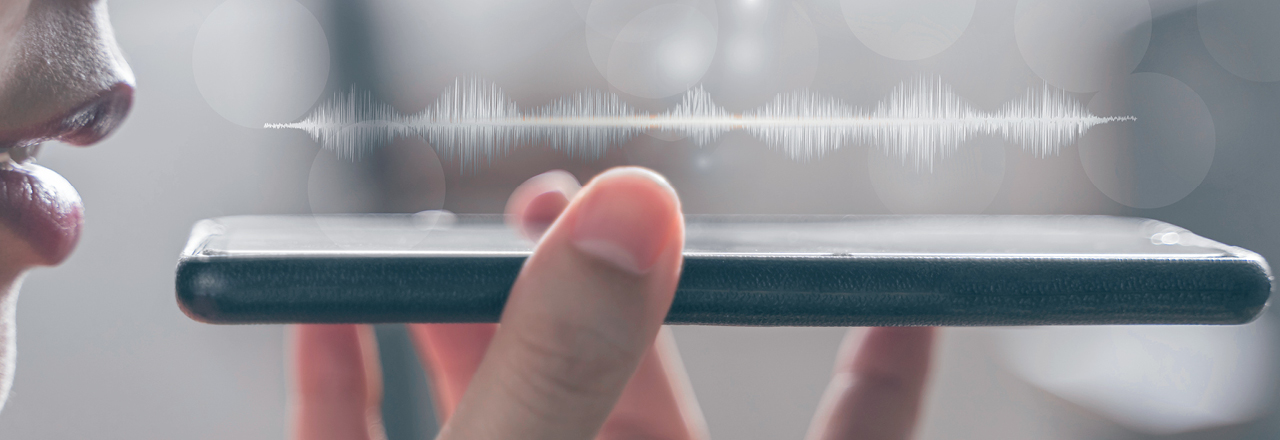
What we've done
Enhancing conversational AI with language models with Jugalbandi
We worked on a chatbot that helps users navigate the complexity of the Indian government’s various welfare schemes. It’s a testament to AI’s ability to not only navigate, process and summarize vast amounts of information in an easily digestible format, but also to meet a much more inclusive user base on its own terms. We combined a number of existing LLMs and translation models to power conversational AI via voice, both incoming and outgoing, in multiple local languages and dialects. This provides an access point and source of information on government services to a rural user base with high illiteracy rates. This has vastly extended and simplified interactions between the Indian government and many of its citizens in remote and non-urban areas.
Actionable advice
Things to do (Adopt)
Identify AI champions who can help guide and teach your organization about the potential use cases for emerging solutions — but understand that AI can and will be applied in different ways in almost every part of the enterprise, which means these champions need to keep an open mind. Having people with a clear idea of what ‘good’ looks like can reduce risks and ensure AI initiatives focus on meaningful business results.
Especially in the short term, focus on how humans and AI work together. Ensure teams understand how AI can augment, not threaten, the tasks that are core to their roles, and where their judgment will need to take over. Watch and manage the costs of services people use, which are generally not visible to an individual user but can add up quickly. Depending on the task, it can be worth sacrificing a degree of accuracy for lower costs, as models that are only slightly less accurate may be substantially cheaper to run.
Identify clear AI use cases that drive real value for your organization, as well as areas where you explicitly will not use AI, either because it makes no business sense, the costs outweigh the necessary investments or the associated risks are simply too high. As the list of potential applications is massive and constantly expanding, having these decisions to orient around will ensure your AI efforts are carefully targeted and therefore more likely to bear fruit.
Be deliberate about which technology you’re using. ‘AI’ has become at times a catchall for a range of distinct technologies and, more recently, used to refer to GenAI alone. The capabilities and use cases for GenAI versus other technologies that are at times lumped under the AI umbrella — like machine learning — can be very different, and clarity is needed on what you’re planning to implement and how it connects to the problems you’re trying to solve.
- Define and communicate ‘guardrails’ early on. Well before they’re interacting with AI on an everyday basis, teams should be aware of standards and expectations in terms of security, data sources and vetting systems or their outputs for transparency and/or bias. They should also be aware of when to give up on experiments when they are unlikely to yield the desired outcome or result in excessive risks.
Things to consider (Analyze)
Open-source alternatives to commercial LLMs, which are improving and more of which are emerging every day. Freely available models like FastChat-T5 can provide a solid base for chatbot and customer support applications, and be developed into specialized models that protect the organization’s intellectual property.
AI Agents. Recent programming interfaces by companies such as OpenAI offer the ability to combine the functionality of publicly available generative AI models with specific knowledge from outside the model, such as product information.
- New vendor offerings. Public cloud vendors such as Amazon and Google Cloud announced a wave of new products and services for people who are creating software towards the end of 2023. In many cases these tools offer compelling features, such as AI-assisted deployment and operation of the software being created. Encourage your AI champions to regularly evaluate a variety of offerings.
Things to watch for (Anticipate)
- Waves of regulation. As demonstrated by the ongoing debate over an AI act in the European Union, governments are scrambling to legislate against some of AI’s more negative perceived impacts and new rules about all facets of AI are likely to be coming fast from all directions. Organizations need to be proactive about establishing policies to ‘do the right thing’ before they are forced to, so compliance becomes a matter of course.